Predicting Patient-ventilator Asynchronies with Hidden Markov Models
SCIENTIFIC REPORTS(2018)
摘要
In mechanical ventilation, it is paramount to ensure the patient’s ventilatory demand is met while minimizing asynchronies. We aimed to develop a model to predict the likelihood of asynchronies occurring. We analyzed 10,409,357 breaths from 51 critically ill patients who underwent mechanical ventilation >24 h. Patients were continuously monitored and common asynchronies were identified and regularly indexed. Based on discrete time-series data representing the total count of asynchronies, we defined four states or levels of risk of asynchronies, z1 (very-low-risk) – z4 (very-high-risk). A Poisson hidden Markov model was used to predict the probability of each level of risk occurring in the next period. Long periods with very few asynchronous events, and consequently very-low-risk, were more likely than periods with many events (state z4). States were persistent; large shifts of states were uncommon and most switches were to neighbouring states. Thus, patients entering states with a high number of asynchronies were very likely to continue in that state, which may have serious implications. This novel approach to dealing with patient-ventilator asynchrony is a first step in developing smart alarms to alert professionals to patients entering high-risk states so they can consider actions to improve patient-ventilator interaction.
更多查看译文
关键词
Patient-ventilator Asynchrony,Hidden Markov Models (HMM),Poor Patient-ventilator Interaction,Ineffective Inspiratory Efforts,Double Cycling
AI 理解论文
溯源树
样例
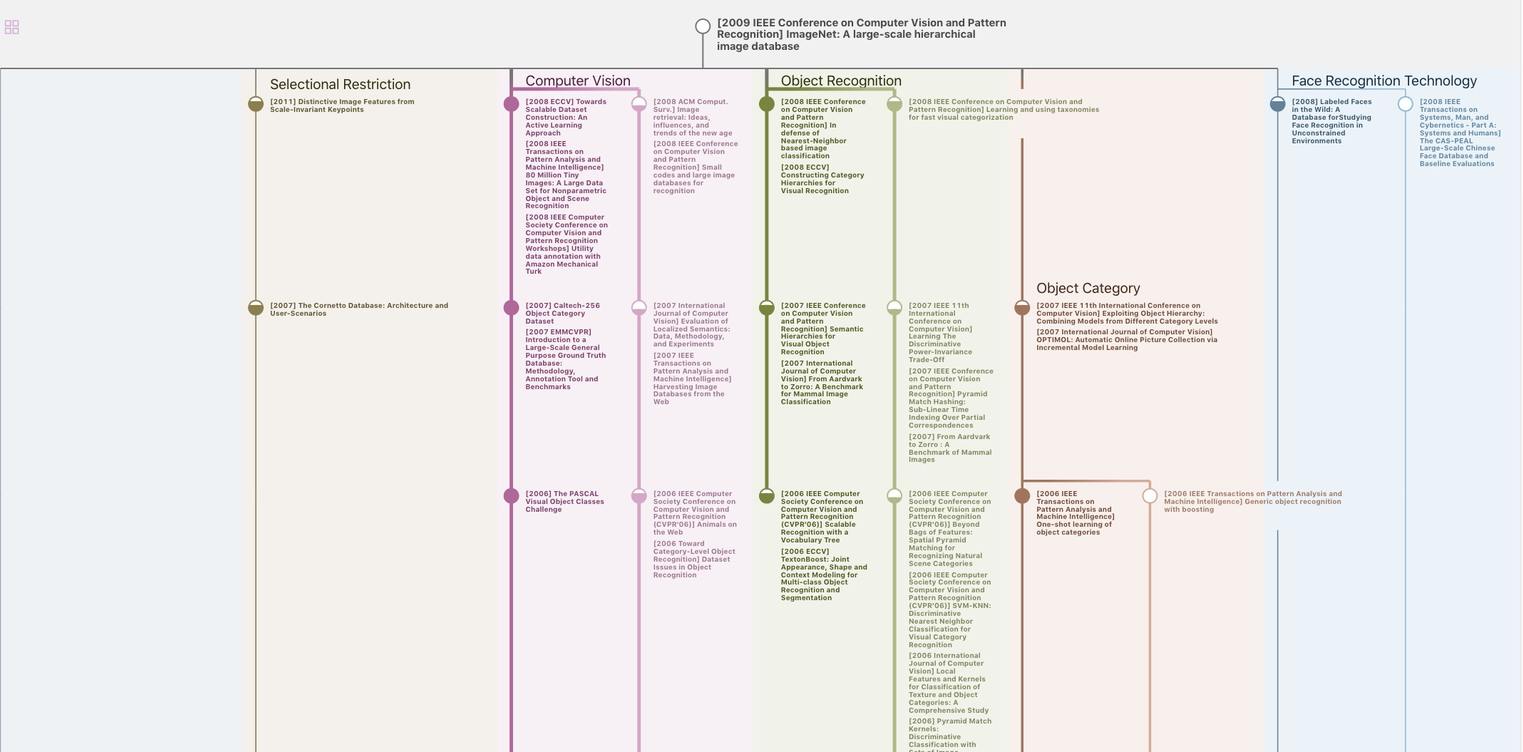
生成溯源树,研究论文发展脉络
Chat Paper
正在生成论文摘要