Efficient Robust Estimation for Linear Models with Missing Response at Random.
SCANDINAVIAN JOURNAL OF STATISTICS(2018)
摘要
Coefficient estimation in linear regression models with missing data is routinely carried out in the mean regression framework. However, the mean regression theory breaks down if the error variance is infinite. In addition, correct specification of the likelihood function for existing imputation approach is often challenging in practice, especially for skewed data. In this paper, we develop a novel composite quantile regression and a weighted quantile average estimation procedure for parameter estimation in linear regression models when some responses are missing at random. Instead of imputing the missing response by randomly drawing from its conditional distribution, we propose to impute both missing and observed responses by their estimated conditional quantiles given the observed data and to use the parametrically estimated propensity scores to weigh check functions that define a regression parameter. Both estimation procedures are resistant to heavy-tailed errors or outliers in the response and can achieve nice robustness and efficiency. Moreover, we propose adaptive penalization methods to simultaneously select significant variables and estimate unknown parameters. Asymptotic properties of the proposed estimators are carefully investigated. An efficient algorithm is developed for fast implementation of the proposed methodologies. We also discuss a model selection criterion, which is based on an ICQ-type statistic, to select the penalty parameters. The performance of the proposed methods is illustrated via simulated and real data sets.
更多查看译文
关键词
conditional quantile,imputation,missing at random,model selection,quantile regression
AI 理解论文
溯源树
样例
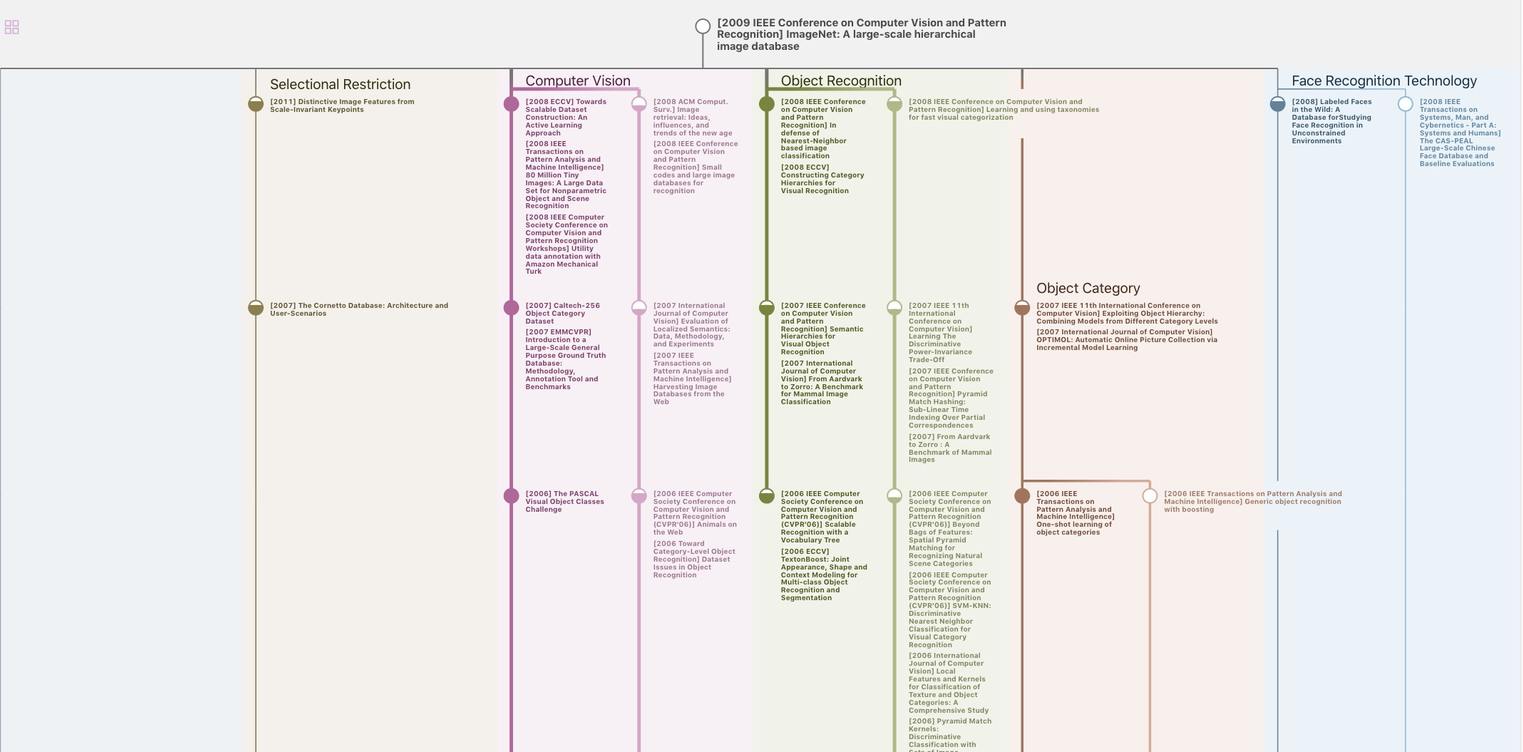
生成溯源树,研究论文发展脉络
Chat Paper
正在生成论文摘要