Accuracy of climate-based forecasts of pathogen spread.
ROYAL SOCIETY OPEN SCIENCE(2017)
摘要
Species distribution models (SDMs) are a tool for predicting the eventual geographical range of an emerging pathogen. Most SDMs, however, rely on an assumption of equilibrium with the environment, which an emerging pathogen, by definition, has not reached. To determine if some SDM approaches work better than others for modelling the spread of emerging, non-equilibrium pathogens, we studied time-sensitive predictive performance of SDMs for Batrachochytrium dendrobatidis, a devastating infectious fungus of amphibians, using multiple methods trained on time-incremented subsets of the available data. We split our data into timeline-based training and testing sets, and evaluated models on each set using standard performance criteria, including AUC, kappa, false negative rate and the Boyce index. Of eight models examined, we found that boosted regression trees and random forests performed best, closely followed by MaxEnt. As expected, predictive performance generally improved with the length of time series used for model training. These results provide information on how quickly the potential extent of an emerging disease may be determined, and identify which modelling frameworks are likely to provide useful information during the early phases of pathogen expansion.
更多查看译文
关键词
species distribution model,Batrachochytrium dendrobatidis,machine learning,hindcasting
AI 理解论文
溯源树
样例
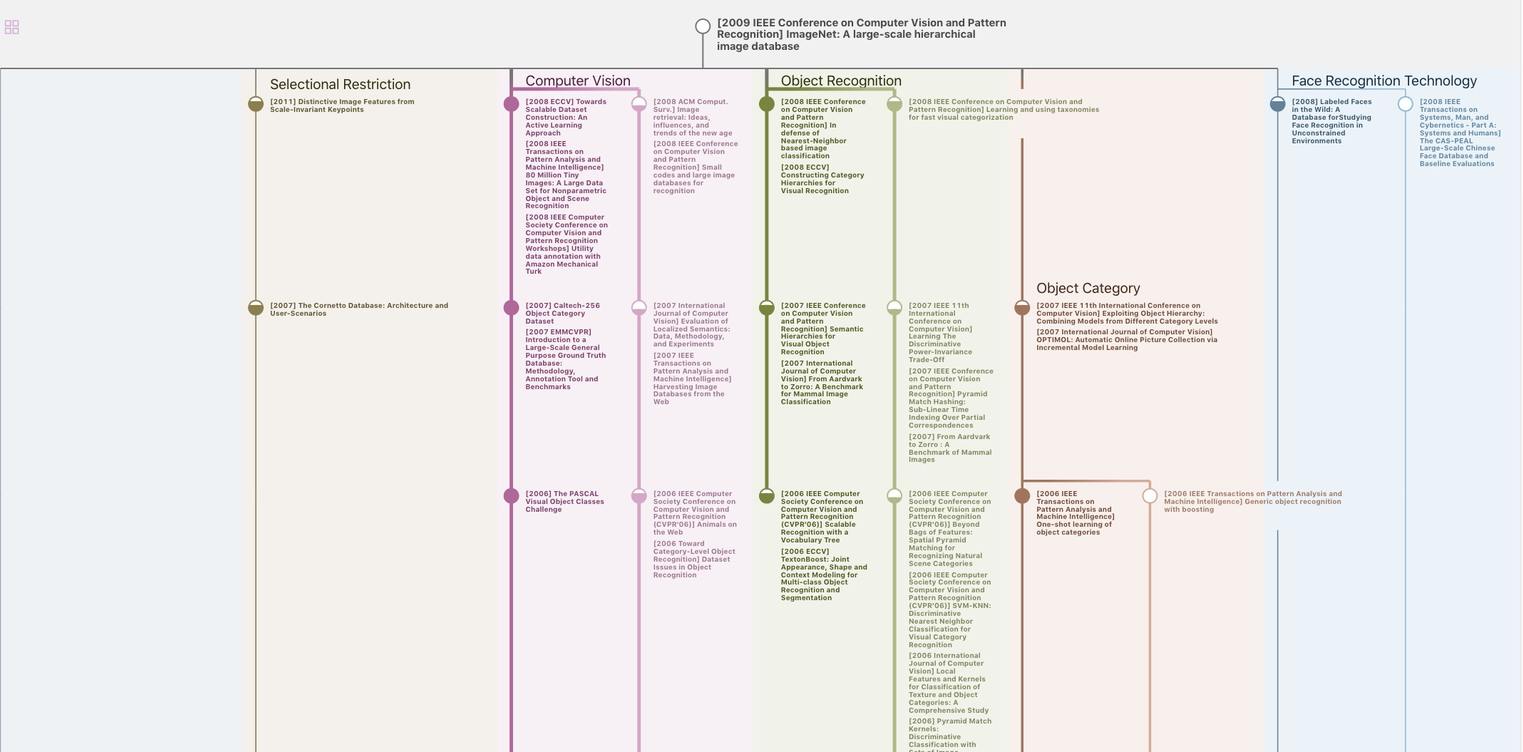
生成溯源树,研究论文发展脉络
Chat Paper
正在生成论文摘要