Computational identification of chemical compounds with potential activity against Leishmania amazonensis using nonlinear machine learning techniques.
CURRENT TOPICS IN MEDICINAL CHEMISTRY(2019)
摘要
Leishmaniasis is a poverty-related disease endemic in 98 countries worldwide, with morbidity and mortality increasing daily. All currently used first-line and second-line drugs for the treatment of leishmaniasis exhibit several drawbacks including toxicity, high costs and route of administration. Consequently, the development of new treatments for leishmaniasis is a priority in the field of neglected tropical diseases. The aim of this work is to develop computational models those allow the identification of new chemical compounds with potential anti-leishmanial activity. A data set of 116 organic chemicals, assayed against promastigotes of Leishmania amazonensis, is used to develop the theoretical models. The cutoff value to consider a compound as active one was IC50 <= 1.5 mu M. For this study, we employed Dragon software to calculate the molecular descriptors and WEKA to obtain machine learning (ML) models. All ML models showed accuracy values between 82% and 91%, for the training set. The models developed with kappa-nearest neighbors and classification trees showed sensitivity values of 97% and 100%, respectively; while the models developed with artificial neural networks and support vector machine showed specificity values of 94% and 92%, respectively. In order to validate our models, an external test-set was evaluated with good behavior for all models. A virtual screening was performed and 156 compounds were identified as potential anti-leishmanial by all the ML models. This investigation highlights the merits of ML-based techniques as an alternative to other more traditional methods to find new chemical compounds with anti-leishmanial activity.
更多查看译文
关键词
Anti-leishmanial activity,Chemical compound,L. amazonensis,ML-based model,Virtual screening,WEKA
AI 理解论文
溯源树
样例
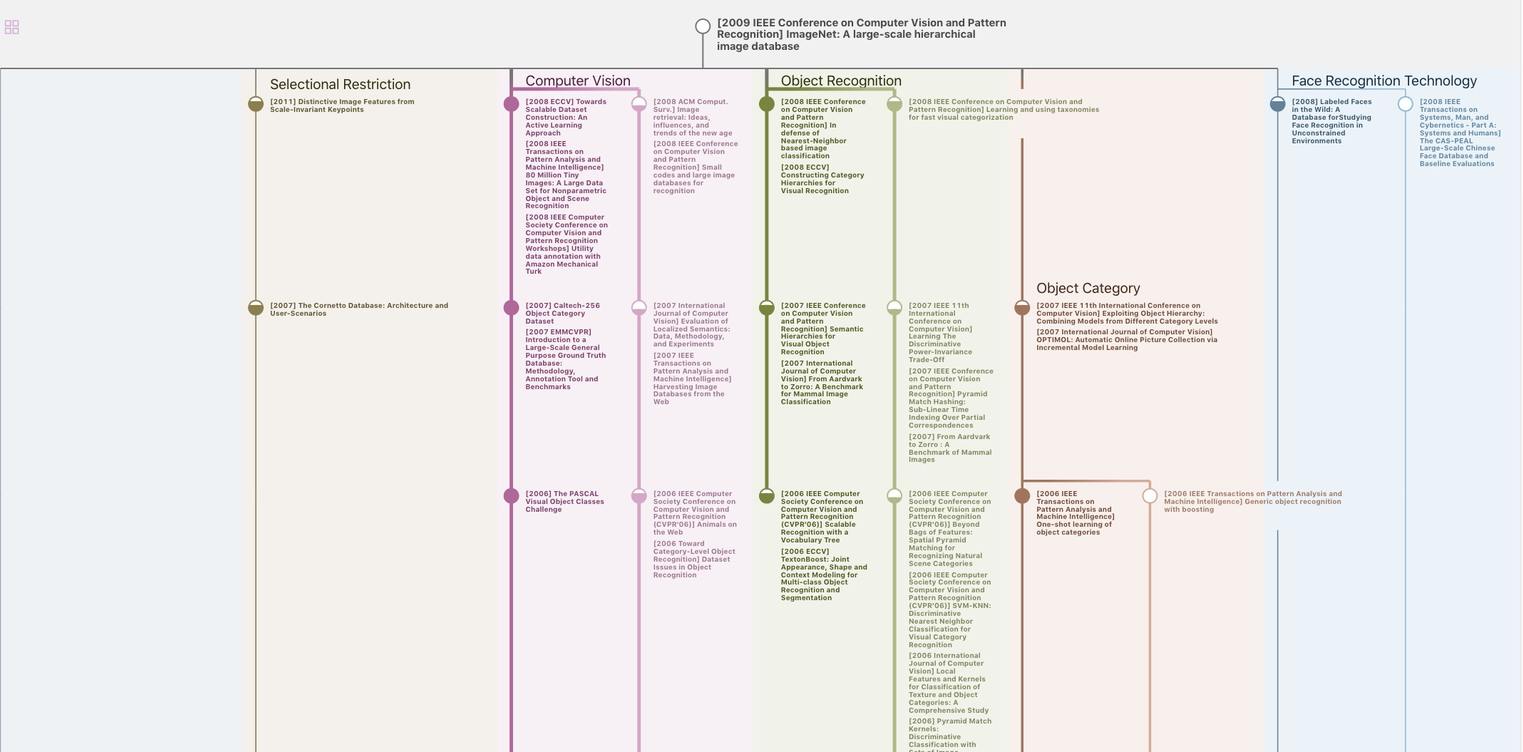
生成溯源树,研究论文发展脉络
Chat Paper
正在生成论文摘要