Machine learning for intraoperative prediction of viability in ischemic small intestine.
PHYSIOLOGICAL MEASUREMENT(2018)
摘要
Objective: Evaluation of intestinal viability is essential in surgical decision-making in patients with acute intestinal ischemia. There has been no substantial change in the mortality rate (30%-93%) of patients with acute mesenteric ischemia (AMI) since the 1980s. As the accuracy from the first laparotomy alone is 50%, the gold standard is a second-look laparotomy, increasing the accuracy to 87%-89%. This study investigates the use of machine learning to classify intestinal viability and histological grading in pig jejunum, based on multivariate time-series of bioimpedance sensor data. Approach: We have previously used a bioimpedance sensor system to acquire electrical parameters from perfused, ischemic and reperfused pig jejunum (7 + 15 pigs) over 1-16 h of ischemia and 1-8 h of reperfusion following selected durations of ischemia. In this study we compare the accuracy of using end-point bioimpedance measurements with a feedforward neural network (FNN), versus the accuracy when using a recurrent neural network with long short-term memory units (LSTM-RNN) with bioimpedance data history over different periods of time. Main results: Accuracies in the range of what has been reported clinically can be achieved using FNN's on a single bioimpedance measurement, and higher accuracies can be achieved when employing LSTM-RNN on a sequence of data history. Significance: Intraoperative bioimpedance measurements on intestine of suspect viability combined with machine learning can increase the accuracy of intraoperative assessment of intestinal viability. Increased accuracy in intraoperative assessment of intestinal viability has the potential to reduce the high mortality and morbidity rate of the patients.
更多查看译文
关键词
intestinal viability,bioimpedance,recurring neural networks,LSTM,ischemia
AI 理解论文
溯源树
样例
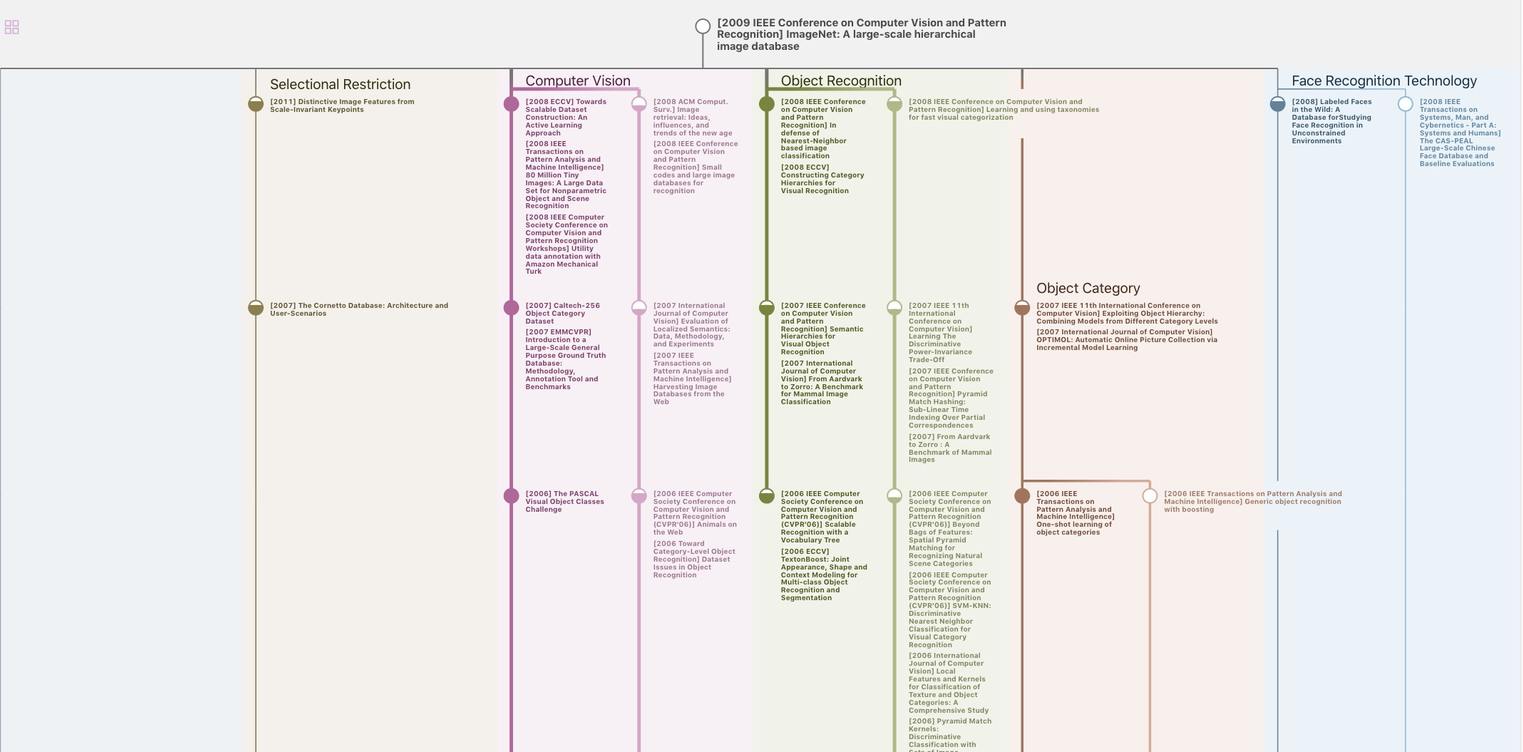
生成溯源树,研究论文发展脉络
Chat Paper
正在生成论文摘要