Multi-Classification Of Parkinson'S Disease Via Sparse Low-Rank Learning
2018 24TH INTERNATIONAL CONFERENCE ON PATTERN RECOGNITION (ICPR)(2018)
摘要
Neuroimaging techniques have been widely applied to various neurodegenerative disease analysis to reveal the intricate brain structure. The high dimensional neuroimaging features and limited sample size are the main challenges for the diagnosis task due to the unbalanced input data. To handle it, a sparse low-rank learning framework is proposed, which unveils the underlying relationships between input data and output targets by building a matrix-regularized feature network. Then we obtain the feature weight from the network based on local clustering coefficients. By discarding the irrelevant features and preserving the discriminative structured features, our proposed method can select the most relevant features and identify different stages of Parkinson's disease (PD) from normal controls. Extensive experimental results evaluated on the Parkinson's progression markers initiative (PPMI) dataset demonstrate that the proposed method achieves promising classification performance and outperforms the conventional algorithms. Furthermore, it can detect potential brain regions related to PD for future medical analysis.
更多查看译文
关键词
Parkinson's disease, sparse learning, low-rank learning, feature selection
AI 理解论文
溯源树
样例
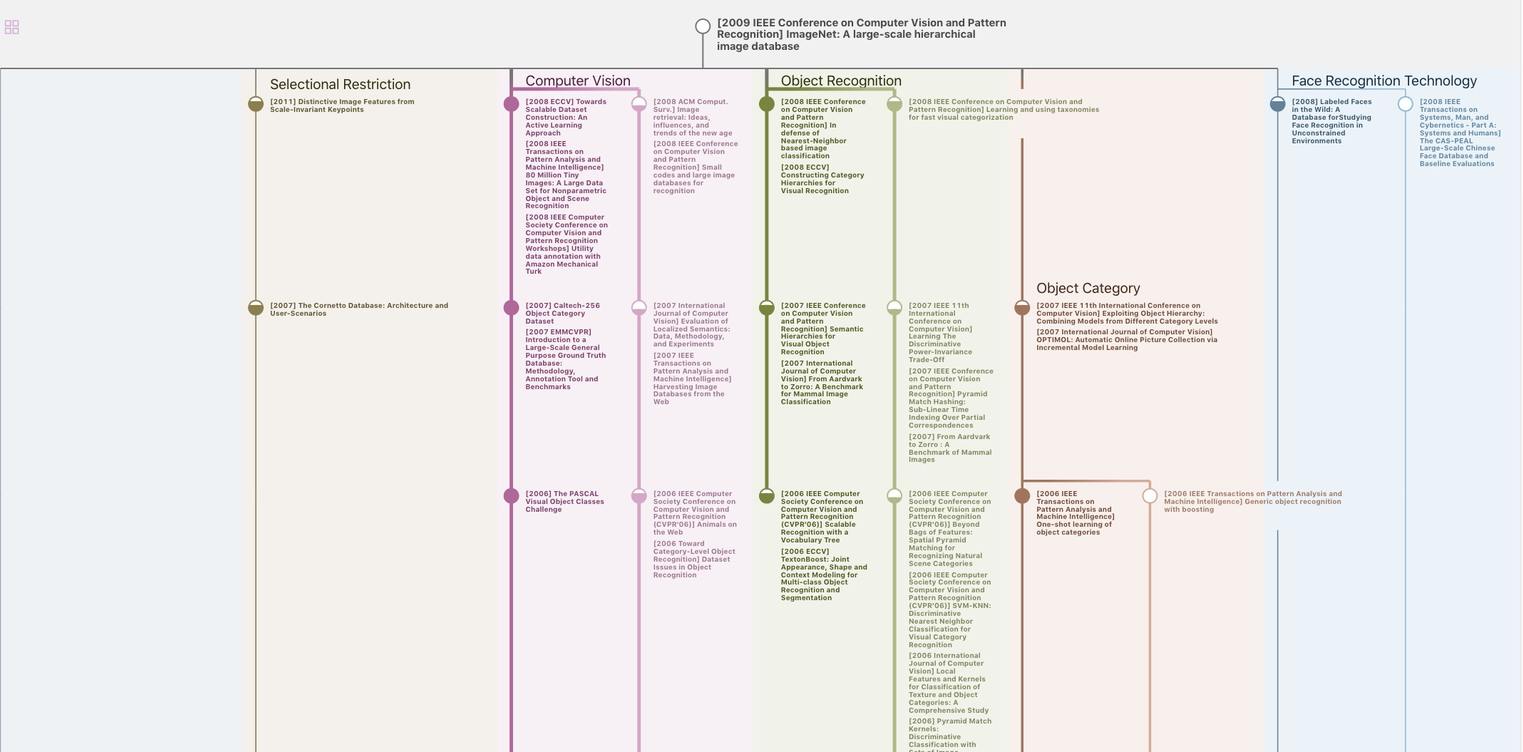
生成溯源树,研究论文发展脉络
Chat Paper
正在生成论文摘要