Vehicle Behavior Prediction and Generalization Using Imbalanced Learning Techniques.
ITSC(2021)
摘要
The use of learning-based methods for vehicle behavior prediction is a promising research topic. However, many publicly available data sets suffer from class distribution skews which limits learning performance if not addressed. This paper proposes an interaction-aware prediction model consisting of an LSTM autoencoder and SVM classifier. Additionally, an imbalanced learning technique, the multiclass balancing ensemble is proposed. Evaluations show that the method enhances model performance, resulting in improved classification accuracy. Good generalization properties of learned models are important and therefore a generalization study is done where models are evaluated on unseen traffic data with dissimilar traffic behavior stemming from different road configurations. This is realized by using two distinct highway traffic recordings, the publicly available NGSIM US-101 and I80 data sets. Moreover, methods for encoding structural and static features into the learning process for improved generalization are evaluated. The resulting methods show substantial improvements in classification as well as generalization performance.
更多查看译文
关键词
prediction,vehicle,generalization
AI 理解论文
溯源树
样例
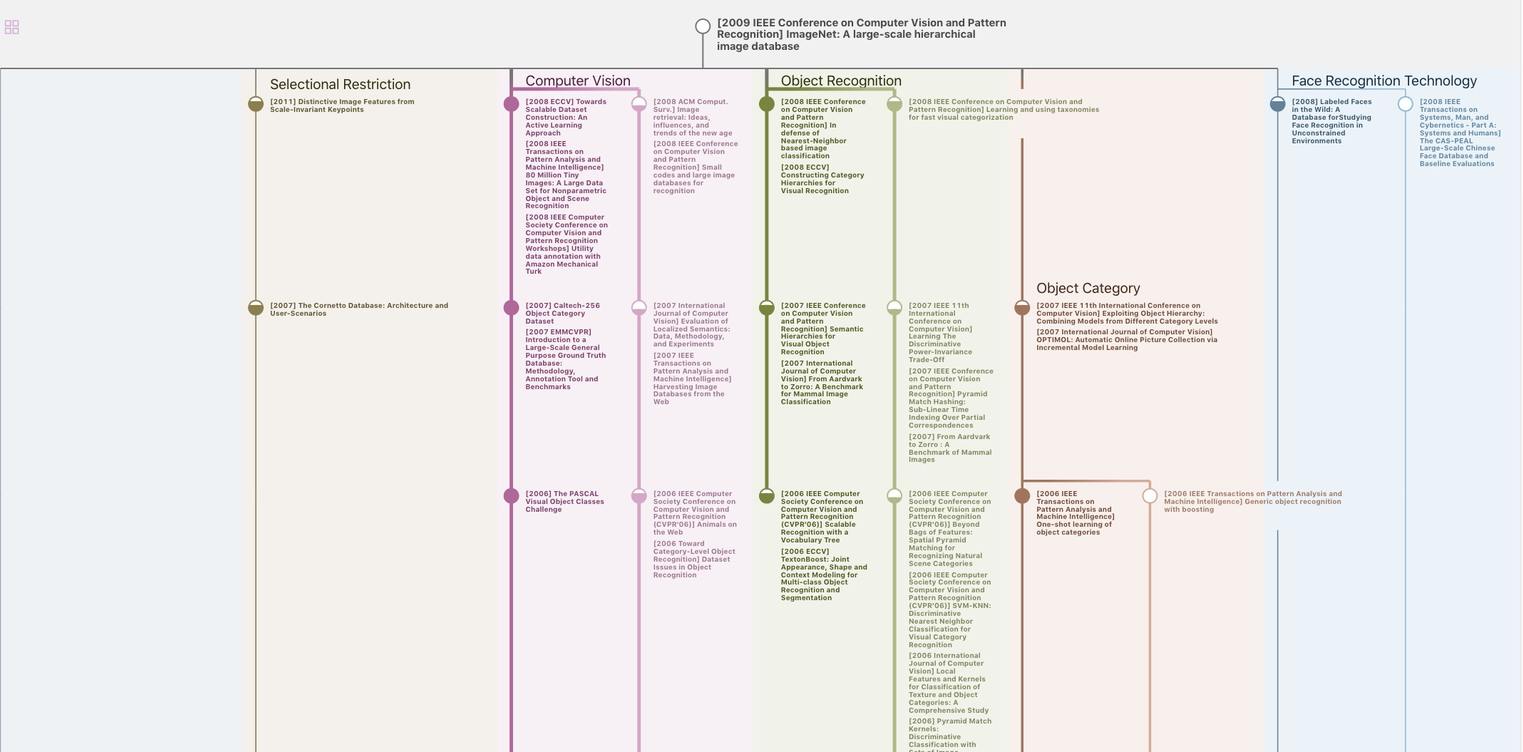
生成溯源树,研究论文发展脉络
Chat Paper
正在生成论文摘要