Advances with support vector machines for novel drug discovery.
EXPERT OPINION ON DRUG DISCOVERY(2019)
摘要
Introduction: Novel drug discovery remains an enormous challenge, with various computer-aided drug design (CADD) approaches having been widely employed for this purpose. CADD, specifically the commonly used support vector machines (SVMs), can employ machine learning techniques. SVMs and their variations offer numerous drug discovery applications, which range from the classification of substances (as active or inactive) to the construction of regression models and the ranking/virtual screening of databased compounds. Areas covered: Herein, the authors consider some of the applications of SVMs in medicinal chemistry, illustrating their main advantages and disadvantages, as well as trends in their utilization, via the available published literature. The aim of this review is to provide an up-to-date review of the recent applications of SVMs in drug discovery as described by the literature, thereby highlighting their strengths, weaknesses, and future challenges. Expert opinion: Techniques based on SVMs are considered as powerful approaches in early drug discovery. The ability of SVMs to classify active or inactive compounds has enabled the prioritization of substances for virtual screening. Indeed, one of the main advantages of SVMs is related to their potential in the analysis of nonlinear problems. However, despite successes in employing SVMs, the challenges of improving accuracy remain.
更多查看译文
关键词
Drug discovery,medicinal chemistry,machine learning,support vector machines,QSAR,classification,virtual screening
AI 理解论文
溯源树
样例
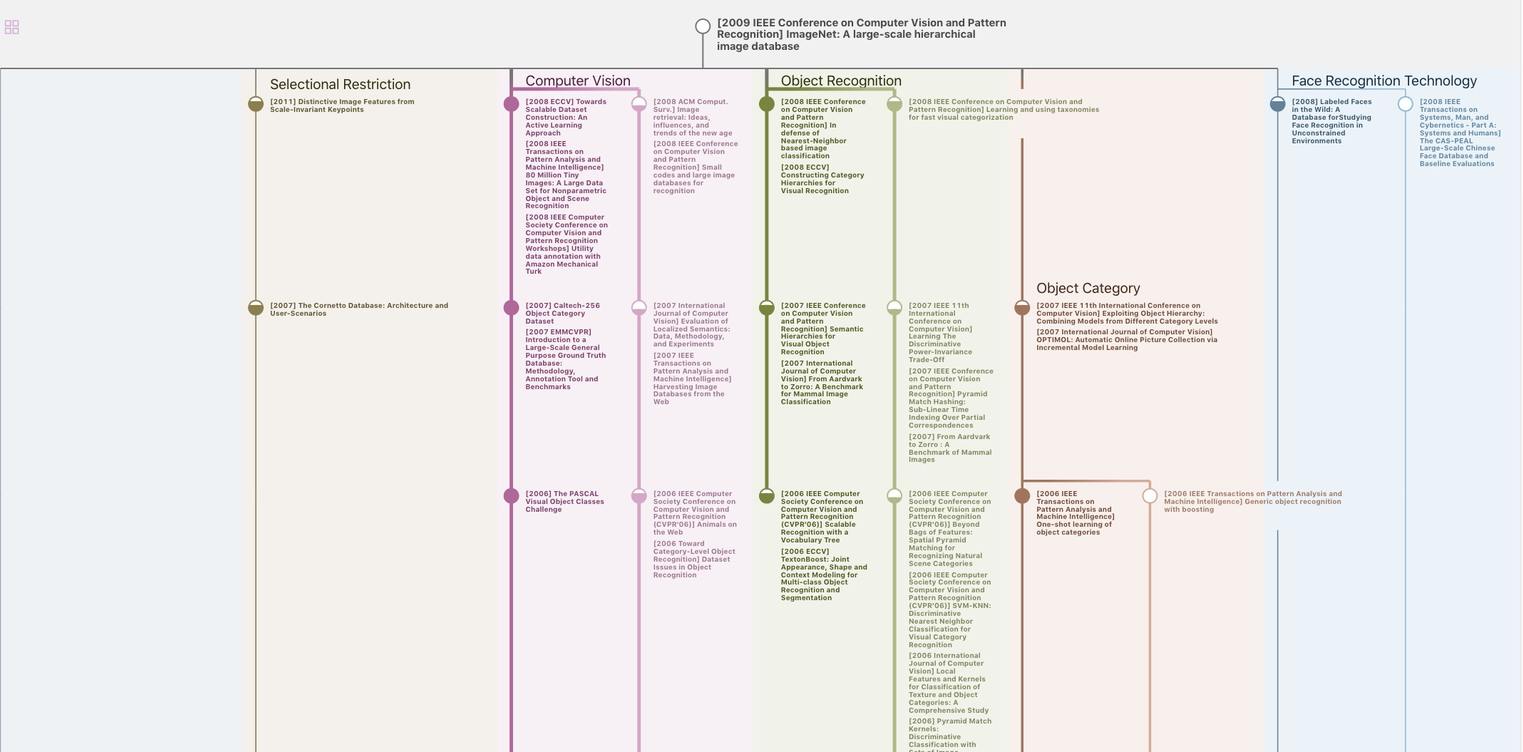
生成溯源树,研究论文发展脉络
Chat Paper
正在生成论文摘要