Pre-ignition Detection Using Deep Neural Networks: A Step Towards Data-driven Automotive Diagnostics
2018 21st International Conference on Intelligent Transportation Systems (ITSC)(2018)
摘要
Fault detection in vehicles is currently carried out using model-based or rule-based approaches. Due to advances in automotive technology such as autonomous driving and further connectivity, the complexity of vehicles and their subsystems increases continuously. As a consequence, models and rule-based systems for fault detection become more complex and require more extensive implementation effort and expert knowledge not only within but also across several domains. Besides, vehicles produce rich amounts of data including valuable information for fault detection. These amounts cannot fully be considered by current fault detection approaches. Deep neural networks offer promising capabilities to address these challenges by allowing automated model generation for fault detection without extensive domain knowledge using vast amounts of in-vehicle data. Hence, in this work a data-driven automotive diagnostics approach to fault detection with deep neural networks is proposed in two steps. First, a novel data-driven diagnostics process to learn data-driven algorithms on in-vehicle data is presented. Second, as a key element of this process, a novel fault detection model is proposed using a combination of convolutional and long short-term memory neural networks. To demonstrate the suitability for fault detection, the model is evaluated on internal engine control unit signals for pre-ignition detection in high-pressure turbocharged petrol engines. A classical machine learning processing pipeline and each neural network type separately are used as baselines for a performance comparison. Results show that the proposed model is a promising approach to data-driven automotive diagnostics outperforming all implemented baselines.
更多查看译文
关键词
deep neural networks,in-vehicle data,data-driven automotive diagnostics approach,data-driven algorithms,pre-ignition detection,fault detection approaches,data-driven diagnostics process,automotive technology,convolutional neural networks,long short-term memory neural networks,internal engine control unit signals,high-pressure turbocharged petrol engines,machine learning processing pipeline
AI 理解论文
溯源树
样例
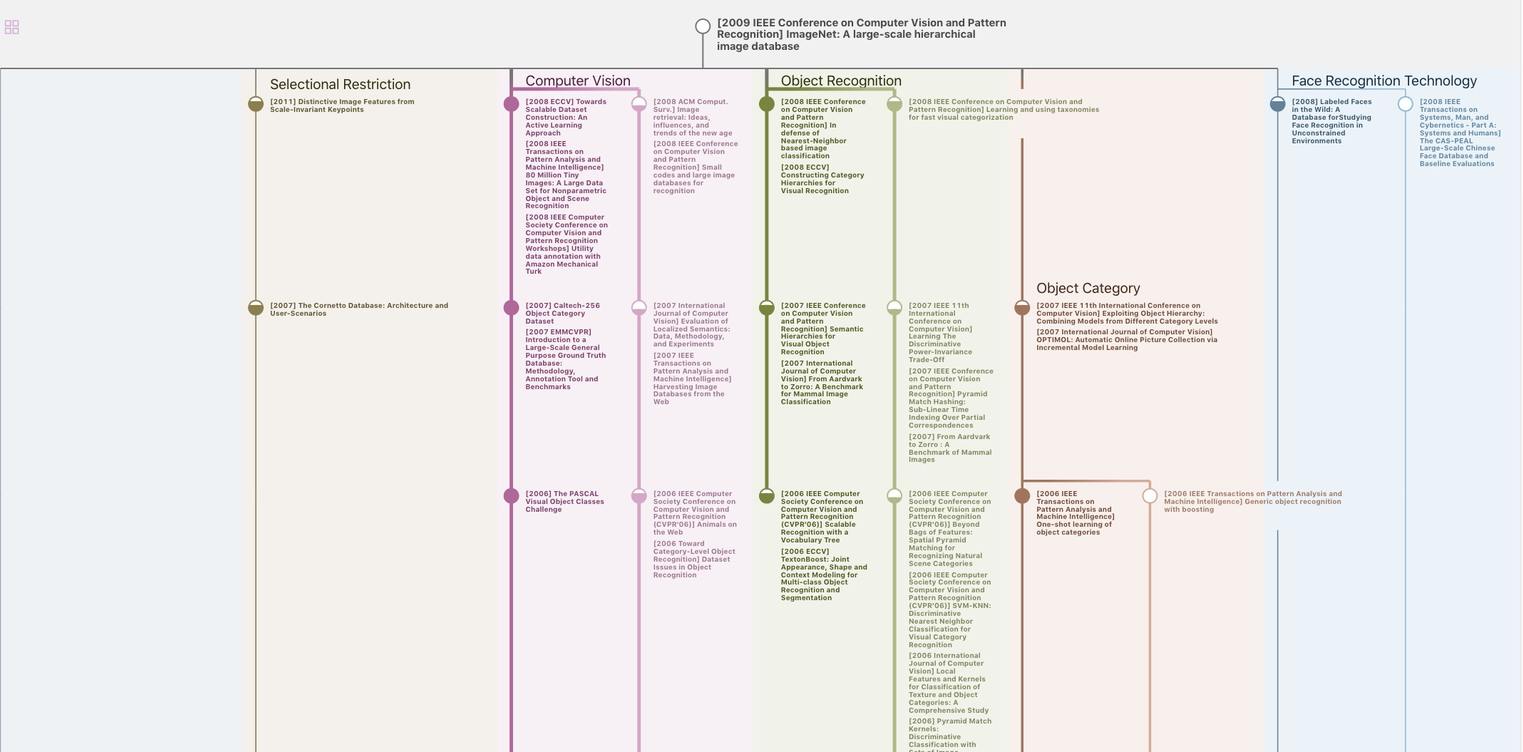
生成溯源树,研究论文发展脉络
Chat Paper
正在生成论文摘要