Cryo-electron microscope image denoising based on the geodesic distance
BMC structural biology(2018)
摘要
Background To perform a three-dimensional (3-D) reconstruction of electron cryomicroscopy (cryo-EM) images of viruses, it is necessary to determine the similarity of image blocks of the two-dimensional (2-D) projections of the virus. The projections containing high resolution information are typically very noisy. Instead of the traditional Euler metric, this paper proposes a new method, based on the geodesic metric, to measure the similarity of blocks. Results Our method is a 2-D image denoising approach. A data set of 2243 cytoplasmic polyhedrosis virus (CPV) capsid particle images in different orientations was used to test the proposed method. Relative to Block-matching and three-dimensional filtering (BM3D), Stein’s unbiased risk estimator (SURE), Bayes shrink and K-means singular value decomposition (K-SVD), the experimental results show that the proposed method can achieve a peak signal-to-noise ratio (PSNR) of 45.65. The method can remove the noise from the cryo-EM image and improve the accuracy of particle picking. Conclusions The main contribution of the proposed model is to apply the geodesic distance to measure the similarity of image blocks. We conclude that manifold learning methods can effectively eliminate the noise of the cryo-EM image and improve the accuracy of particle picking.
更多查看译文
关键词
Electron cryomicroscopy,Geodesic distance,Similar block,Image denoising,Particle picking,Manifold learning
AI 理解论文
溯源树
样例
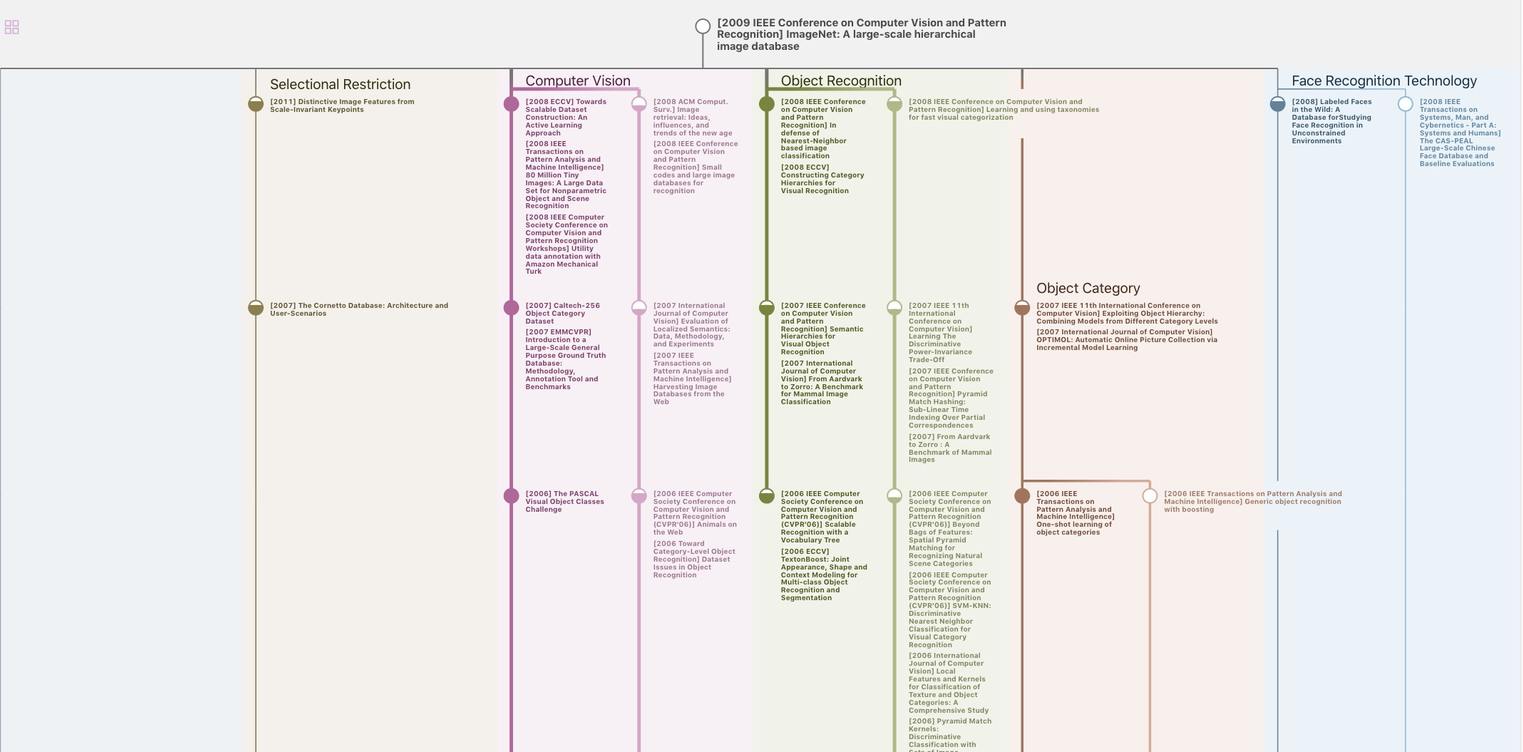
生成溯源树,研究论文发展脉络
Chat Paper
正在生成论文摘要