Lane Change Detection Using V2v Safety Messages
2018 21ST INTERNATIONAL CONFERENCE ON INTELLIGENT TRANSPORTATION SYSTEMS (ITSC)(2018)
摘要
This paper compares three supervised learning techniques (support vector machines, random forest, and decision trees with gradient boosting) applied to the problem of detecting lane change maneuvers of a remote vehicle using Vehicle-to-Vehicle (V2V) safety messages based on automotive standards. The feature vector used for training is derived from the transmitted measurements smoothed over a sliding window, and it includes some differential measurements. Classifier training and evaluation via cross validation is performed on a real vehicle data set consisting of 740 km of drive data capturing over 1000 lane change maneuvers on California highways. The results show that the supervised learning techniques successfully predict 98.4% of remote vehicle lane changes with a mean detection time of 0.31 sec on straight roadways and 89.5% of lane changes with a mean detection time of 0.62 sec on curved roadways. The detection algorithm has also shown to be robust against packet loss for a wide range of Packet Error Rate (PER).
更多查看译文
关键词
Automated Driving, ADAS, Lane Change Detection, V2V Communications, DSRC, Machine Learning
AI 理解论文
溯源树
样例
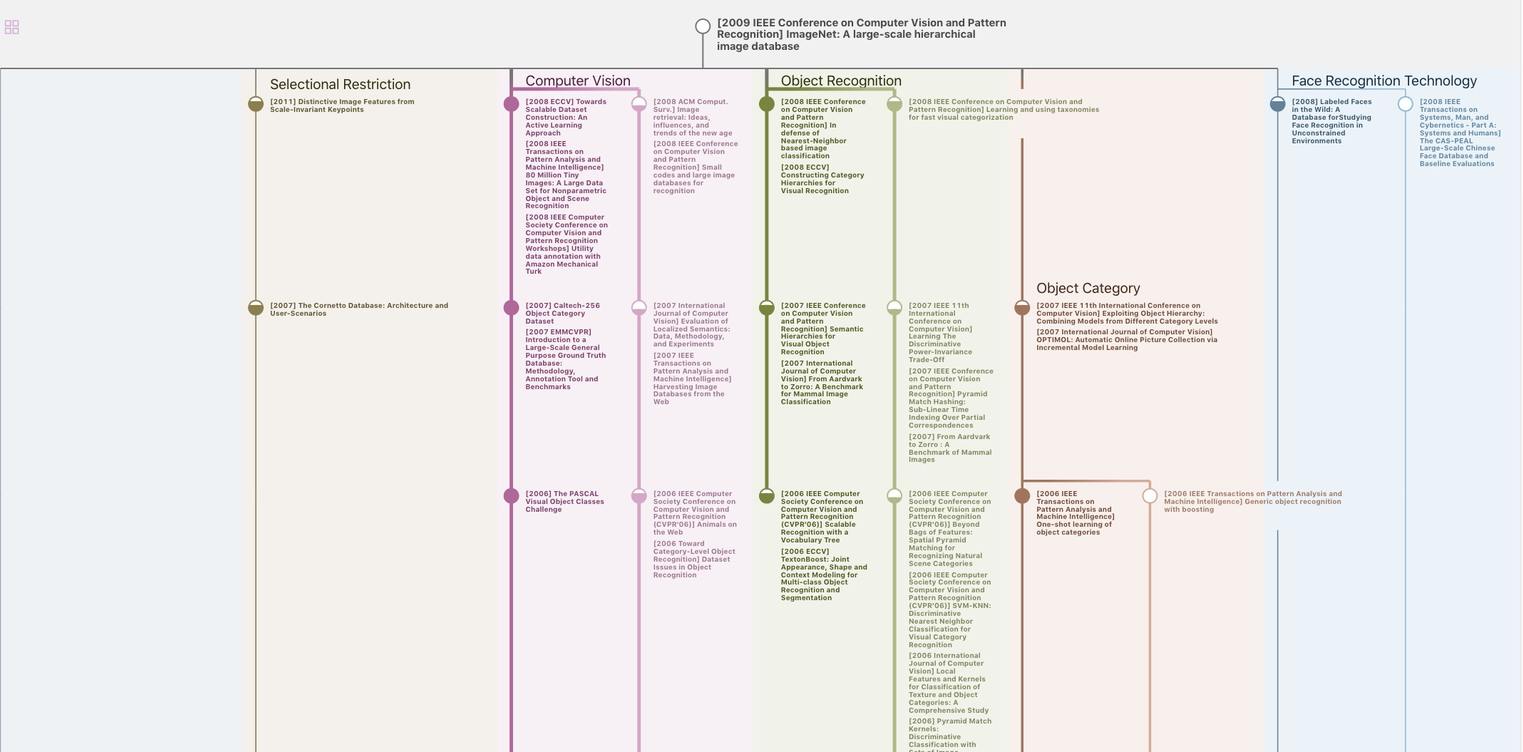
生成溯源树,研究论文发展脉络
Chat Paper
正在生成论文摘要