Misspecified poisson regression models for large-scale registry data: inference for 'large n and small p'.
STATISTICS IN MEDICINE(2016)
摘要
Poisson regression is an important tool in register-based epidemiology where it is used to study the association between exposure variables and event rates. In this paper, we will discuss the situation with 'large n and small p', where n is the sample size and p is the number of available covariates. Specifically, we are concerned with modeling options when there are time-varying covariates that can have time-varying effects. One problem is that tests of the proportional hazards assumption, of no interactions between exposure and other observed variables, or of other modeling assumptions have large power due to the large sample size and will often indicate statistical significance even for numerically small deviations that are unimportant for the subject matter. Another problem is that information on important confounders may be unavailable. In practice, this situation may lead to simple working models that are then likely misspecified. To support and improve conclusions drawn from such models, we discuss methods for sensitivity analysis, for estimation of average exposure effects using aggregated data, and a semi-parametric bootstrap method to obtain robust standard errors. The methods are illustrated using data from the Danish national registries investigating the diabetes incidence for individuals treated with antipsychotics compared with the general unexposed population. Copyright (C) 2015 John Wiley & Sons, Ltd.
更多查看译文
关键词
Poisson regression,sensitivity analysis,registry data,average exposure effect,misspecified models
AI 理解论文
溯源树
样例
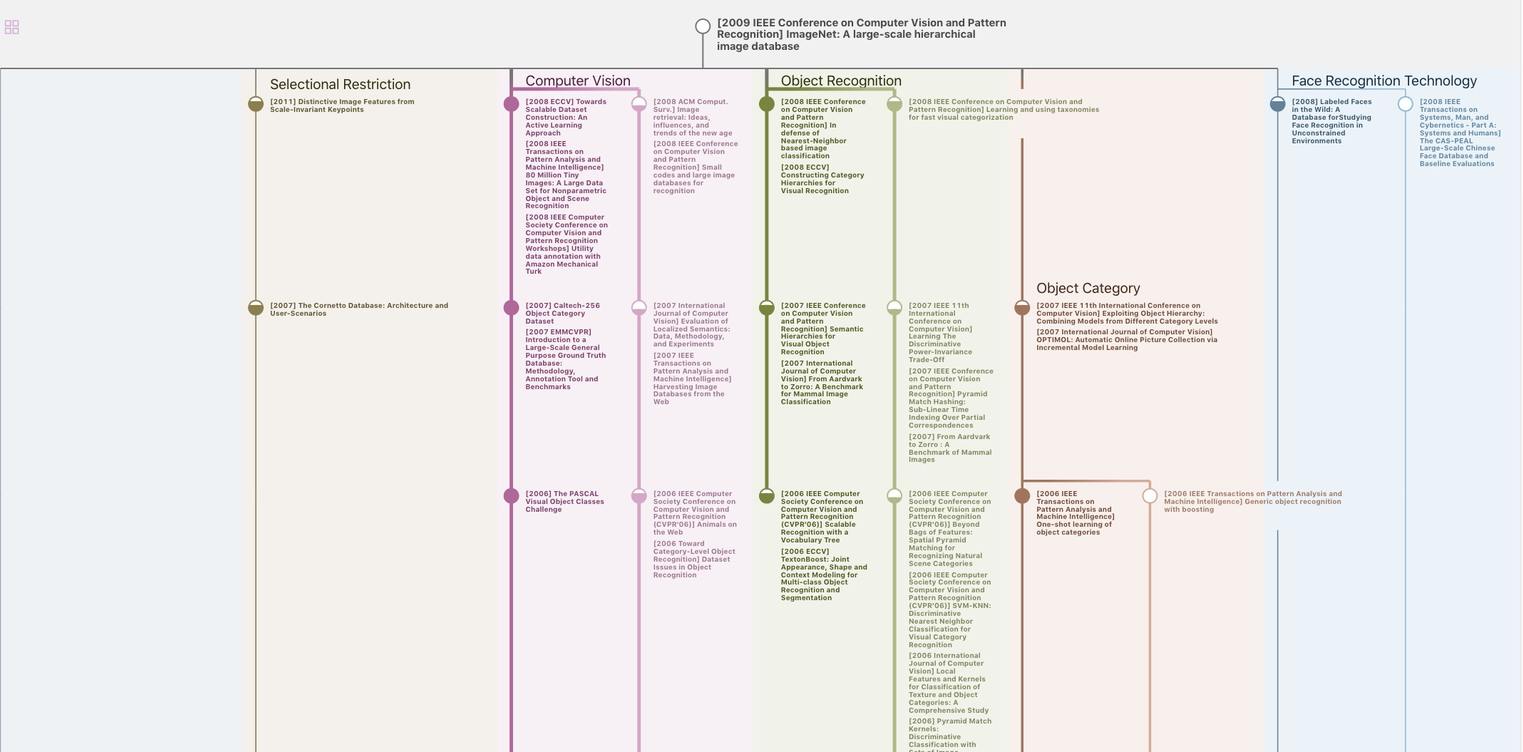
生成溯源树,研究论文发展脉络
Chat Paper
正在生成论文摘要