Mobile Multi-Scale Vehicle Detector And Its Application In Traffic Surveillance
PROCEEDINGS OF THE NINTH INTERNATIONAL SYMPOSIUM ON INFORMATION AND COMMUNICATION TECHNOLOGY (SOICT 2018)(2018)
摘要
Object detection is a major problem in computer vision. Recently, deep neural architectures have shown a dramatic boost in performance, but they are often too slow and burdensome for embedded and real-time applications such as video surveillance. In this paper, we describe a new object detection architecture that is faster than state-of-the-art detectors while improving the performance of small mobile models. Moreover, we apply this new architecture into the problem of vehicle detection, which is central to traffic surveillance systems. In more detail, our architecture uses an efficient backbone network in MobileNetV2, whose building blocks consist of depth wise convolutional layers. On top of this network, we build a feature pyramid using separable layers so that the model can detect objects at many scales. We train this network with smooth localization loss and weighted softmax loss in tandem with hard negative mining. Both training and test sets are built from recorded videos of Ho Chi Minh and Da Nang traffic or selected from DETRAC dataset. The experimental results show that our proposed solution can still achieve an mAP of 75% on the test set while using only around 3.4 million parameters and running at 100ms per image on a cheap machine.
更多查看译文
关键词
object detection, deep neural network, real-time, feature pyramid, vehicle dataset, traffic surveillance
AI 理解论文
溯源树
样例
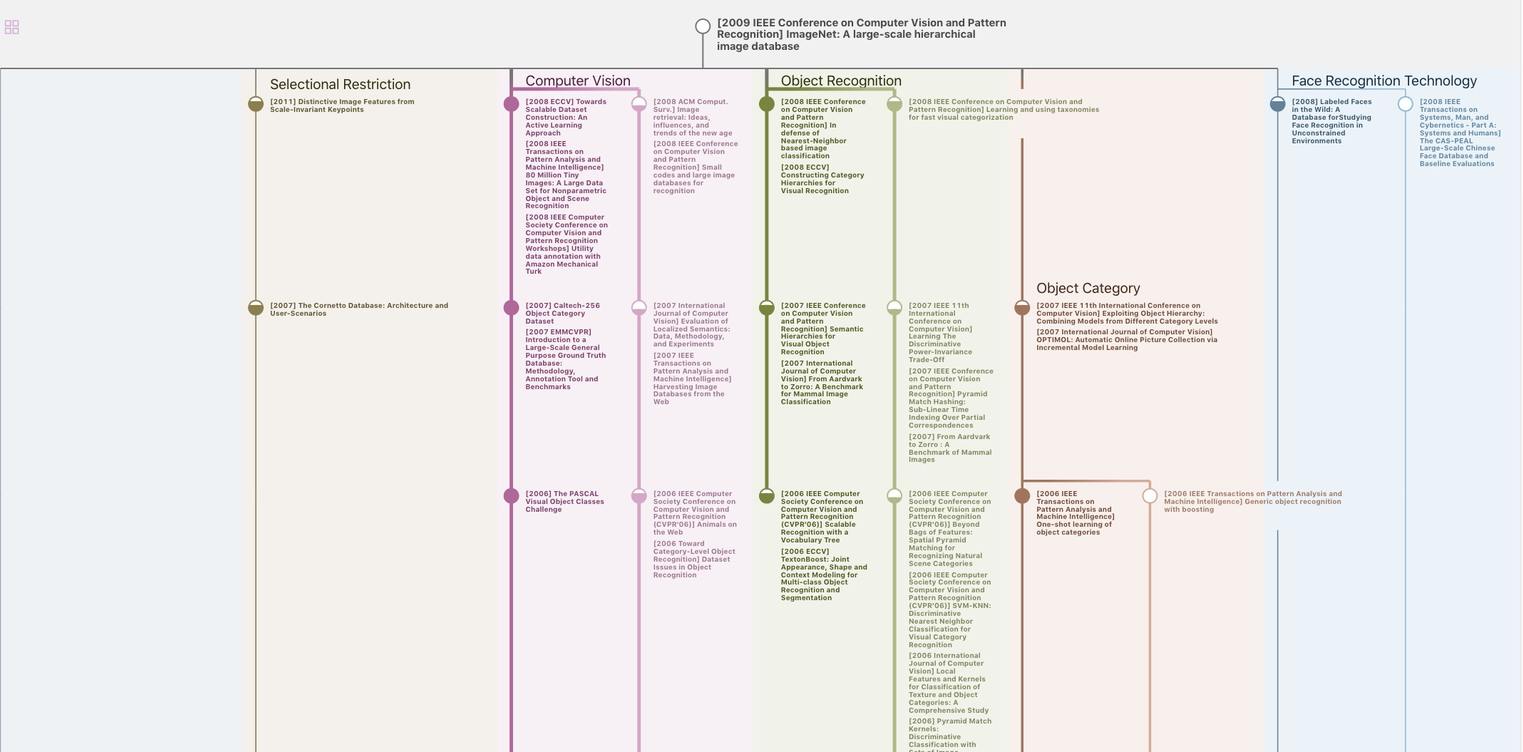
生成溯源树,研究论文发展脉络
Chat Paper
正在生成论文摘要