Considering online consumer reviews to predict movie box-office performance between the years 2009 and 2014 in the US.
ELECTRONIC LIBRARY(2018)
摘要
Purpose The purpose of this paper is to combine basic movie information factors, external factors and review factors, to predict box-office performance and identify the most crucial factor of influence for box-office performance. Design/methodology/approach Five movie genres and first-week movie reviews found on IMDb were collected. The movie reviews were quantified using sentiment analysis tools SentiStrength and Stanford CoreNLP, in which quantified data were combined with basic movie information and external environment factors to predict movie box-office performance. A movie box-office performance prediction model was then developed using data mining (DM) technologies with M5 model trees (M5P), linear regression (LR) and support vector regression (SVR), after which movie box-office performance predictions were made. Findings The results of this paper showed that the inclusion of movie reviews generated more accurate prediction results. Concerning movie review-related factors, the one that exhibited the greatest effect on box-office performance was the number of movie reviews made, whereas movie review content only displayed an effect on box-office performance for specific movie genres. Research limitations/implications Because this paper collected movie data from the IMDb, the data were limited and primarily consisted of movies released in the USA; data pertaining to less popular movies or those released outside of the USA were, thus, insufficient. Practical implications This paper helps to verify whether the consideration of the features extracted from movie reviews can improve the performance of movie box-office. Originality/value Through various DM technologies, this paper shows that movie reviews enhanced the accuracy of box-office performance predictions and the content of movie reviews has an effect on box-office performance.
更多查看译文
关键词
Online reviews,Machine learning,Box-office predictions,IMDb,Online consumer reviews
AI 理解论文
溯源树
样例
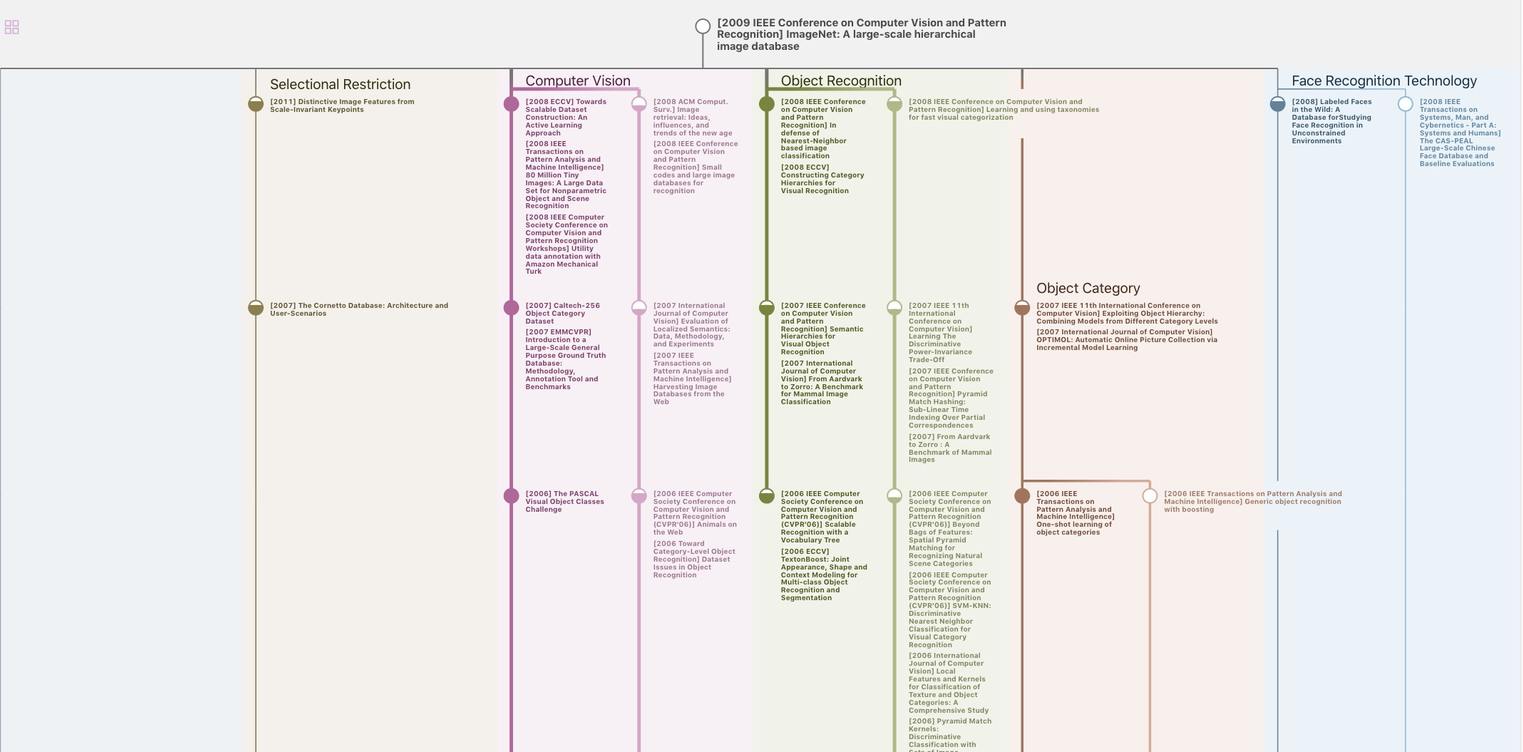
生成溯源树,研究论文发展脉络
Chat Paper
正在生成论文摘要