Software defect prediction based on kernel PCA and weighted extreme learning machine.
Information and Software Technology(2019)
摘要
Abstract Context Software defect prediction strives to detect defect-prone software modules by mining the historical data. Effective prediction enables reasonable testing resource allocation, which eventually leads to a more reliable software. Objective The complex structures and the imbalanced class distribution in software defect data make it challenging to obtain suitable data features and learn an effective defect prediction model. In this paper, we propose a method to address these two challenges. Method We propose a defect prediction framework called KPWE that combines two techniques, i.e., Kernel Principal Component A nalysis ( KPCA ) and Weighted Extreme Learning Machine ( WELM ). Our framework consists of two major stages. In the first stage, KPWE aims to extract representative data features. It leverages the KPCA technique to project the original data into a latent feature space by nonlinear mapping. In the second stage, KPWE aims to alleviate the class imbalance. It exploits the WELM technique to learn an effective defect prediction model with a weighting-based scheme. Results We have conducted extensive experiments on 34 projects from the PROMISE dataset and 10 projects from the NASA dataset. The experimental results show that KPWE achieves promising performance compared with 41 baseline methods, including seven basic classifiers with KPCA, five variants of KPWE, eight representative feature selection methods with WELM, 21 imbalanced learning methods. Conclusion In this paper, we propose KPWE, a new software defect prediction framework that considers the feature extraction and class imbalance issues. The empirical study on 44 software projects indicate that KPWE is superior to the baseline methods in most cases.
更多查看译文
关键词
Feature extraction,Nonlinear mapping,Kernel principal component analysis,Weighted extreme learning machine
AI 理解论文
溯源树
样例
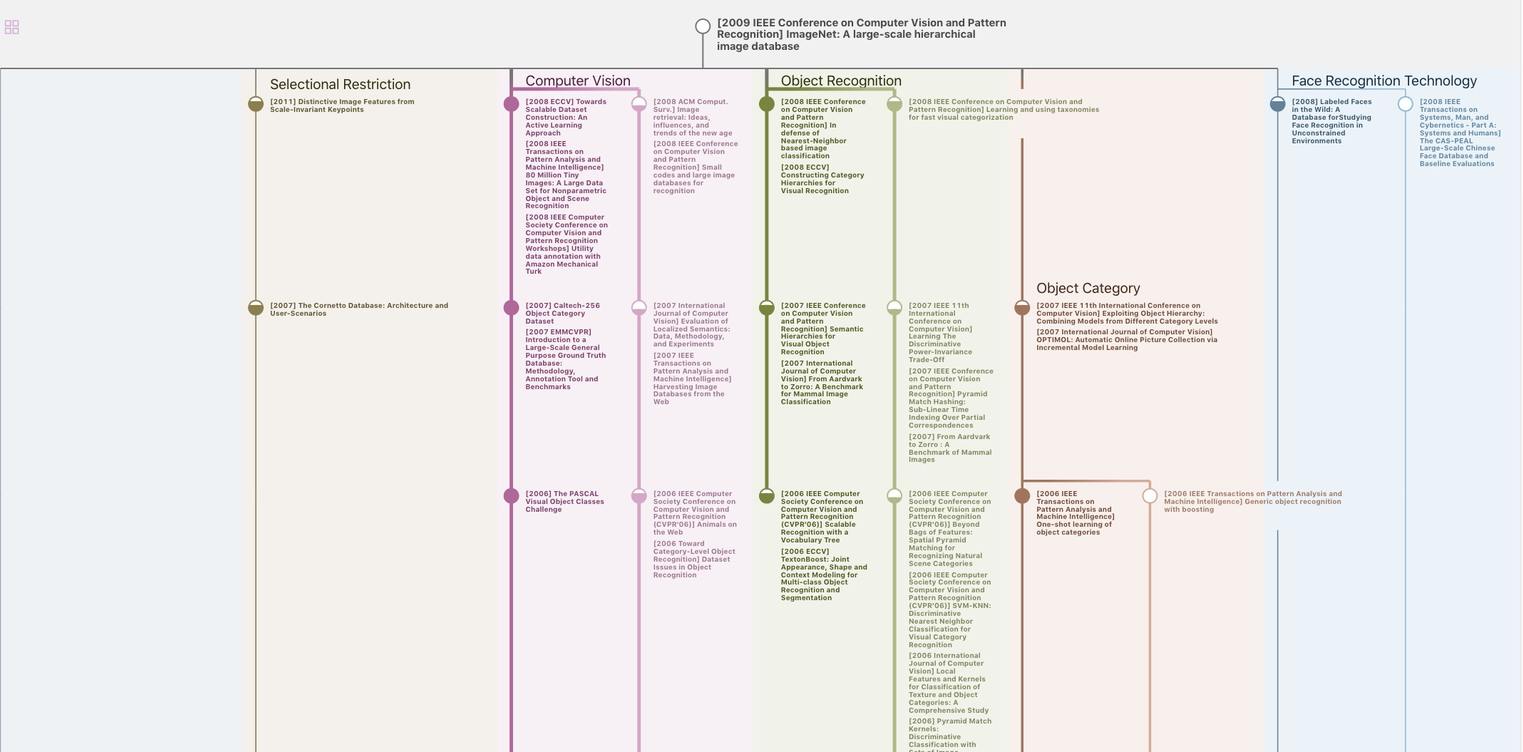
生成溯源树,研究论文发展脉络
Chat Paper
正在生成论文摘要