DDT: Deep Driving Tree for Proactive Planning in Interactive Scenarios
2018 21st International Conference on Intelligent Transportation Systems (ITSC)(2018)
摘要
We consider long-term planning problems for autonomous vehicles in complex traffic scenarios where vehicles and pedestrians interact. The decisions of an autonomous vehicle can influence surrounding other participants in these scenarios. Therefore, planning algorithms that preprocess the long-term prediction of other participants restrict freedom in action. In this paper, we process both problems of long-term planning and prediction at the same time. Our approach which we call DDT (Deep Driving Tree) is based on game tree accumulating a short-term prediction. Machine learning techniques are applied to this short-term prediction instead of model-based techniques that depends on domain knowledge. In contrast to Q-learning, this prediction part is trained off-line and does not require feedback from collision data. Our approach using a game tree models multiple future states of other participants to decide a proactive action taking uncertainties of their intentions into consideration. This approach is demonstrated in a left turning scenario at an intersection of left-hand traffic with oncoming vehicles without V2V communication.
更多查看译文
关键词
Autonomous driving,intent estimation,machine learning,decision making
AI 理解论文
溯源树
样例
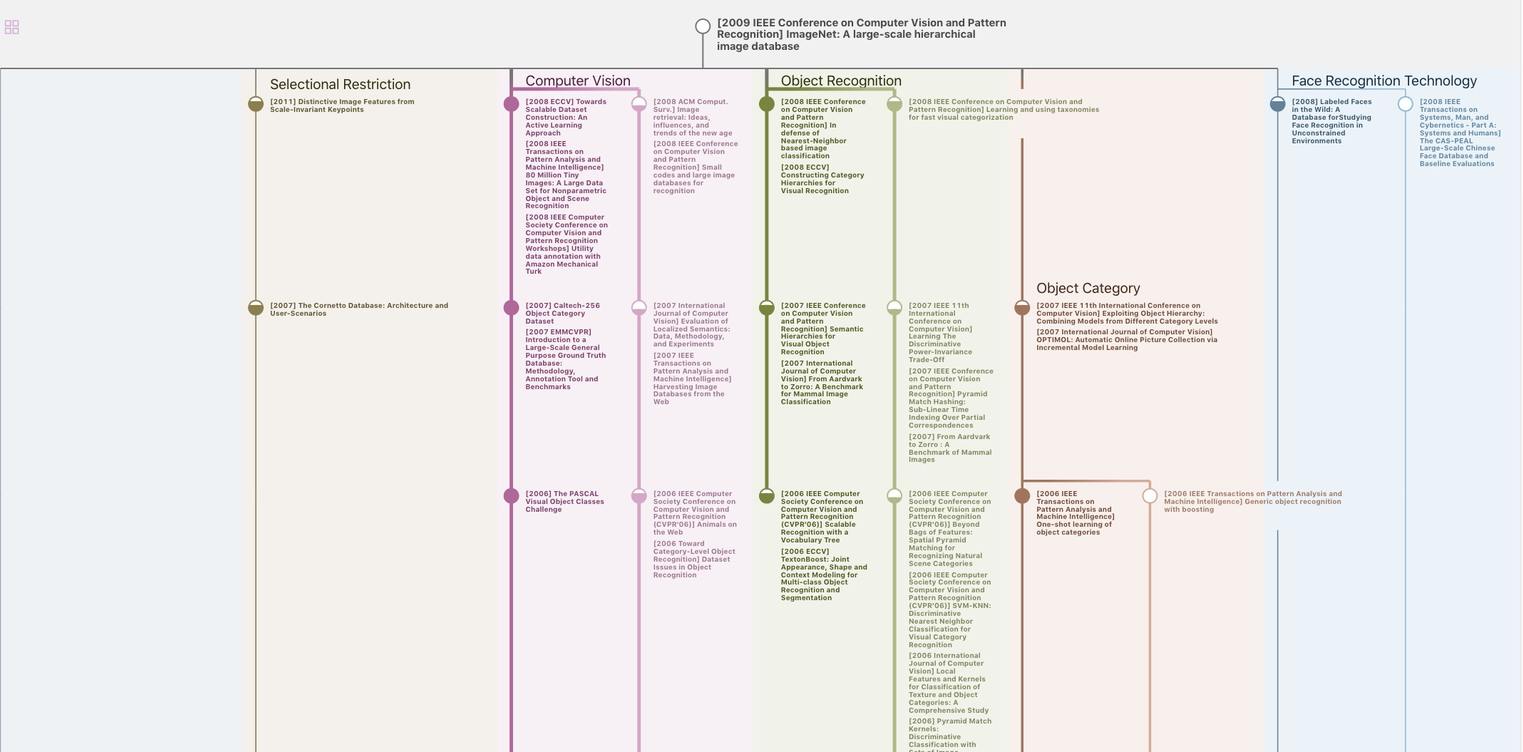
生成溯源树,研究论文发展脉络
Chat Paper
正在生成论文摘要