Semi-Supervised Dictionary Learning Based on Atom Graph Regularization
2018 IEEE INTERNATIONAL CONFERENCE ON BIG DATA (BIG DATA)(2018)
摘要
In this paper, we propose a novel unified optimization framework for semi-supervised dictionary learning, which optimizes a graph Laplacian component and the dictionary simultaneously. In the framework, the graph Laplacian is defined on the atoms and the corresponding sparse codings. Since the atoms are more concise and representative than the original training samples, the constructed graph Laplacian can not only effectively capture the manifold structure of training samples, but also be more robust to noise and outliers. Moreover, the dictionary and the graph Laplacian can facilitate each other during the learning iterations. We derive an efficient algorithm by combining the block coordinate descent method with the alternating direction method of multipliers to solve the unified optimization problem. Extensive experimental evaluation on several challenging datasets demonstrates the superior performance of the proposed method.
更多查看译文
关键词
atom graph regularization,dictionary learning,semi-supervised learning,image classification
AI 理解论文
溯源树
样例
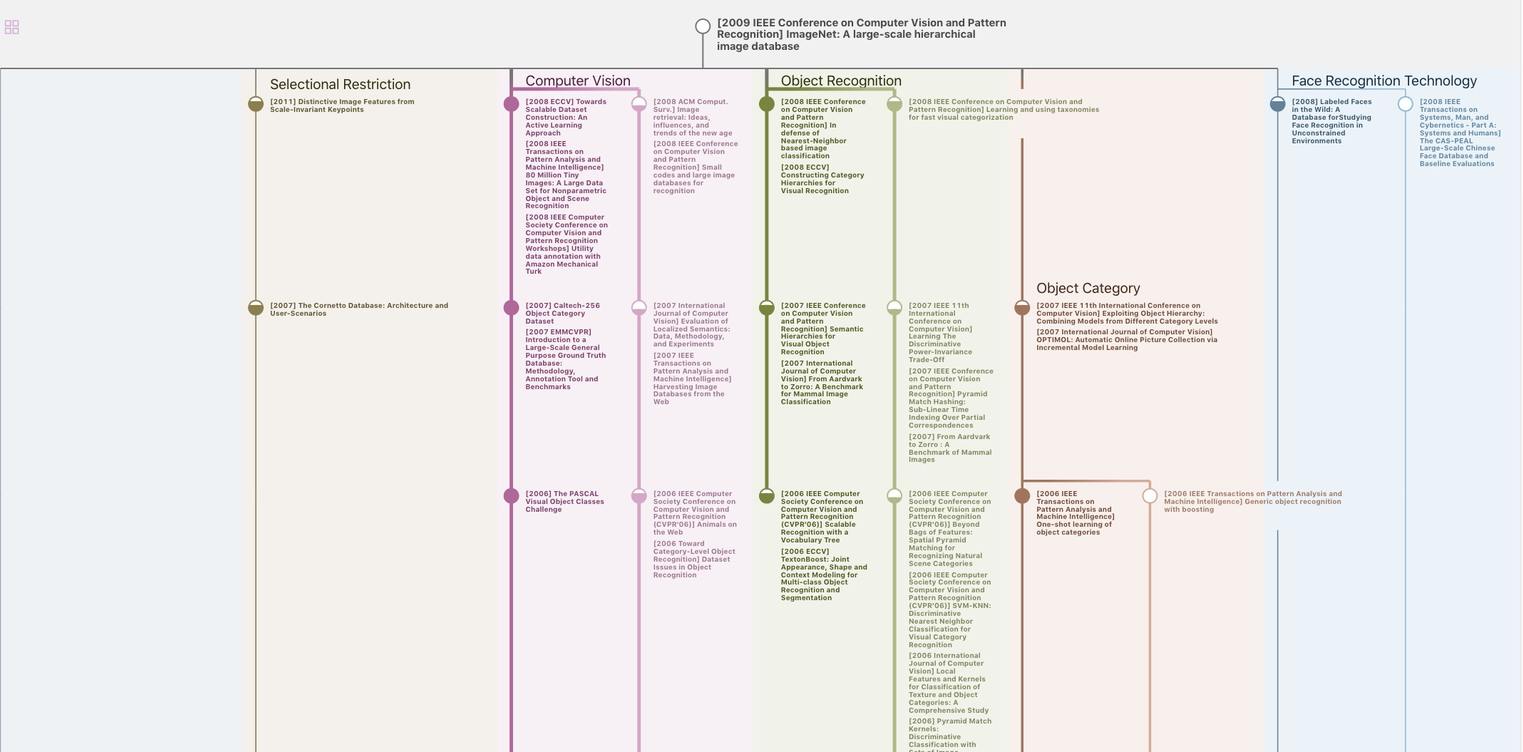
生成溯源树,研究论文发展脉络
Chat Paper
正在生成论文摘要