Hyperspectral Image Classification via Joint Sparse representation of Multi-layer Superpixles.
KSII TRANSACTIONS ON INTERNET AND INFORMATION SYSTEMS(2018)
摘要
In this paper, a novel spectral-spatial joint sparse representation algorithm for hyperspectral image classification is proposed based on multi-layer superpixels in various scales. Superpixels of various scales can provide complete yet redundant correlated information of the class attribute for test pixels. Therefore, we design a joint sparse model for a test pixel by sampling similar pixels from its corresponding superpixels combinations. Firstly, multi-layer superpixels are extracted on the false color image of the HSI data by principal components analysis model. Secondly, a group of discriminative sampling pixels are exploited as reconstruction matrix of test pixel which can be jointly represented by the structured dictionary and recovered sparse coefficients. Thirdly, the orthogonal matching pursuit strategy is employed for estimating sparse vector for the test pixel. In each iteration, the approximation can be computed from the dictionary and corresponding sparse vector. Finally, the class label of test pixel can be directly determined with minimum reconstruction error between the reconstruction matrix and its approximation. The advantages of this algorithm lie in the development of complete neighborhood and homogeneous pixels to share a common sparsity pattern, and it is able to achieve more flexible joint sparse coding of spectral-spatial information. Experimental results on three real hyperspectral datasets show that the proposed joint sparse model can achieve better performance than a series of excellent sparse classification methods and superpixels-based classification methods.
更多查看译文
关键词
Hyperspectral image classification,Multi-layer superpixels,Joint sparse representation,Discriminative optimization sampling,Reconstruction matrix
AI 理解论文
溯源树
样例
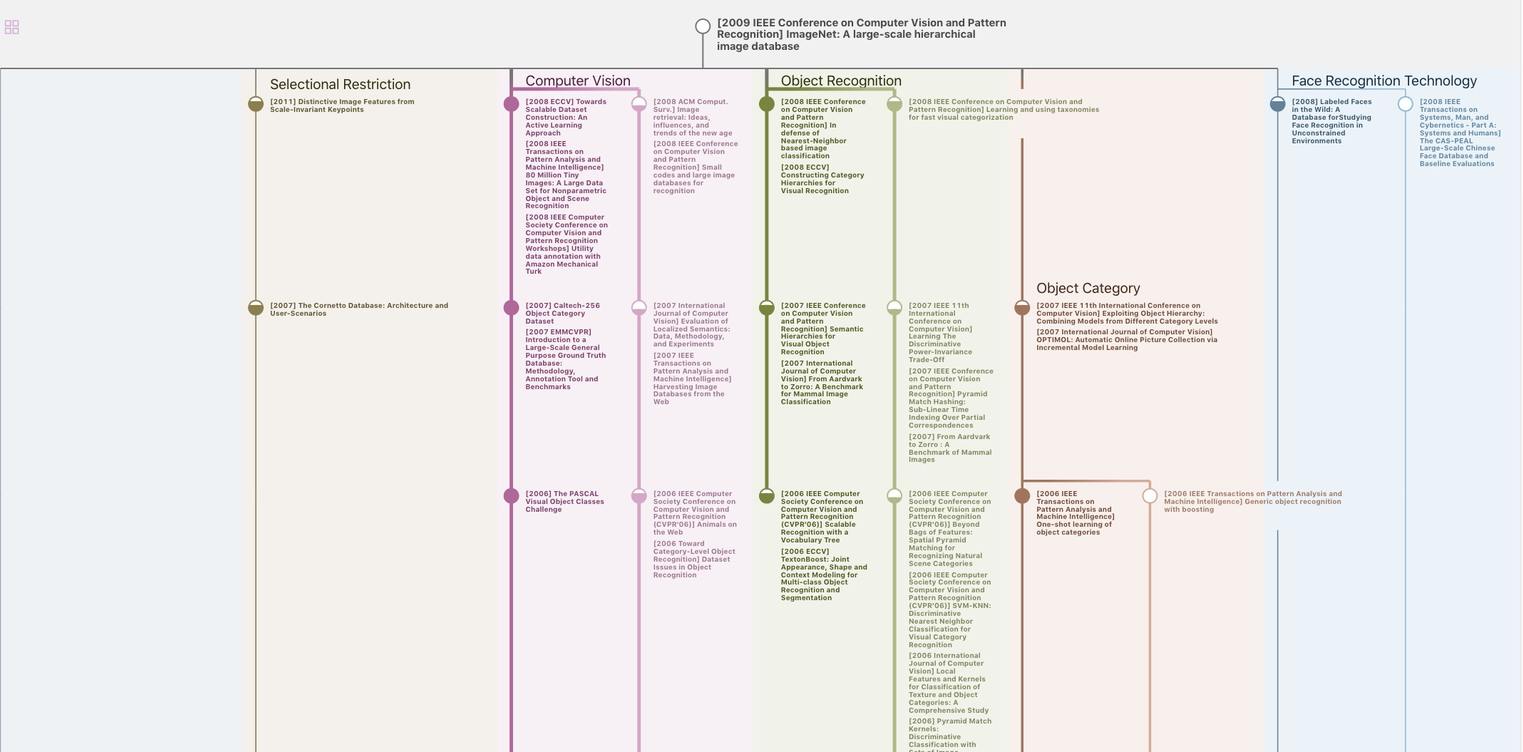
生成溯源树,研究论文发展脉络
Chat Paper
正在生成论文摘要