Computing Curvature For Volume Of Fluid Methods Using Machine Learning
JOURNAL OF COMPUTATIONAL PHYSICS(2019)
摘要
In spite of considerable progress, computing curvature in Volume of Fluid (VOF) methods continues to be a challenge. The goal is to develop a function or a subroutine that returns the curvature in computational cells containing an interface separating two immiscible fluids, given the volume fraction in the cell and the adjacent cells. Currently, the most accurate approach is to fit a curve (2D), or a surface (3D), matching the volume fractions and finding the curvature by differentiation. Here, a different approach is examined. A synthetic data set, relating curvature to volume fractions, is generated using well-defined shapes where the curvature and volume fractions are easily found and then machine learning is used to fit the data (training). The resulting function is used to find the curvature for shapes not used for the training and implemented into a code to track moving interfaces. The results suggest that using machine learning to generate the relationship is a viable approach that results in reasonably accurate predictions. (C) 2018 Elsevier Inc. All rights reserved.
更多查看译文
关键词
Machine learning,Volume of fluid,Curvature,Interface,Two-phase flow
AI 理解论文
溯源树
样例
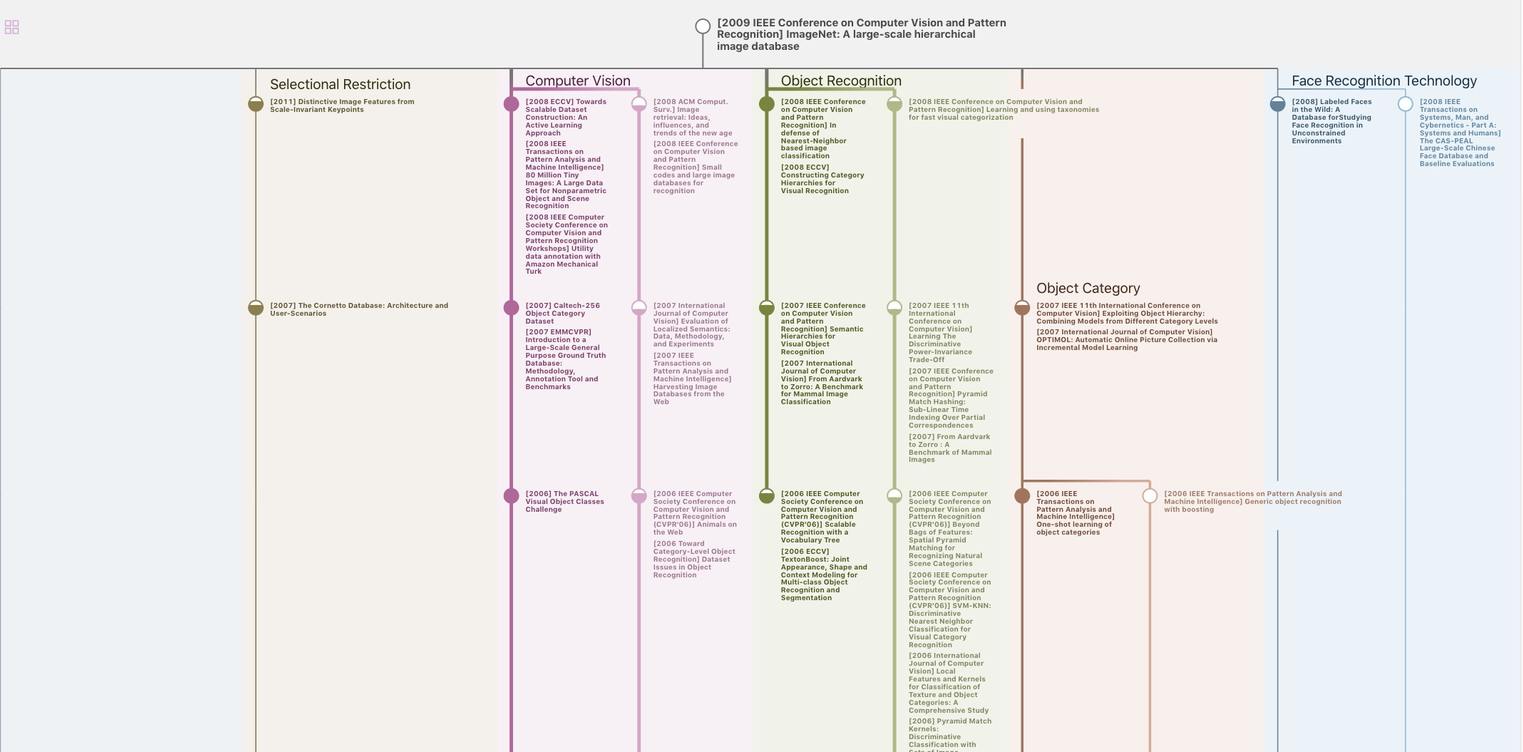
生成溯源树,研究论文发展脉络
Chat Paper
正在生成论文摘要