Enhancing the representation of functional connectivity networks by fusing multi-view information for autism spectrum disorder diagnosis.
HUMAN BRAIN MAPPING(2019)
摘要
Functional connectivity network provides novel insights on how distributed brain regions are functionally integrated, and its deviations from healthy brain have recently been employed to identify biomarkers for neuropsychiatric disorders. However, most of brain network analysis methods utilized features extracted only from one functional connectivity network for brain disease detection and cannot provide a comprehensive representation on the subtle disruptions of brain functional organization induced by neuropsychiatric disorders. Inspired by the principles of multi-view learning which utilizes information from multiple views to enhance object representation, we propose a novel multiple network based framework to enhance the representation of functional connectivity networks by fusing the common and complementary information conveyed in multiple networks. Specifically, four functional connectivity networks corresponding to the four adjacent values of regularization parameter are generated via a sparse regression model with group constraint (l(2,1)-norm), to enhance the common intrinsic topological structure and limit the error rate caused by different views. To obtain a set of more meaningful and discriminative features, we propose using a modified version of weighted clustering coefficients to quantify the subtle differences of each group-sparse network at local level. We then linearly fuse the selected features from each individual network via a multi-kernel support vector machine for autism spectrum disorder (ASD) diagnosis. The proposed framework achieves an accuracy of 79.35%, outperforming all the compared single network methods for at least 7% improvement. Moreover, compared with other multiple network methods, our method also achieves the best performance, that is, with at least 11% improvement in accuracy.
更多查看译文
关键词
computer-aided diagnosis,functional connectivity network,multi-kernel fusion,multi-view group-sparse network,multi-view learning,resting-state functional magnetic resonance imaging (R-fMRI)
AI 理解论文
溯源树
样例
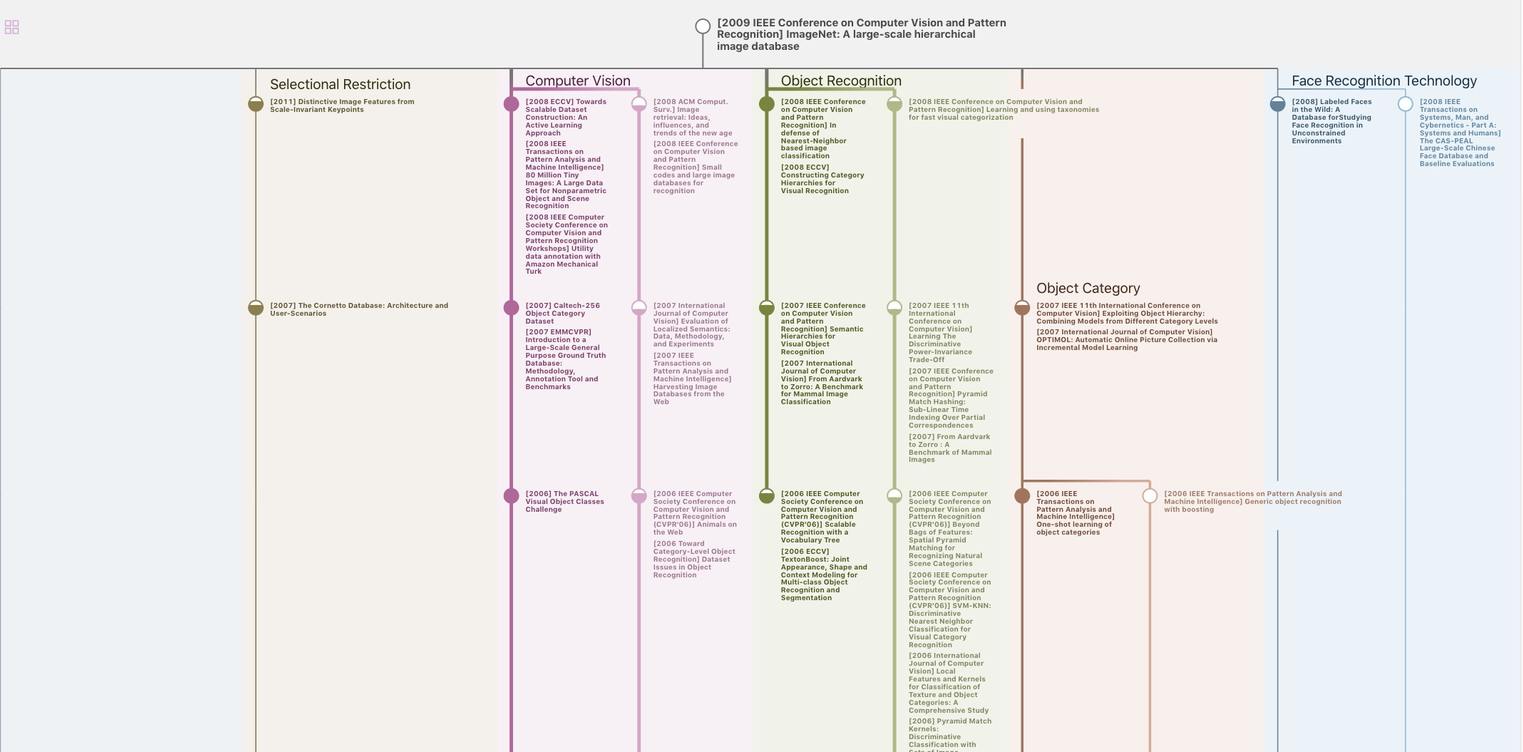
生成溯源树,研究论文发展脉络
Chat Paper
正在生成论文摘要