SEFRON: A New Spiking Neuron Model With Time-Varying Synaptic Efficacy Function for Pattern Classification.
IEEE transactions on neural networks and learning systems(2019)
摘要
This paper presents a new time-varying long-term Synaptic Efficacy Function-based leaky-integrate-and-fire neuRON model, referred to as SEFRON and its supervised learning rule for pattern classification problems. The time-varying synaptic efficacy function is represented by a sum of amplitude modulated Gaussian distribution functions located at different times. For a given pattern, the SEFRON's learning rule determines the changes in the amplitudes of weights at selected presynaptic spike times by minimizing a new error function reflecting the differences between the desired and actual postsynaptic firing times. Similar to the gamma-aminobutyric acid-switch phenomenon observed in a biological neuron that switches between excitatory and inhibitory postsynaptic potentials based on the physiological needs, the time-varying synapse model proposed in this paper allows the synaptic efficacy (weight) to switch signs in a continuous manner. The computational power and the functioning of SEFRON are first illustrated using a binary pattern classification problem. The detailed performance comparisons of a single SEFRON classifier with other spiking neural networks (SNNs) are also presented using four benchmark data sets from the UCI machine learning repository. The results clearly indicate that a single SEFRON provides a similar generalization performance compared to other SNNs with multiple layers and multiple neurons.
更多查看译文
关键词
Neurons,Synapses,Biological system modeling,Computational modeling,Data models,Heuristic algorithms,Microsoft Windows
AI 理解论文
溯源树
样例
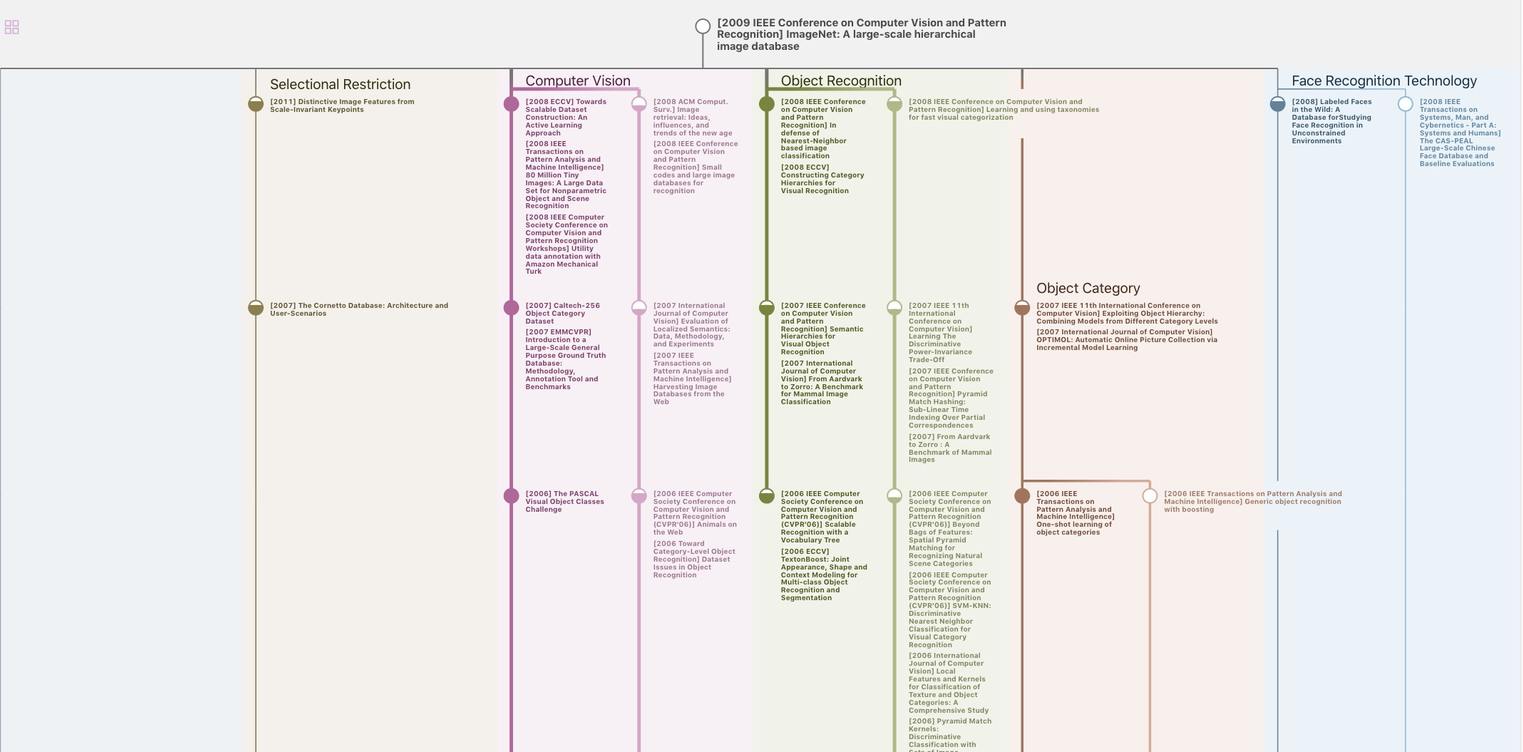
生成溯源树,研究论文发展脉络
Chat Paper
正在生成论文摘要