Supervised Filter Learning For Representation Based Face Recognition
PLOS ONE(2016)
摘要
Representation based classification methods, such as Sparse Representation Classification (SRC) and Linear Regression Classification (LRC) have been developed for face recognition problem successfully. However, most of these methods use the original face images without any preprocessing for recognition. Thus, their performances may be affected by some problematic factors (such as illumination and expression variances) in the face images. In order to overcome this limitation, a novel supervised filter learning algorithm is proposed for representation based face recognition in this paper. The underlying idea of our algorithm is to learn a filter so that the within-class representation residuals of the faces' Local Binary Pattern (LBP) features are minimized and the between-class representation residuals of the faces' LBP features are maximized. Therefore, the LBP features of filtered face images are more discriminative for representation based classifiers. Furthermore, we also extend our algorithm for heterogeneous face recognition problem. Extensive experiments are carried out on five databases and the experimental results verify the efficacy of the proposed algorithm.
更多查看译文
关键词
Sparse Representations,Feature Learning,Facial Landmark Detection,Face Recognition,Local Binary Patterns
AI 理解论文
溯源树
样例
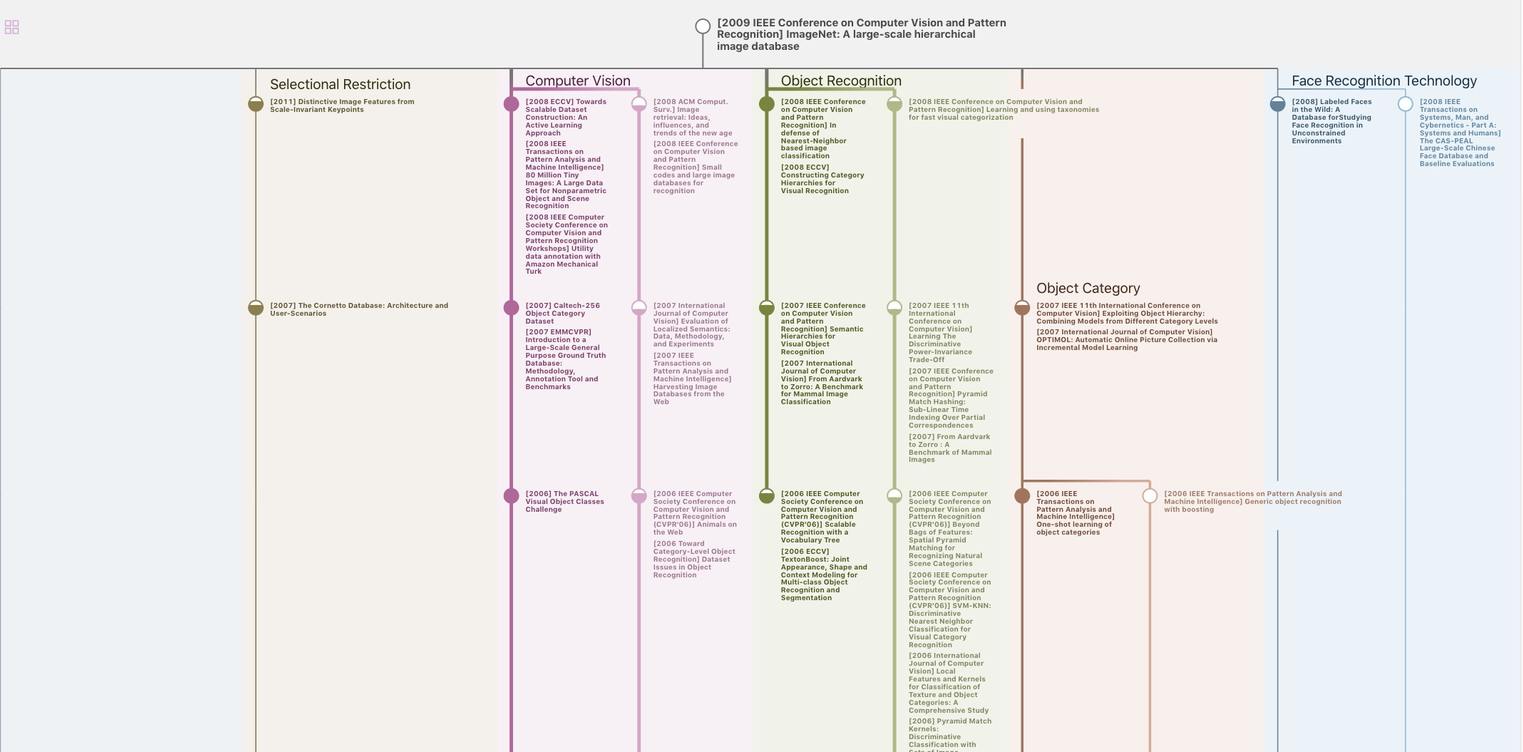
生成溯源树,研究论文发展脉络
Chat Paper
正在生成论文摘要