Rapid Identification of Disulfide Bonds and Cysteine-Related Variants in an IgG1 Knob-into-Hole Bispecific Antibody Enhanced by Machine Learning.
ANALYTICAL CHEMISTRY(2019)
摘要
Bispecific antibodies are regarded as the next generation of therapeutic modalities as they can simultaneously bind multiple targets, increasing the efficacy of treatments for several diseases and opening up previously unattainable treatment designs. Linking two half antibodies to form the knob-into-hole bispecific antibody requires an additional in vitro assembly step, starting with reduction of the antibodies and then reoxidization. Analysis of the disulfide bonds (DSBs) is vital to ensuring the correct assembly, stability, and higher order structures of these important biomolecules because incorrect disulfide bond formation and/or presence of cysteine-related post translational modifications can cause a loss of biological activity or even elicit an immune response from the host. Despite advancements in analytical methods, characterization of cysteine forms remains technically challenging and time-consuming. Herein, we report the development of an improved nonreduced peptide map method coupled with machine learning to enable rapid identification of disulfide bonds and cysteine-related variants in an IgG1 knob-into-hole bispecific antibody. The enhanced method offers a fast, consistent, and accurate workflow in mapping-out expected disulfide bonds in both half antibodies and bispecific antibodies and identifying cysteine-related modifications. Comparisons between two versions of the bispecific antibody molecule and analysis of stressed samples were also accomplished, indicating this method can be utilized to identify alterations originating from bioprocess changes and to determine the impact of assembly and postassembly stress conditions to product quality.
更多查看译文
AI 理解论文
溯源树
样例
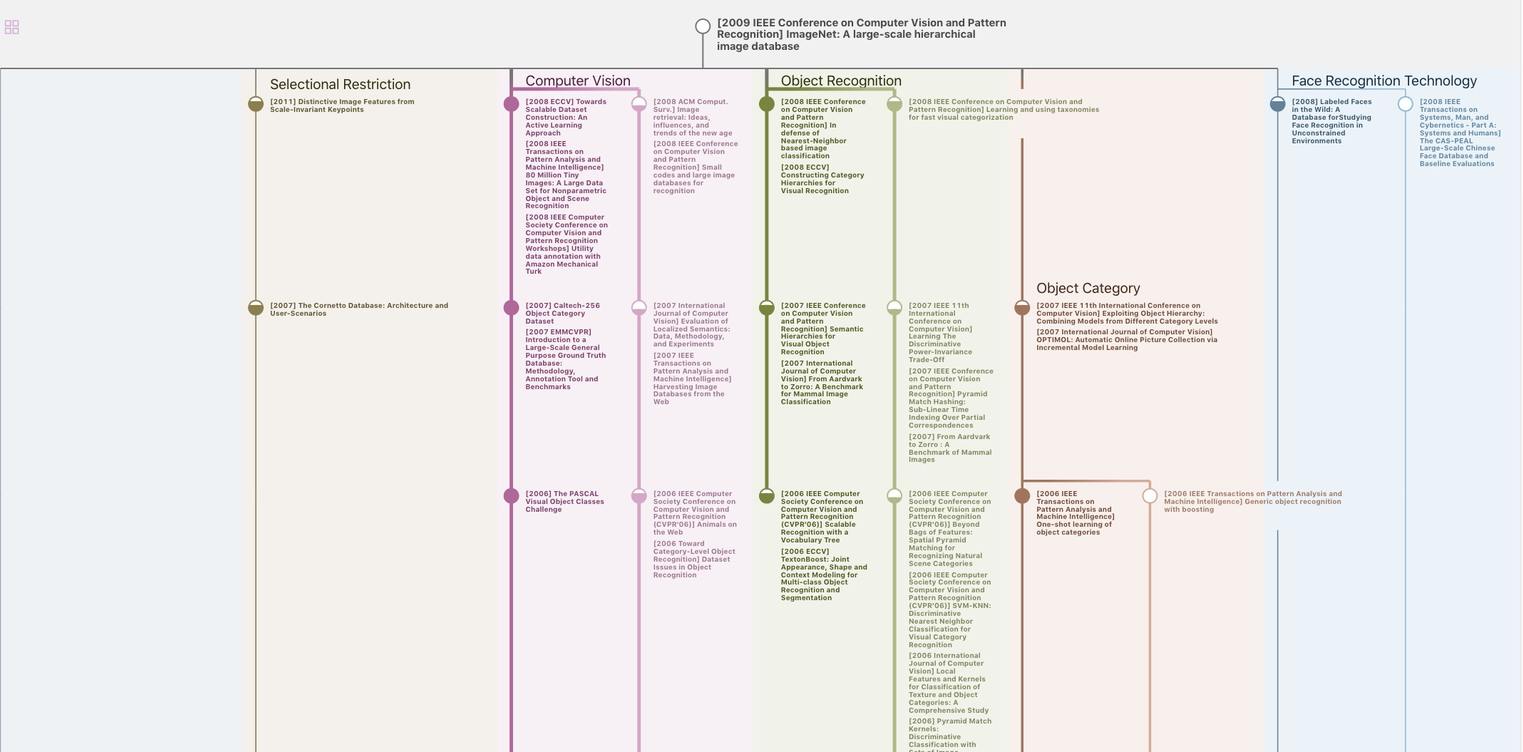
生成溯源树,研究论文发展脉络
Chat Paper
正在生成论文摘要