Sparse random similarity feature decomposition and its application in gear fault diagnosis
MEASUREMENT SCIENCE AND TECHNOLOGY(2023)
摘要
As a new signal decomposition method, sparse random mode decomposition (SRMD) does not depend on the signal sampling process and has good anti-mode aliasing performance. However, SRMD needs preset decomposition parameters and cannot perform accurate decomposition for strong noise interference signals. Thus, this paper proposes a sparse random similarity feature decomposition (SRSFD) method to overcome the problems of SRMD. Firstly, SRSFD utilizes the windowed sinusoidal function to randomly generate time-frequency features. Then, by solving sparse optimization problem, the sparse random feature approximation of the input signal can be obtained and its corresponding time-frequency features can be applied to reconstruct the initial signal components. Finally, the initial signal components are regrouped by using periodic similarity to obtain the final components. The analytical results of simulation and actual gear fault signals show that SRSFD can effectively achieve adaptive signal decomposition and has better decomposition performance and noise robustness compared with existing methods.
更多查看译文
关键词
sparse random similarity feature decomposition,sparse optimization,periodic similarity,gear fault diagnosis
AI 理解论文
溯源树
样例
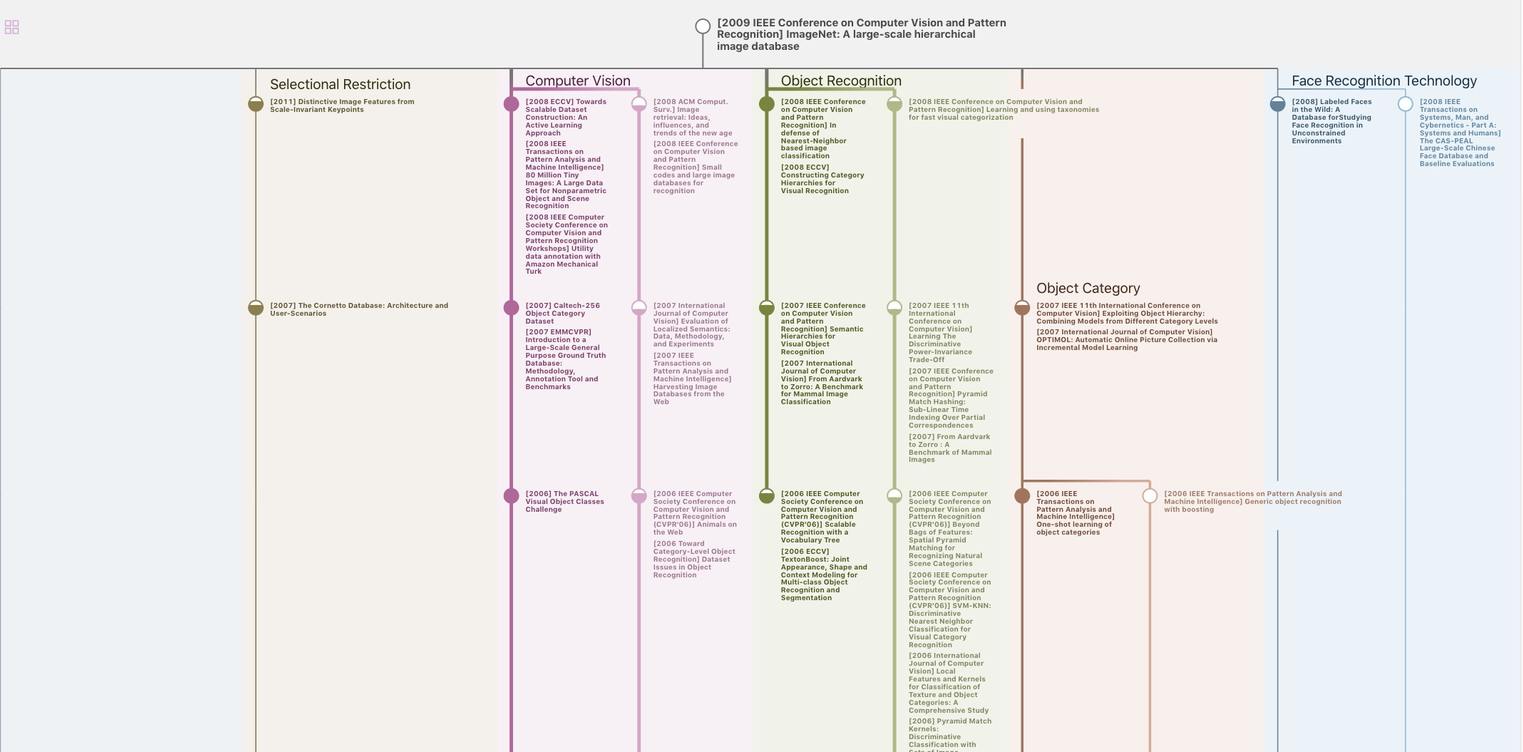
生成溯源树,研究论文发展脉络
Chat Paper
正在生成论文摘要