Efficient liver segmentation in CT images based on graph cuts and bottleneck detection
Physica Medica(2016)
摘要
Liver segmentation from abdominal computed tomography (CT) volumes is extremely important for computer-aided liver disease diagnosis and surgical planning of liver transplantation. Due to ambiguous edges, tissue adhesion, and variation in liver intensity and shape across patients, accurate liver segmentation is a challenging task. In this paper, we present an efficient semi-automatic method using intensity, local context, and spatial correlation of adjacent slices for the segmentation of healthy liver regions in CT volumes. An intensity model is combined with a principal component analysis (PCA) based appearance model to exclude complex background and highlight liver region. They are then integrated with location information from neighboring slices into graph cuts to segment the liver in each slice automatically. Finally, a boundary refinement method based on bottleneck detection is used to increase the segmentation accuracy. Our method does not require heavy training process or statistical model construction, and is capable of dealing with complicated shape and intensity variations. We apply the proposed method on XHCSU14 and SLIVER07 databases, and evaluate it by MICCAI criteria and Dice similarity coefficient. Experimental results show our method outperforms several existing methods on liver segmentation.
更多查看译文
关键词
Liver segmentation,Graph cuts,Bottleneck detection,Gaussian fitting,PCA
AI 理解论文
溯源树
样例
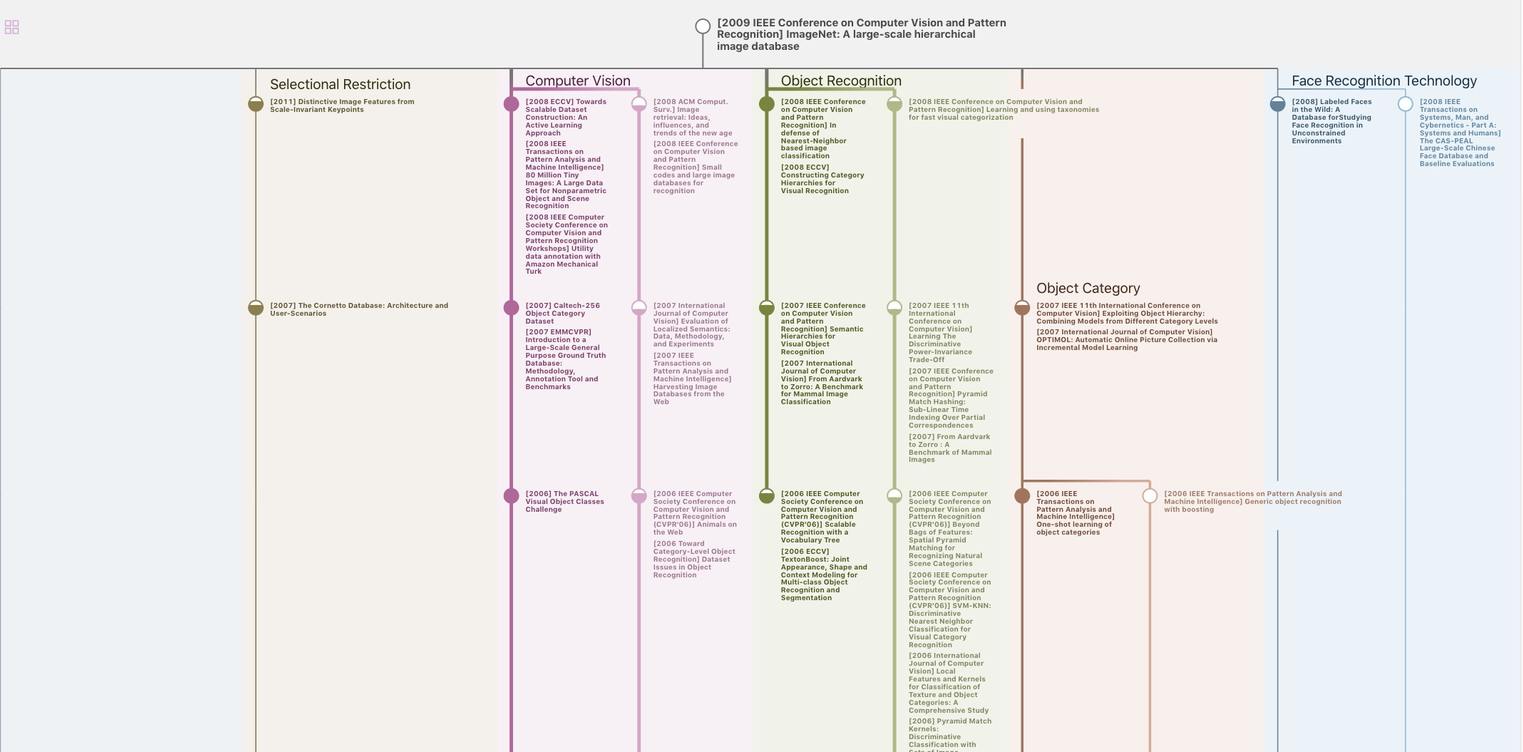
生成溯源树,研究论文发展脉络
Chat Paper
正在生成论文摘要