Multiple Sclerosis Identification by 14-Layer Convolutional Neural Network With Batch Normalization, Dropout, and Stochastic Pooling.
FRONTIERS IN NEUROSCIENCE(2018)
摘要
Aim: Multiple sclerosis is a severe brain and/or spinal cord disease. It may lead to a wide range of symptoms. Hence, the early diagnosis and treatment is quite important. Method: This study proposed a 14-layer convolutional neural network, combined with three advanced techniques: batch normalization, dropout, and stochastic pooling. The output of the stochastic pooling was obtained via sampling from a multinomial distribution formed from the activations of each pooling region. In addition, we used data augmentation method to enhance the training set. In total 10 runs were implemented with the hold-out randomly set for each run. Results: The results showed that our 14-layer CNN secured a sensitivity of 98.77 +/- 0.35%, a specificity of 98.76 +/- 0.58%, and an accuracy of 98.77 +/- 0.39%. Conclusion: Our results were compared with CNN using maximum pooling and average pooling. The comparison shows stochastic pooling gives better performance than other two pooling methods. Furthermore, we compared our proposed method with six state-of-the-art approaches, including five traditional artificial intelligence methods and one deep learning method. The comparison shows our method is superior to all other six state-of-the-art approaches.
更多查看译文
关键词
multiple sclerosis,deep learning,convolutional neural network,batch normalization,dropout,stochastic pooling
AI 理解论文
溯源树
样例
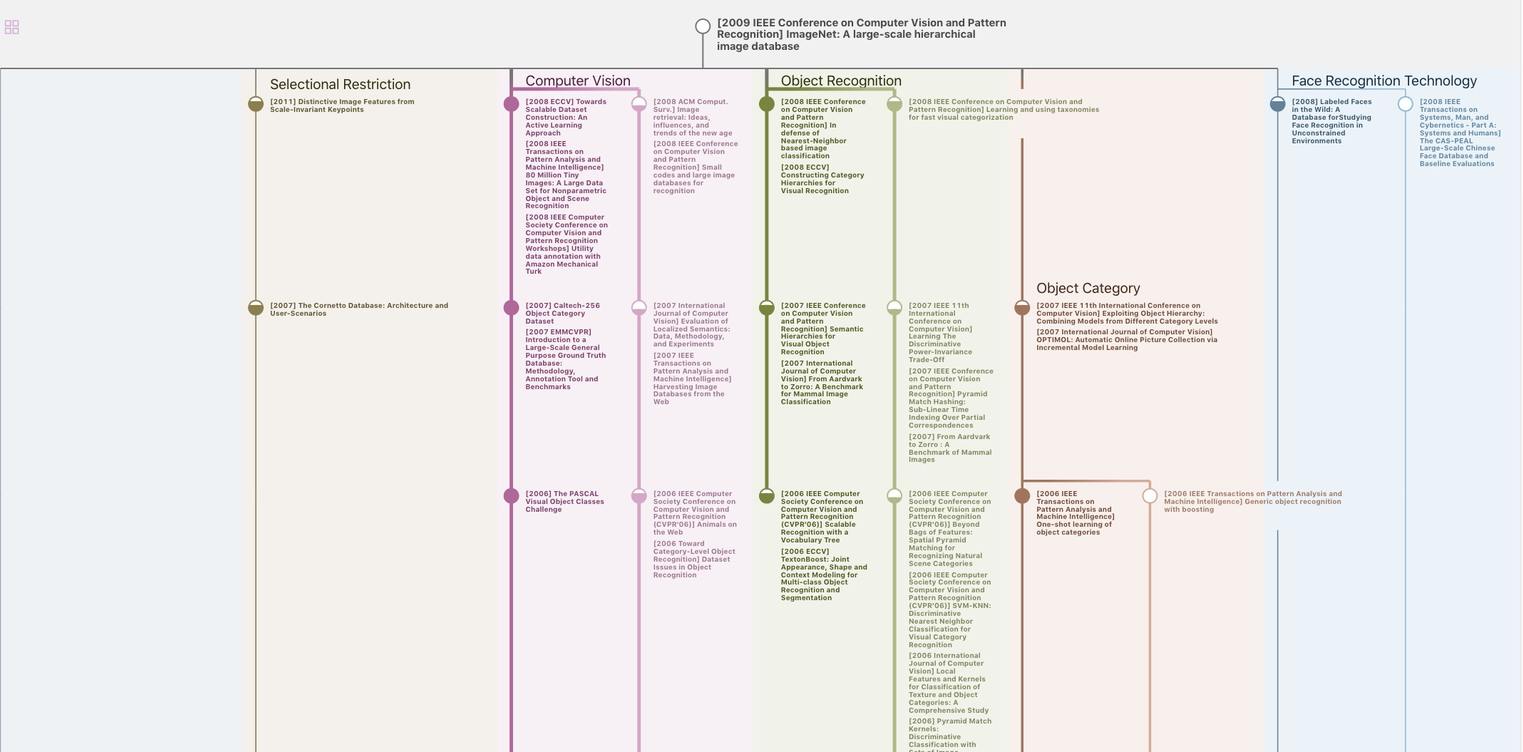
生成溯源树,研究论文发展脉络
Chat Paper
正在生成论文摘要