Metagan: An Adversarial Approach To Few-Shot Learning
ADVANCES IN NEURAL INFORMATION PROCESSING SYSTEMS 31 (NIPS 2018)(2018)
摘要
In this paper, we propose a conceptually simple and general framework called MetaGAN for few-shot learning problems. Most state-of-the-art few-shot classification models can be integrated with MetaGAN in a principled and straightforward way. By introducing an adversarial generator conditioned on tasks, we augment vanilla few-shot classification models with the ability to discriminate between real and fake data. We argue that this GAN-based approach can help few-shot classifiers to learn sharper decision boundary, which could generalize better. We show that with our MetaGAN framework, we can extend supervised few-shot learning models to naturally cope with unlabeled data. Different from previous work in semi-supervised few-shot learning, our algorithms can deal with semi-supervision at both sample-level and task-level. We give theoretical justifications of the strength of MetaGAN, and validate the effectiveness of MetaGAN on challenging few-shot image classification benchmarks.
更多查看译文
关键词
decision boundary,image classification,learning problems
AI 理解论文
溯源树
样例
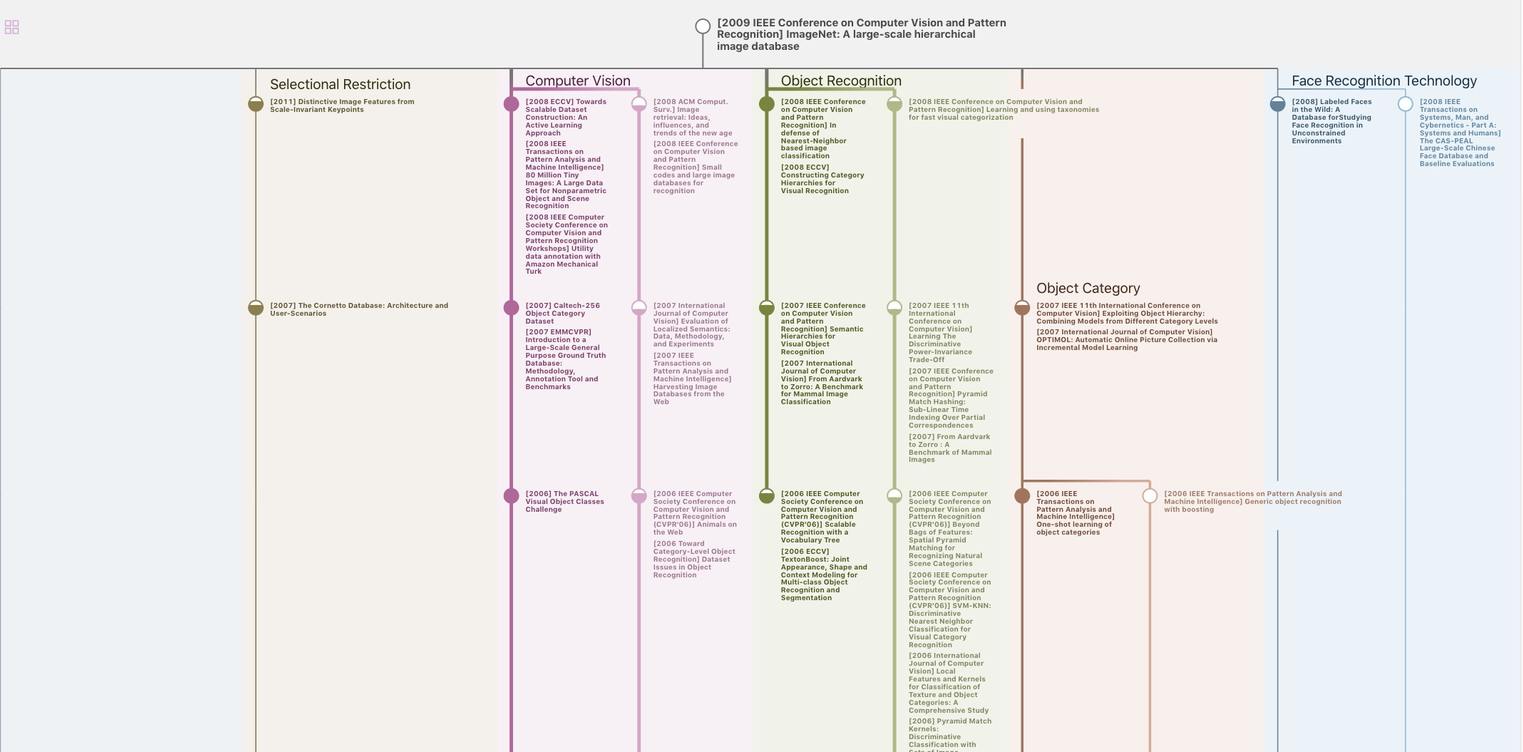
生成溯源树,研究论文发展脉络
Chat Paper
正在生成论文摘要