Removing the Mask of Average Treatment Effects in Chronic Lyme Disease Research Using Big Data and Subgroup Analysis.
HEALTHCARE(2018)
摘要
Lyme disease is caused by the bacteria borrelia burgdorferi and is spread primarily through the bite of a tick. There is considerable uncertainty in the medical community regarding the best approach to treating patients with Lyme disease who do not respond fully to short-term antibiotic therapy. These patients have persistent Lyme disease symptoms resulting from lack of treatment, under-treatment, or lack of response to their antibiotic treatment protocol. In the past, treatment trials have used small restrictive samples and relied on average treatment effects as their measure of success and produced conflicting results. To provide individualized care, clinicians need information that reflects their patient population. Today, we have the ability to analyze large data bases, including patient registries, that reflect the broader range of patients more typically seen in clinical practice. This allows us to examine treatment variation within the sample and identify groups of patients that are most responsive to treatment. Using patient-reported outcome data from the MyLymeData online patient registry, we show that sub-group analysis techniques can unmask valuable information that is hidden if averages alone are used. In our analysis, this approach revealed treatment effectiveness for up to a third of patients with Lyme disease. This study is important because it can help open the door to more individualized patient care using patient-centered outcomes and real-world evidence.
更多查看译文
关键词
Lyme disease,real-world evidence,patient-centered research,patient-reported outcomes,treatment heterogeneity,average treatment effect,global rating of change scale,individualized care,patient registries,big data
AI 理解论文
溯源树
样例
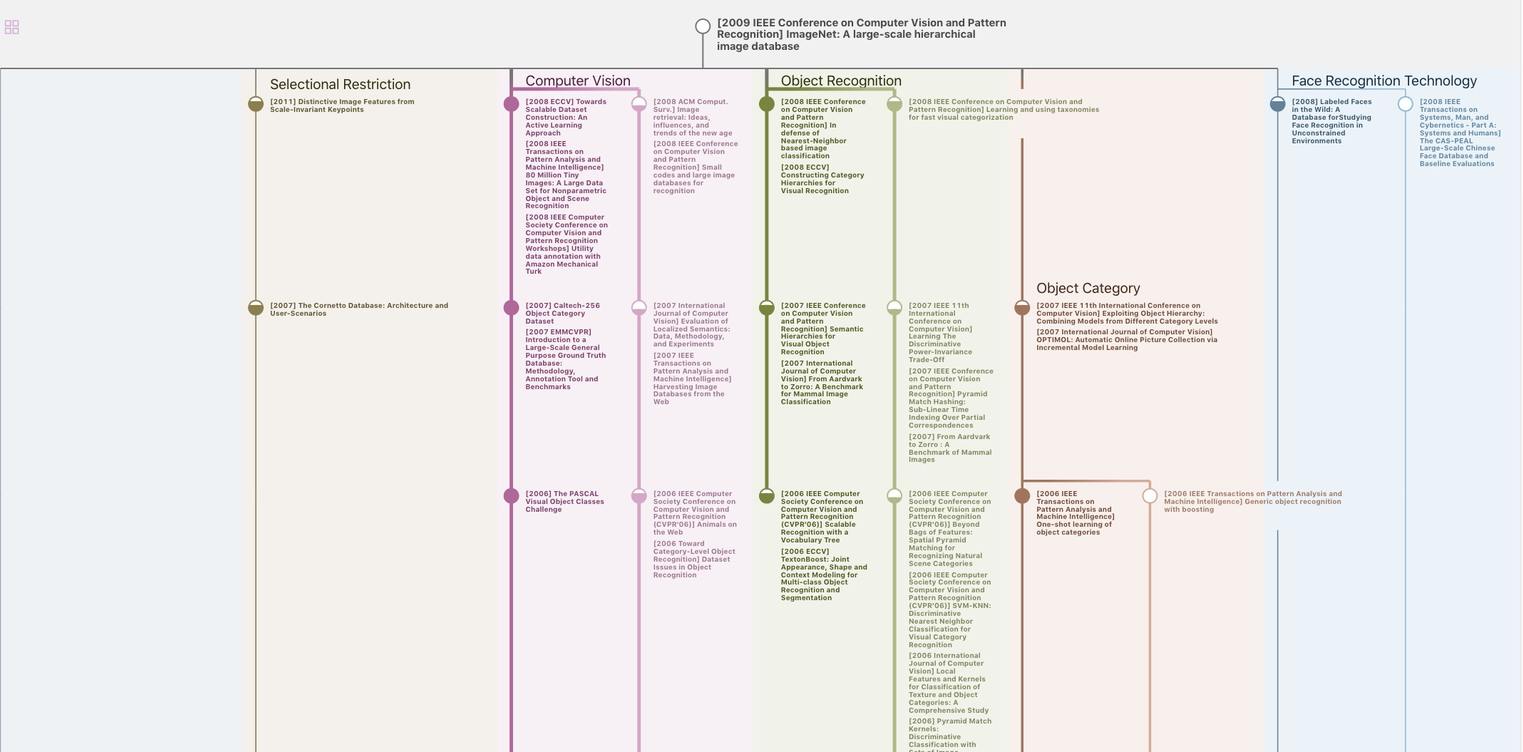
生成溯源树,研究论文发展脉络
Chat Paper
正在生成论文摘要