Learning To Attend To Salient Targets In Driving Videos Using Fully Convolutional Rnn
2018 21ST INTERNATIONAL CONFERENCE ON INTELLIGENT TRANSPORTATION SYSTEMS (ITSC)(2018)
摘要
Driving involves the processing of rich audio, visual and haptic signals to make safe and calculated decisions on the road. Human vision plays a crucial role in this task and analysis of the gaze behavior could provide some insights into the action the driver takes upon seeing an object/region. A typical representation of the gaze behavior is a saliency map. The work in this paper aims to predict this saliency map given a sequence of image frames. Strategies are developed to address important topics for video saliency including active gaze (i.e. gaze that is useful for driving), pixel- and object level information, and suppression of non-negative pixels in the saliency maps. These strategies enabled the development of a novel pixel- and object-level saliency ground truth dataset using real-world driving data around traffic intersections. We further proposed a fully convolutional RNN architecture capable of handling time sequence image data to estimate saliency map. Our methodology shows promising results.
更多查看译文
关键词
human vision,gaze behavior,saliency map,video saliency,active gaze,object-level information,nonnegative pixels,real-world driving data,time sequence image data,salient targets,driving videos,object-level saliency ground truth dataset,fully convolutional RNN architecture
AI 理解论文
溯源树
样例
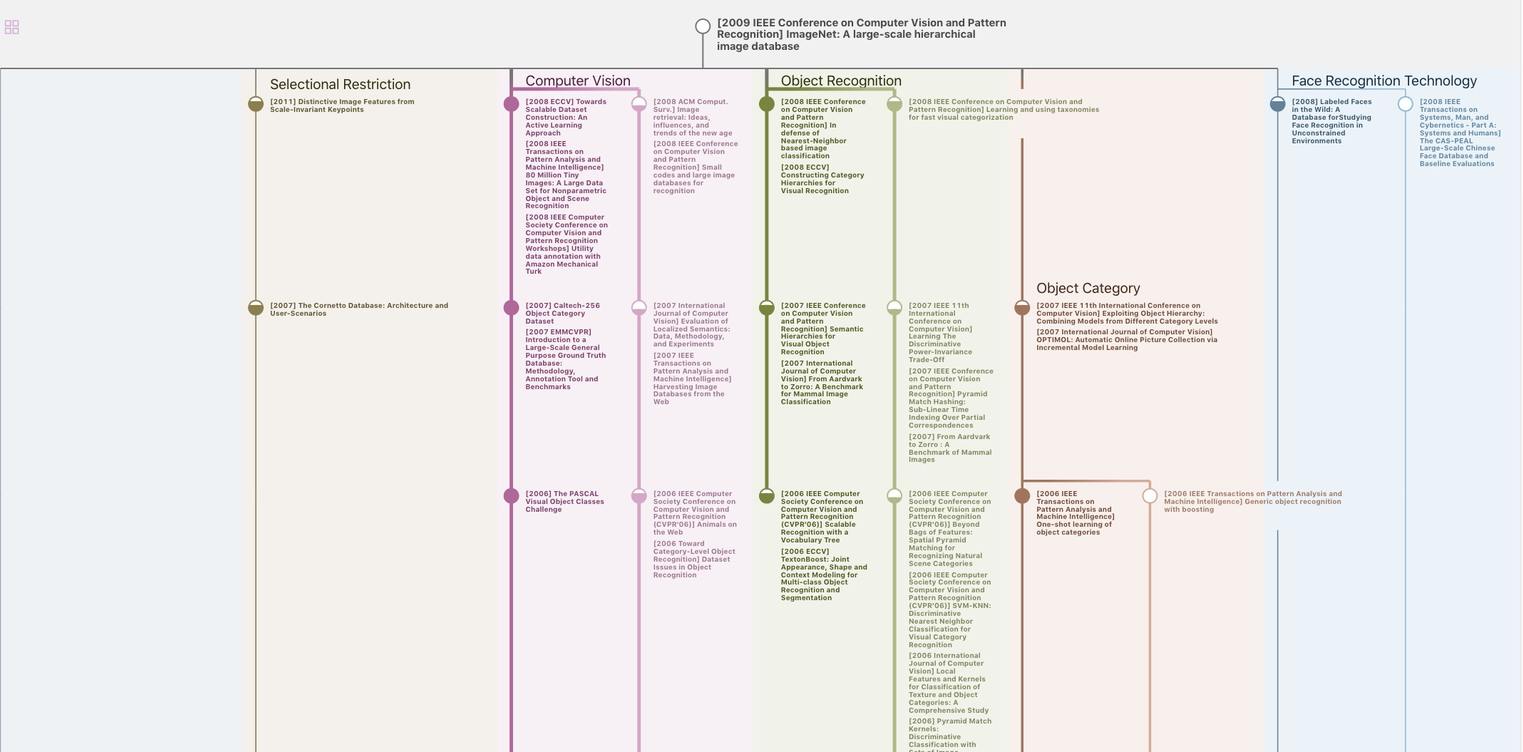
生成溯源树,研究论文发展脉络
Chat Paper
正在生成论文摘要