Environment Estimation with Dynamic Grid Maps and Self-Localizing Tracklets.
ITSC(2018)
摘要
Dynamic environment representation is an important and demanding topic in the field of autonomous driving. One of the generic ways to estimate the surrounding world of an intelligent vehicle is to use dynamic grid maps. However, there are still several unsolved challenges in the grid-based tracking solutions like the ability to converge faster and providing a more efficient way to fuse multi-sensorial information. In this work, we address both of these challenges as a single probabilistic estimator. First, we treat the grid map estimation process as a multi-channel tracking mechanism. In particular, we use a particle filter based solution to integrate both the occupancy and semantic grids. Second, we adapt the idea of simultaneous grid cell tracking and object shape estimation into the grid map domain and propose “self-localizing tracklets”, which are individual particle filter based estimators that are used for two main tasks: stabilizing the position estimation accuracy of dynamic cells with respect to the object boundary, and estimating a better object shape. The presented concepts offer an improved representation flexibility and a faster algorithm convergence.
更多查看译文
关键词
environment estimation,self-localizing tracklets,dynamic environment representation,multisensorial information,single probabilistic estimator,multichannel tracking mechanism,semantic grids,position estimation accuracy,dynamic grid map estimation process,grid cell tracking,autonomous driving,intelligent vehicle,grid-based tracking solutions,object shape estimation,particle filter based estimator solution
AI 理解论文
溯源树
样例
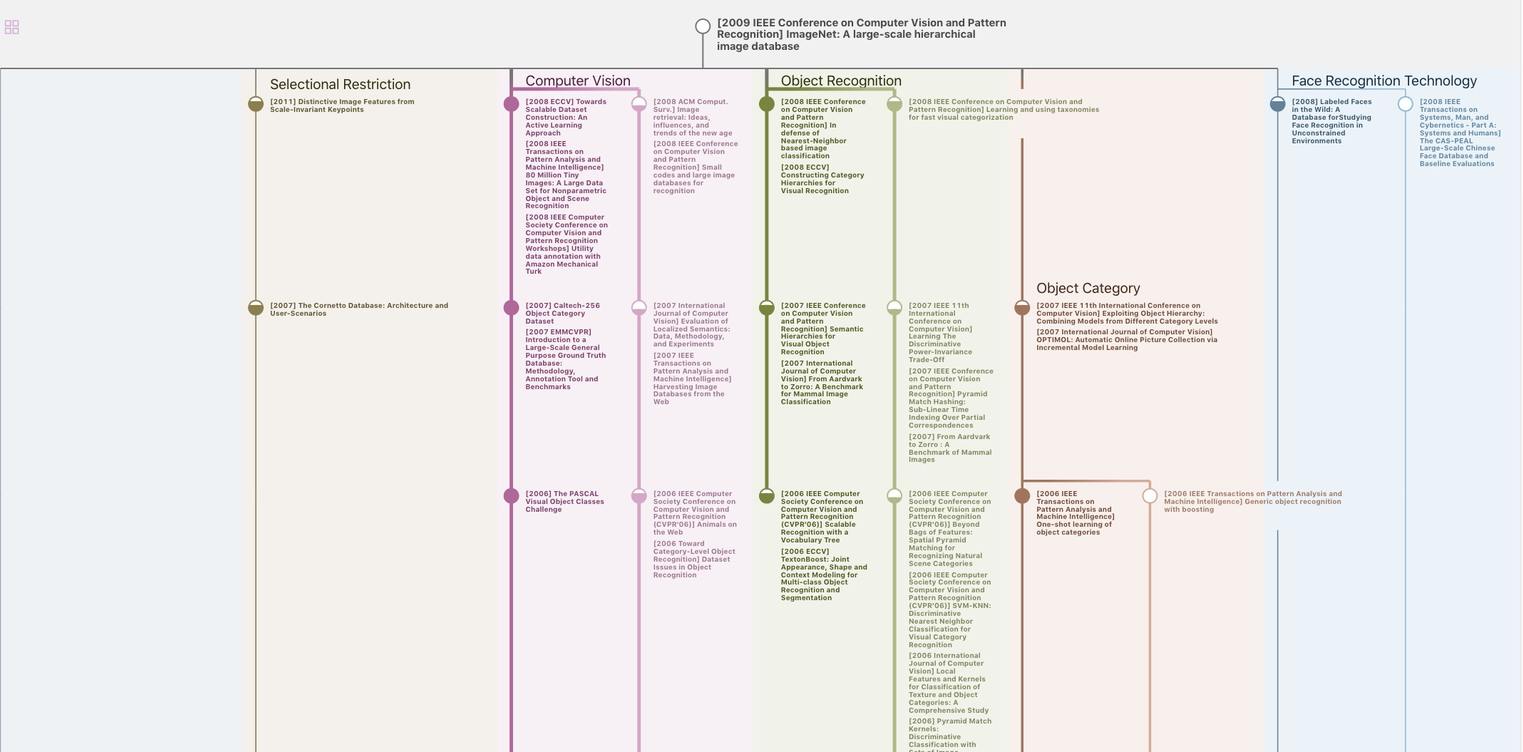
生成溯源树,研究论文发展脉络
Chat Paper
正在生成论文摘要