A Regularized Nonnegative Third Order Tensor decomposition Using a Primal-Dual Projected Gradient Algorithm - Application to 3D Fluorescence Spectroscopy.
ICSM(2018)
摘要
This paper investigates the use of Primal-Dual optimization algorithms on multidimensional signal processing problems. The data blocks interpreted in a tensor way can be modeled by means of multi-linear decomposition. Here we will focus on the Canonical Polyadic Decomposition (CPD), and we will present an application to fluorescence spectroscopy using this decomposition. In order to estimate the factors or latent variables involved in these decompositions, it is usual to use criteria optimization algorithms. A classical cost function consists of a measure of the modeling error (fidelity term) to which a regularization term can be added if necessary. Here, we consider one of the most efficient optimization methods, Primal-Dual Projected Gradient.
更多查看译文
关键词
Constrained optimization, Nonnegative tensor decomposition, Primal-Dual, Regularization, Projected gradient
AI 理解论文
溯源树
样例
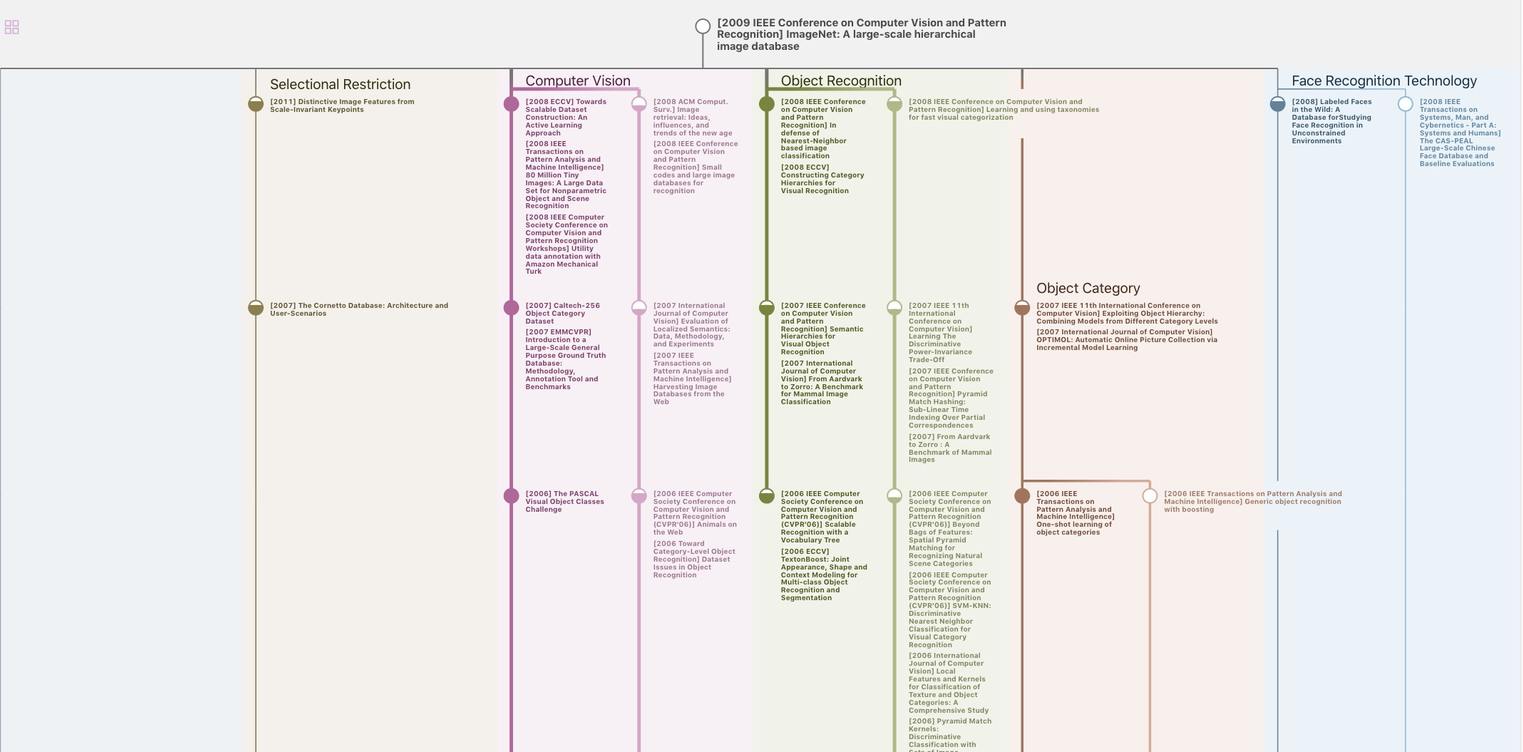
生成溯源树,研究论文发展脉络
Chat Paper
正在生成论文摘要