An Entailment-Based Scoring Method For Content Selection In Document Summarization
PROCEEDINGS OF THE NINTH INTERNATIONAL SYMPOSIUM ON INFORMATION AND COMMUNICATION TECHNOLOGY (SOICT 2018)(2018)
摘要
This paper introduces a scoring method to improve the quality of content selection in an extractive summarization system. Different from previous models mainly using local information inside sentences such as sentence position or sentence length, our method judges the importance of a sentence based on its own information and the relation between sentences. For the relation between sentences, we utilize textual entailment, a relationship indicating that the meaning of a sentence can be inferred from another one. Unlike previous work on using textual entailment for summarization, we go a step further by looking at aligned words in an entailment sentence pair. Assuming that important words in a salient sentence can be aligned by several words in other sentences, word alignment scores are exploited to compute the entailment score of a sentence. To take advantage of local and neighbor information for facilitating the salient estimation of sentences, we combine entailment scores with sentence position scores. We validate the proposed scoring method with greedy or integer linear programming approaches for extracting summaries. Experiments on three datasets (including DUC 2001 and 2002) in two different domains show that our model obtains competitive ROUGE-scores with state-of-the-art methods for single-document summarization.
更多查看译文
关键词
Web Document Summarization, Entailment, Sentence Scoring, Integer Linear Programming (ILP)
AI 理解论文
溯源树
样例
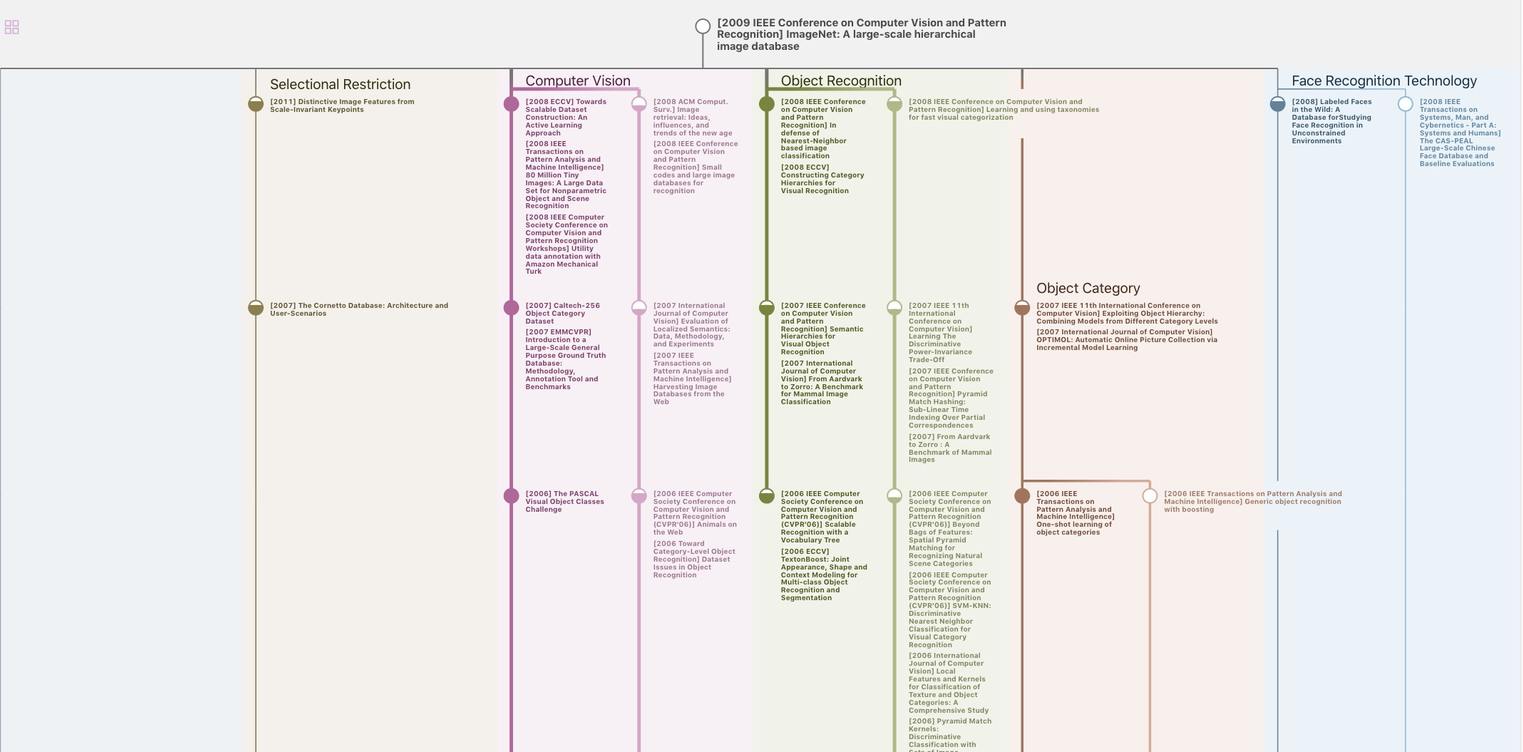
生成溯源树,研究论文发展脉络
Chat Paper
正在生成论文摘要