Relative Accuracy of Two Modified Parallel Analysis Methods that Use the Proper Reference Distribution.
EDUCATIONAL AND PSYCHOLOGICAL MEASUREMENT(2018)
摘要
Parallel analysis (PA) assesses the number of factors in exploratory factor analysis. Traditionally PA compares the eigenvalues for a sample correlation matrix with the eigenvalues for correlation matrices for 100 comparison datasets generated such that the variables are independent, but this approach uses the wrong reference distribution. The proper reference distribution of eigenvalues assesses the kth factor based on comparison datasets with k-1 underlying factors. Two methods that use the proper reference distribution are revised PA (R-PA) and the comparison data method (CDM). We compare the accuracies of these methods using Monte Carlo methods by manipulating the factor structure, factor loadings, factor correlations, and number of observations. In the 17 conditions in which CDM was more accurate than R-PA, both methods evidenced high accuracies (i.e.,>94.5%). In these conditions, CDM had slightly higher accuracies (mean difference of 1.6%). In contrast, in the remaining 25 conditions, R-PA evidenced higher accuracies (mean difference of 12.1%, and considerably higher for some conditions). We consider these findings in conjunction with previous research investigating PA methods and concluded that R-PA tends to offer somewhat stronger results. Nevertheless, further research is required. Given that both CDM and R-PA involve hypothesis testing, we argue that future research should explore effect size statistics to augment these methods.
更多查看译文
关键词
exploratory factor analysis,parallel analysis,psychometrics
AI 理解论文
溯源树
样例
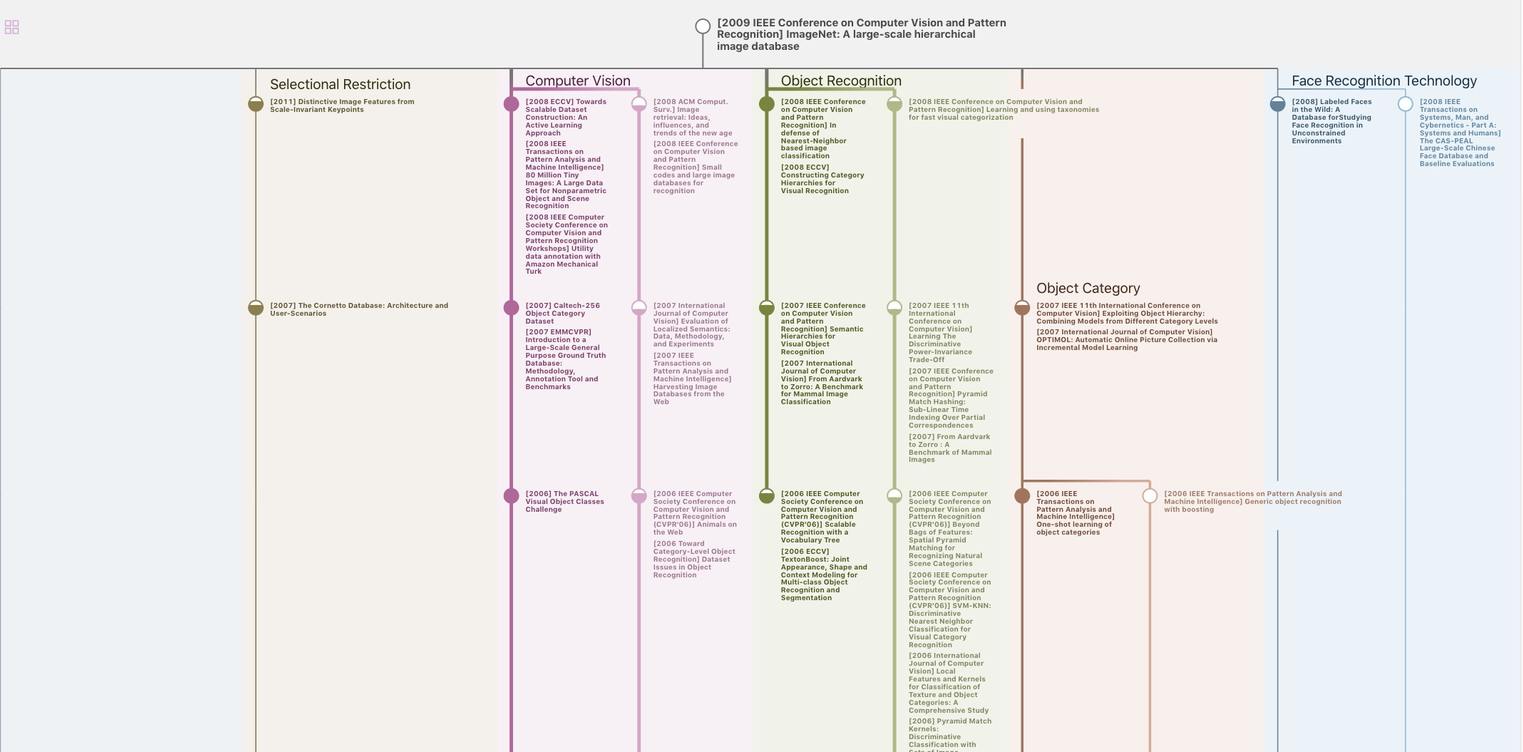
生成溯源树,研究论文发展脉络
Chat Paper
正在生成论文摘要