Automated robust proton planning using dose-volume histogram-based mimicking of the photon reference dose and reducing organ at risk dose optimization.
International Journal of Radiation Oncology*Biology*Physics(2019)
摘要
Patient selection for proton therapy is increasingly based on proton to photon plan comparisons. To improve efficient decision making, we developed a dose mimicking and reducing (DMR) algorithm to automatically generate a robust proton plan from a reference photon dose and target and organs at risk (OARs) delineations. The DMR algorithm was evaluated in 40 head and neck cancer patients. The first step of the DMR algorithm comprises DVH-based mimicking of the photon dose distribution in the clinical target volumes and OARs. Target robustness is included by mimicking the nominal photon dose in 21 perturbed scenarios. The second step of the optimization aims to reduce the OAR doses while retaining the robust target coverage as achieved in the first step. We evaluated each DMR plan against the 'manually' robustly optimized reference proton plan in terms of plan robustness (voxel-wise minimum dose). Furthermore, the DMR plans were evaluated against the reference photon plan using normal tissue complication probability (NTCP) models of xerostomia, dysphagia and tube feeding dependence. Consequently, ΔNTCPs were defined as the difference between the NTCPs of the photon and proton plans. The dose distributions of the DMR and reference proton plans were very similar in terms of target robustness and OAR dose values. Regardless of proton planning technique (i.e. DMR or reference proton plan), the same treatment modality was selected in 80% (32/40) of cases based on the ∑ΔNTCPs. In 15% (6/40) of cases a conflicting decision was made based on relatively small dose differences to the OARs (<2.0 Gy). The DMR algorithm automatically optimized robust proton plans from a photon reference dose comparable to the dosimetrist-optimized proton plans in head and neck cancer patients. This algorithm has been successfully embedded into a framework to automatically select patients for proton therapy based on normal tissue complication probabilities.
更多查看译文
AI 理解论文
溯源树
样例
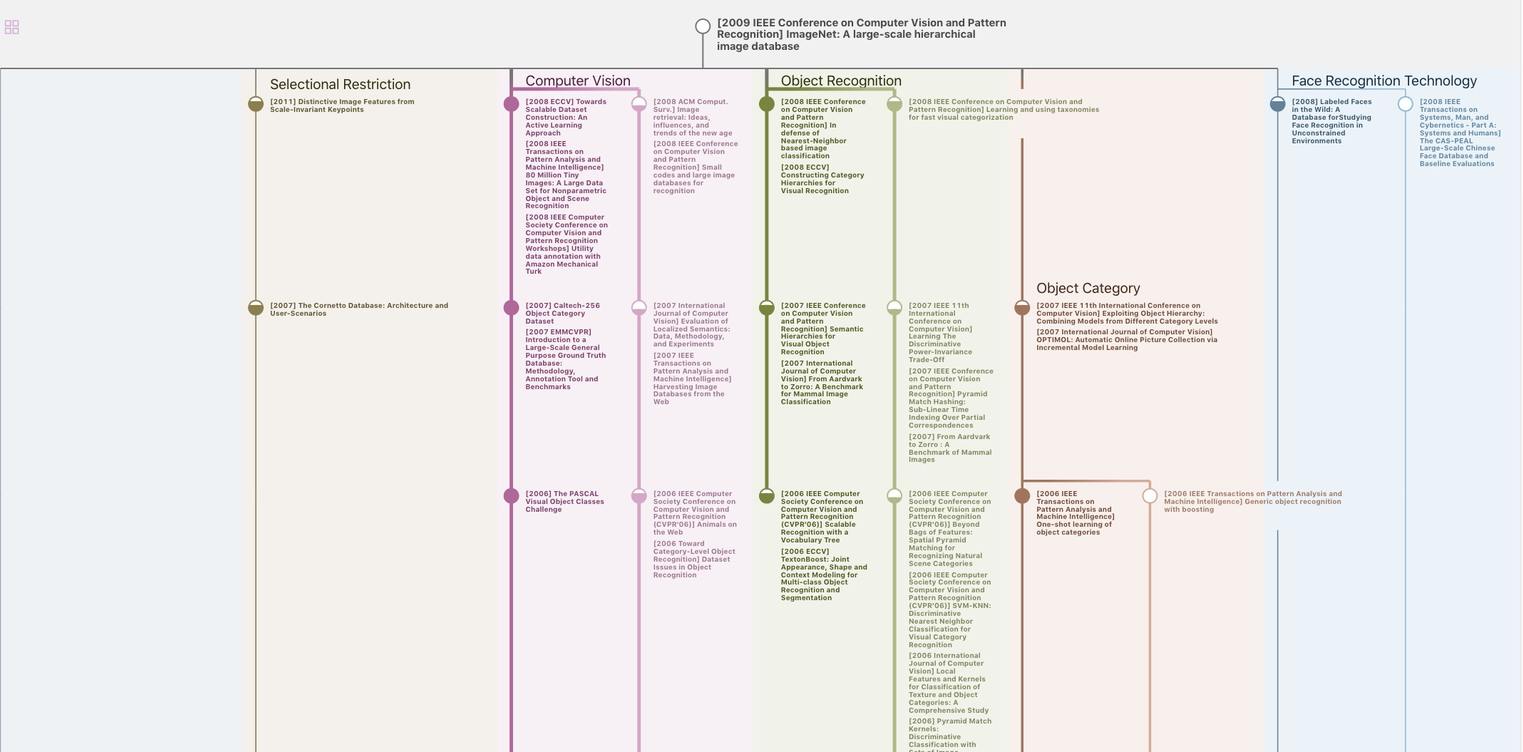
生成溯源树,研究论文发展脉络
Chat Paper
正在生成论文摘要