Virtual water quality monitoring at inactive monitoring sites using Monte Carlo optimized artificial neural networks: A case study of Danube River (Serbia).
SCIENCE OF THE TOTAL ENVIRONMENT(2019)
摘要
Rationalization of water quality monitoring stations nowadays is applied in many countries. In some cases, missing data from abandoned/inactive stations, spatial and temporal, could be very important, hence the use of artificial neural networks (ANNs) for virtual water quality monitoring at inactive monitoring sites was investigated. The aim was to develop single-output and simultaneous ANNs for the spatial interpolation of 18 water quality parameters at single- and multi-inactive monitoring sites on Danube River course through Serbia. Those different modeling approaches were considered in order to determine the most suitable combination of models. The variable selection and sensitivity analysis in the case of simultaneous models were performed using a modified procedure based on Monte Carlo Simulations (MCS). In general, the multi-target models tend to be more accurate than single target ones, while single output models outperform the simultaneous ones. Hence, for particular monitoring network and set of water quality parameters the optimal combination of models must be defined based on model's accuracy and computational effort needed. The MCS selection procedure has proved to be efficient only in the case of simultaneous multi-target model. MCS based analysis of input-output interactions has shown all significant interactions in the case of simultaneous single-target are grouped as a complex duster of interactions, where majority of inputs influence on several outputs. In the case multi-target model those interactions were portioned in five separate clusters, there majority of them mimic the input-output interactions that are present in single output models. The modeling strategy for study area was proposed on the basis of the performance of created models (mean average percentage error < 10%): simultaneous multi-target model for pH, alkalinity, conductivity, hardness, dissolved oxygen, HCO3-,SO42- and Ca, single-output multi-target models for temperature and Cl-, simultaneous single-target models for Mg and CO2, single output single target models for NO3-. (C) 2018 Elsevier B.V. All rights reserved.
更多查看译文
关键词
ANNs,Monte Carlo simulations,River water monitoring,Water quality prediction,Inactive monitoring sites
AI 理解论文
溯源树
样例
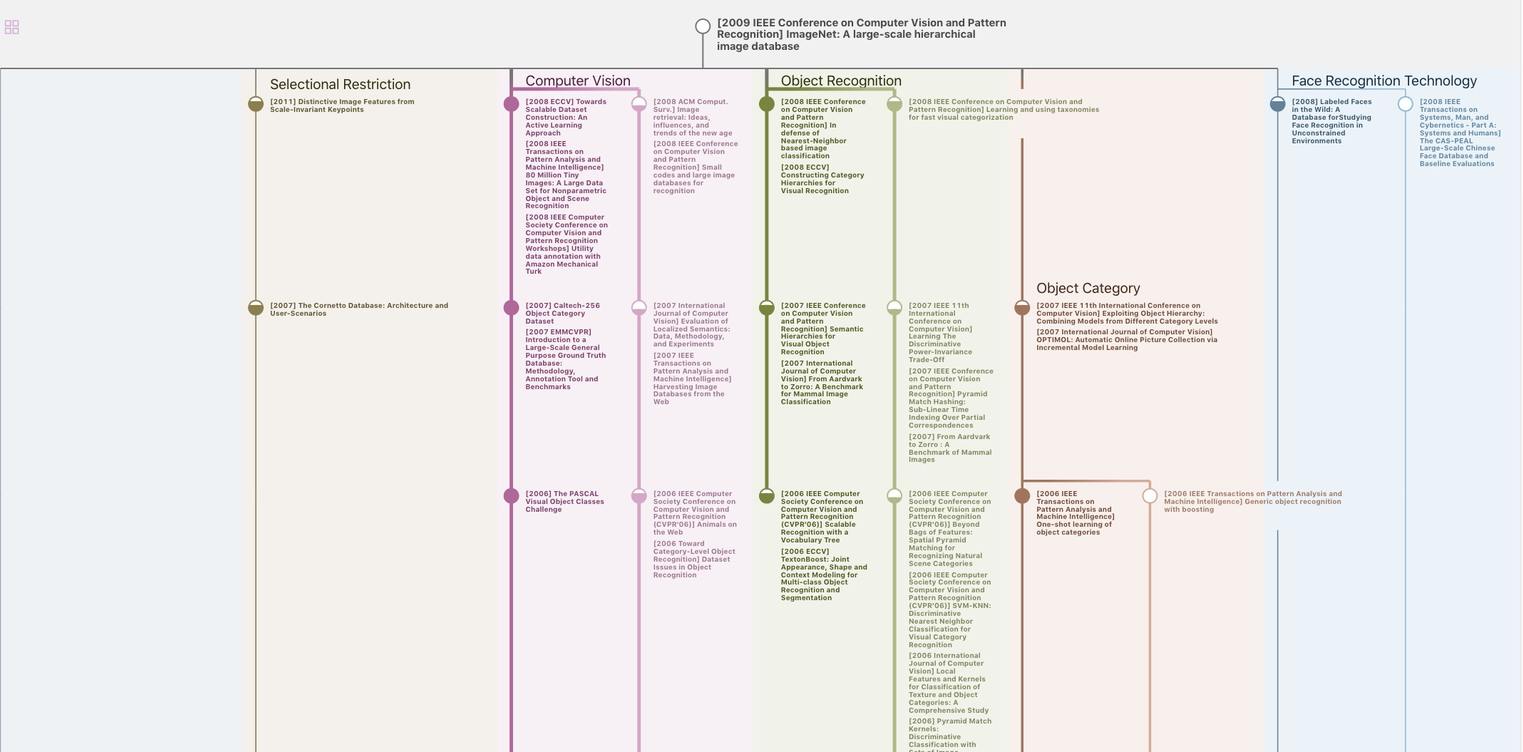
生成溯源树,研究论文发展脉络
Chat Paper
正在生成论文摘要