Neural Reconstruction Integrity: A Metric for Assessing the Connectivity Accuracy of Reconstructed Neural Networks.
FRONTIERS IN NEUROINFORMATICS(2018)
摘要
Neuroscientists are actively pursuing high-precision maps, or graphs consisting of networks of neurons and connecting synapses in mammalian and non-mammalian brains. Such graphs, when coupled with physiological and behavioral data, are likely to facilitate greater understanding of how circuits in these networks give rise to complex information processing capabilities. Given that the automated or semi-automated methods required to achieve the acquisition of these graphs are still evolving, we developed a metric for measuring the performance of such methods by comparing their output with those generated by human annotators ("ground truth" data). Whereas classic metrics for comparing annotated neural tissue reconstructions generally do so at the voxel level, the metric proposed here measures the "integrity" of neurons based on the degree to which a collection of synaptic terminals belonging to a single neuron of the reconstruction can be matched to those of a single neuron in the ground truth data. The metric is largely insensitive to small errors in segmentation and more directly measures accuracy of the generated brain graph. It is our hope that use of the metric will facilitate the broader community's efforts to improve upon existing methods for acquiring brain graphs. Herein we describe the metric in detail, provide demonstrative examples of the intuitive scores it generates, and apply it to a synthesized neural network with simulated reconstruction errors. Demonstration code is available.
更多查看译文
关键词
connectome,computer vision,segmentation,brain graph,evaluation,Electron Microscopy,Neural Reconstruction Integrity
AI 理解论文
溯源树
样例
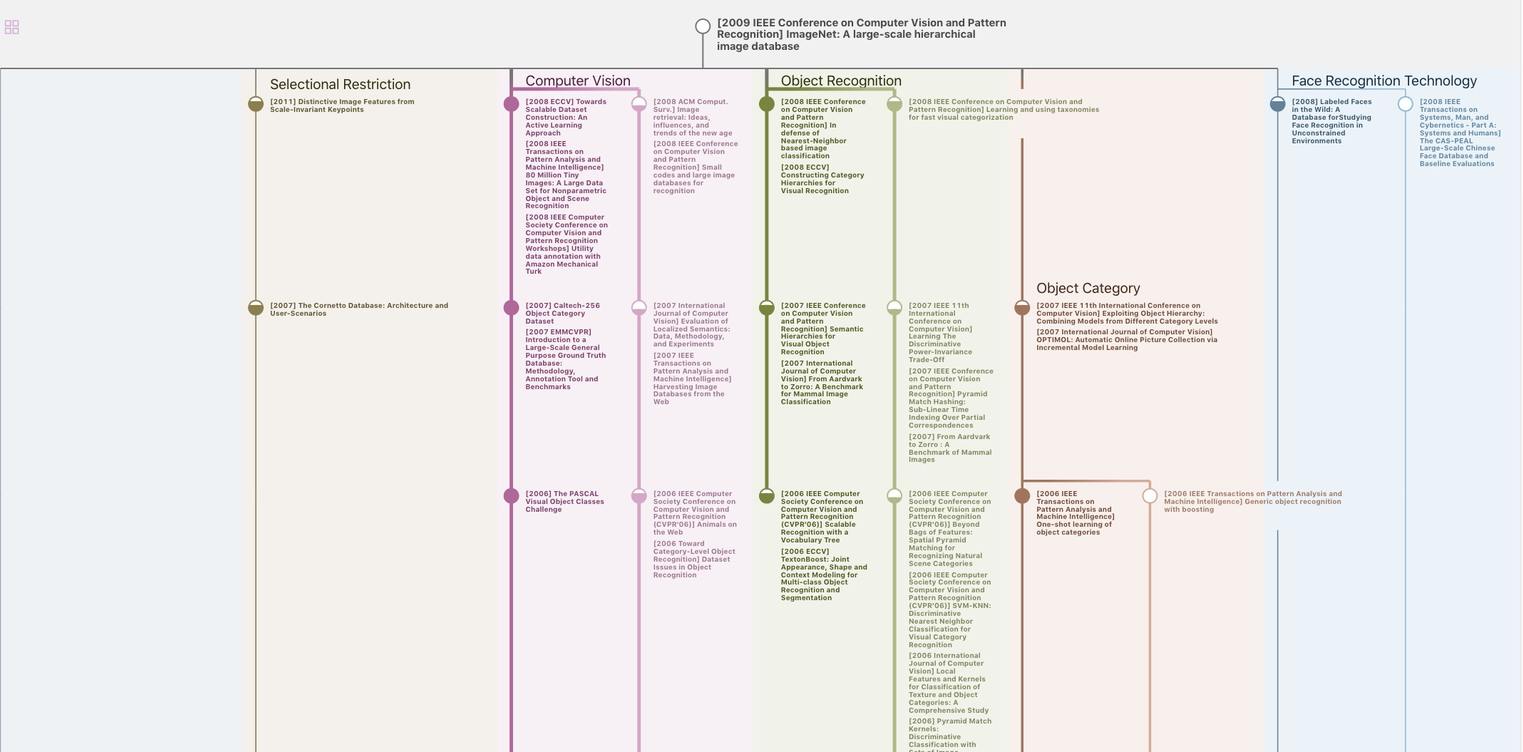
生成溯源树,研究论文发展脉络
Chat Paper
正在生成论文摘要