Cost-sensitive approximate attribute reduction with three-way decisions.
International Journal of Approximate Reasoning(2019)
摘要
In the research spectrum of rough set, the task of attribute reduction is obtaining a minimal attribute subset that preserves certain properties of the original data. Cost-sensitive attribute reduction aims at minimizing various types of costs. Approximate attribute reduction allows decision makers to leverage the advantages of knowledge discovery and their own preferences. This paper proposes the cost-sensitive approximate attribute reduction problem under both qualitative and quantitative criteria. The qualitative criterion refers to the indiscernibility, while the quantitative criterion refers to the approximate parameter ε and the cost. We present a framework based on three-way decisions and discernibility matrix to handle this new problem. First, a quality function for attribute subsets is designed with the interpretation of a hierarchical granular structure. Second, a fitness function is designed for cost performance index by investigating attribute significance. Third, three-way decision theory is applied to partition the attributes into three groups based on the fitness function and a threshold pair (α,β). Finally, deletion-based and addition-based cost-sensitive approximate reduction algorithms are designed under this framework. Experimental results indicate that our algorithms outperform the state-of-the-art methods.
更多查看译文
关键词
Attribute reduction,Cost-sensitive learning,(In)discernibility,Granular computing,Three-way decisions
AI 理解论文
溯源树
样例
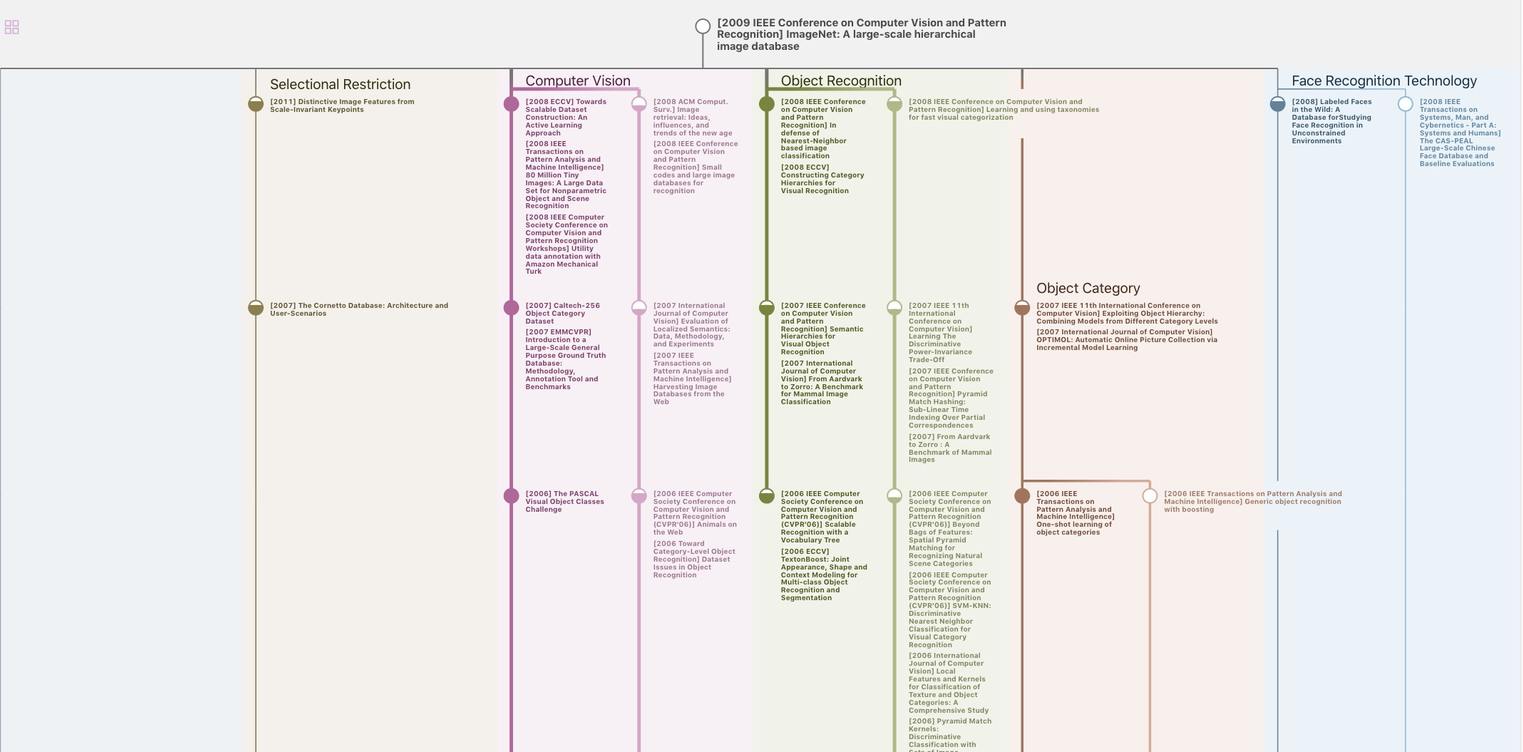
生成溯源树,研究论文发展脉络
Chat Paper
正在生成论文摘要