Robust Visual Tracking via Hierarchical Convolutional Features-Based Sparse Learning
2018 10th International Conference on Wireless Communications and Signal Processing (WCSP)(2018)
摘要
In recent years, convolutional features have significantly advanced the Discriminative Correlation Filter (DCF) based trackers. In contrast to hand-crafted ones, features extracted from a CNN retain high spatial resolution while preserving semantic information. The improvements come at the risk of reduction in speed and over-fitting caused by the insufficiency of training data for tracking. In this paper, a novel Hierarchical Convolutional Features and Sparse learning based Tracker (HCFST) is proposed. We effectively tackle the issues of computational bottlenecks and over-fitting in the DCF formulation via the multi-task sparse learning. First, most of the noisy and irrelevant feature maps are safely removed for robust appearance modeling. Redundant features rejection effectively mitigates the redundancy among features from hierarchical layers of CNNs. Then a sparser updating scheme is further presented for conditional model update. Extensive experiments are performed on various challenging sequences from OTB50 and OTB100 datasets. The proposed HCFST performs favorably against state-of-the-art methods.
更多查看译文
关键词
Feature map selection,hierarchical convolutional features,sparse learning,visual tracking
AI 理解论文
溯源树
样例
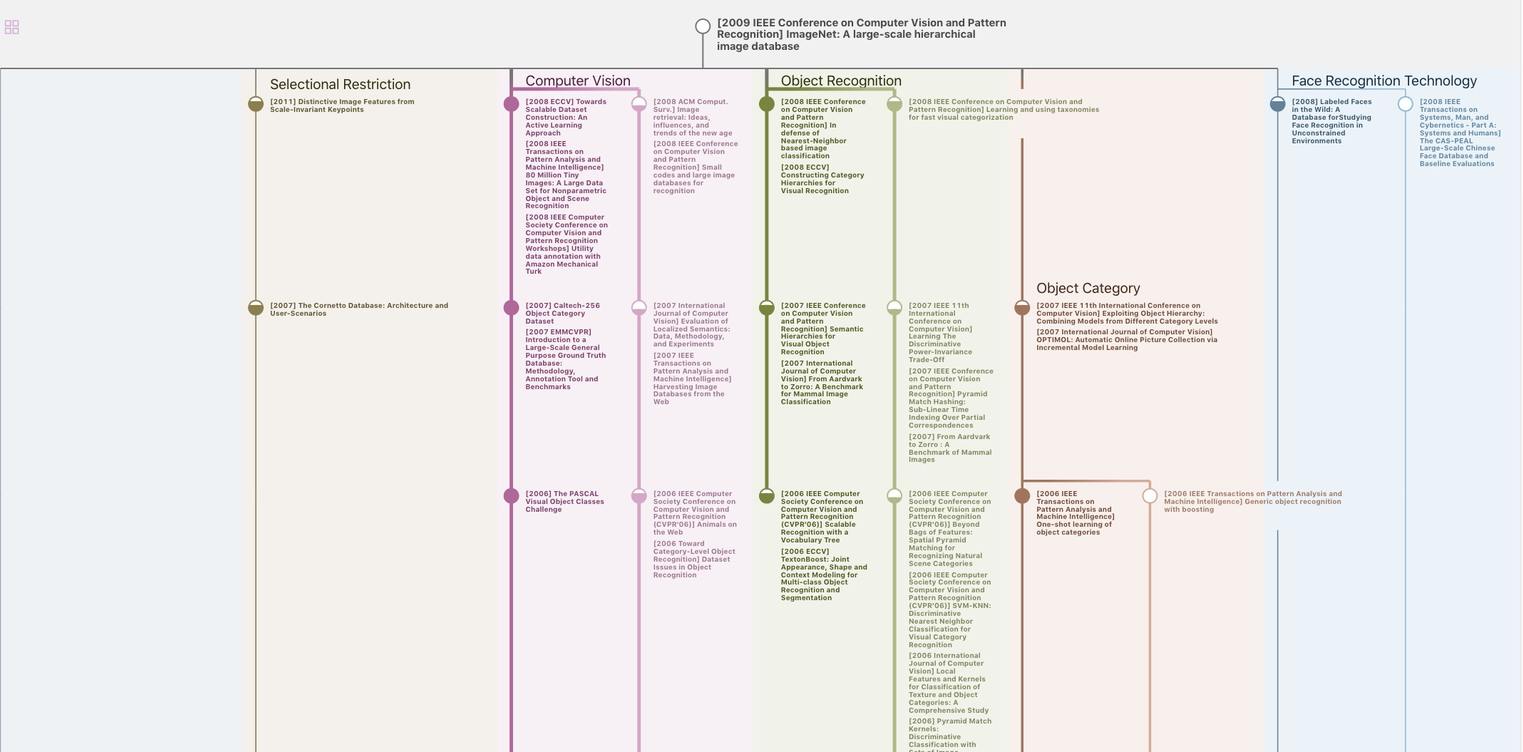
生成溯源树,研究论文发展脉络
Chat Paper
正在生成论文摘要