Mammographic Breast Density Assessment Using Deep Learning: Clinical Implementation.
RADIOLOGY(2019)
摘要
Purpose: To develop a deep learning (DL) algorithm to assess mammographic breast density. Materials and Methods: In this retrospective study, a deep convolutional neural network was trained to assess Breast Imaging Reporting and Data System (BI-RADS) breast density based on the original interpretation by an experienced radiologist of 41 479 digital screening mammograms obtained in 27 684 women from January 2009 to May 2011. The resulting algorithm was tested on a held-out test set of 8677 mammograms in 5741 women. In addition, five radiologists performed a reader study on 500 mammograms randomly selected from the test set. Finally, the algorithm was implemented in routine clinical practice, where eight radiologists reviewed 10 763 consecutive mammograms assessed with the model. Agreement on BI-RADS category for the DL model and for three sets of readings-(a) radiologists in the test set, (b) radiologists working in consensus in the reader study set, and (c) radiologists in the clinical implementation set-were estimated with linear-weighted k statistics and were compared across 5000 bootstrap samples to assess significance. Results: The DL model showed good agreement with radiologists in the test set (kappa = 0.67; 95% confidence interval [CI]: 0.66, 0.68) and with radiologists in consensus in the reader study set (kappa = 0.78; 95% CI: 0.73, 0.82). There was very good agreement (kappa = 0.85; 95% CI: 0.84, 0.86) with radiologists in the clinical implementation set; for binary categorization of dense or nondense breasts, 10 149 of 10 763 (94%; 95% CI: 94%, 95%) DL assessments were accepted by the interpreting radiologist. Conclusion: This DL model can be used to assess mammographic breast density at the level of an experienced mammographer. (c) RSNA, 2018
更多查看译文
关键词
deep learning,breast,density
AI 理解论文
溯源树
样例
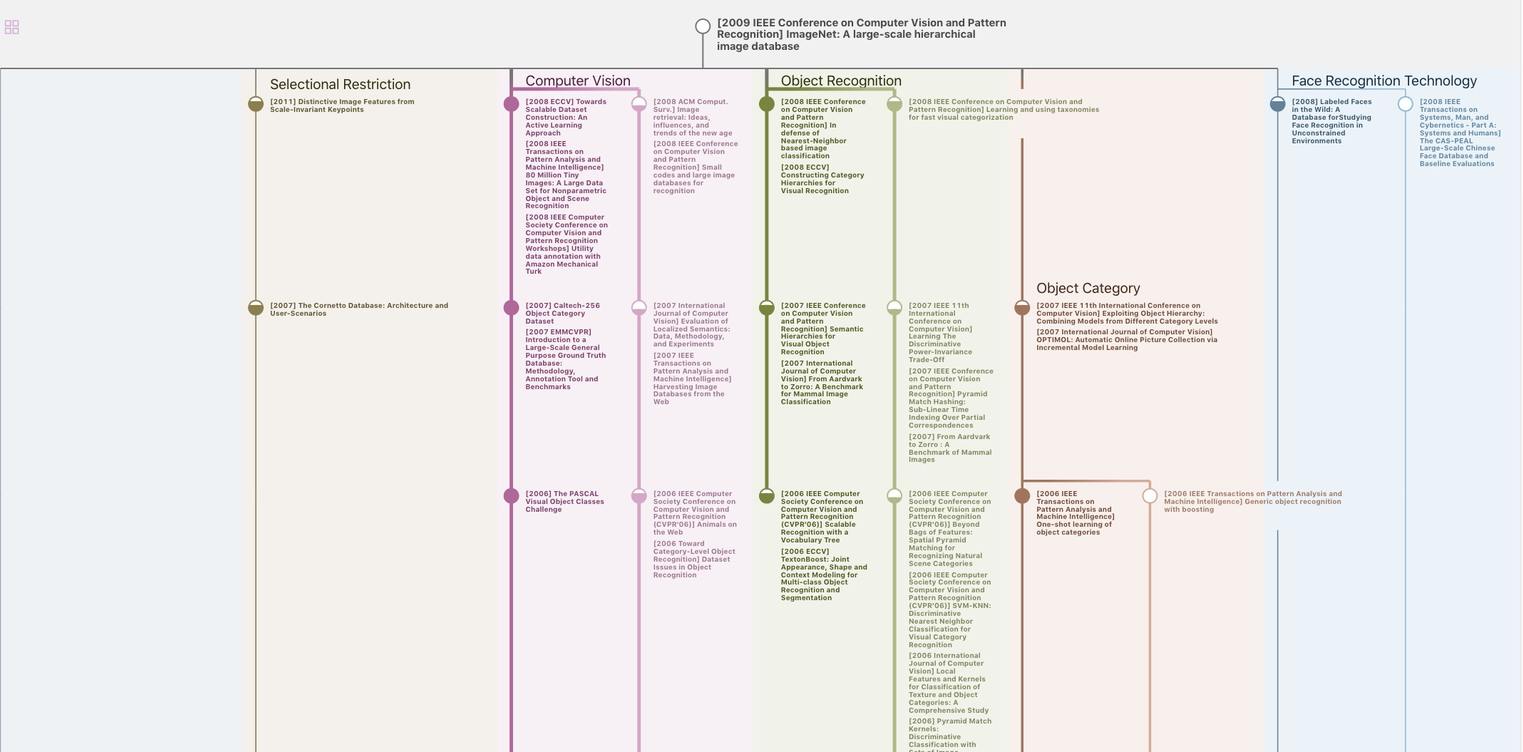
生成溯源树,研究论文发展脉络
Chat Paper
正在生成论文摘要