Marked Loss of Muscle, Visceral Fat, or Subcutaneous Fat After Gastrectomy Predicts Poor Survival in Advanced Gastric Cancer: Single-Center Study from the CLASSIC Trial
Annals of surgical oncology(2018)
摘要
Background There is increasing interest in the influence of body composition on oncological outcomes. We evaluated the role of skeletal muscle and fat among patients with gastric cancer (GC) who underwent gastrectomy with or without adjuvant chemotherapy, as well as those changes’ associations with survival outcomes. Methods The present study evaluated 136 patients with GC who were enrolled in the CLASSIC Trial at Yonsei Cancer Center. Baseline body compositions including skeletal muscle area, Hounsfield units (HU), visceral fat area, and subcutaneous fat area were measured by preoperative computed tomography (CT). CT before and after the gastrectomy were used to determine the 6-month relative changes in body composition parameters. Continuous variables were dichotomized according to the best cutoff values by Contal and O’Quigley method. Results Seventy-three patients (53.7%) underwent surgery alone, and 63 patients (46.3%) underwent surgery followed by adjuvant chemotherapy. The baseline body composition parameters were not associated with disease-free survival (DFS) or overall survival (OS). Except for the HU, the marked loss of muscle, visceral fat, or subcutaneous fat significantly predicted shorter DFS and OS. Patients with a marked loss in at least one significant body composition parameter had significantly shorter DFS (hazard ratio 2.9, 95% confidence interval 1.7–4.8, P < 0.001) and OS (hazard ratio 2.9, 95% confidence interval 1.7–5.0, P < 0.001). Conclusions Marked loss in body composition parameters significantly predicted shorter DFS and OS among patients with GC who underwent gastrectomy. Postoperative nutrition and active healthcare interventions could improve the prognosis of these GC patients.
更多查看译文
关键词
CLASSIC Trial,Marked Loss,Gastric Cancer,Predicted Poorer Survival,Body Composition Parameters
AI 理解论文
溯源树
样例
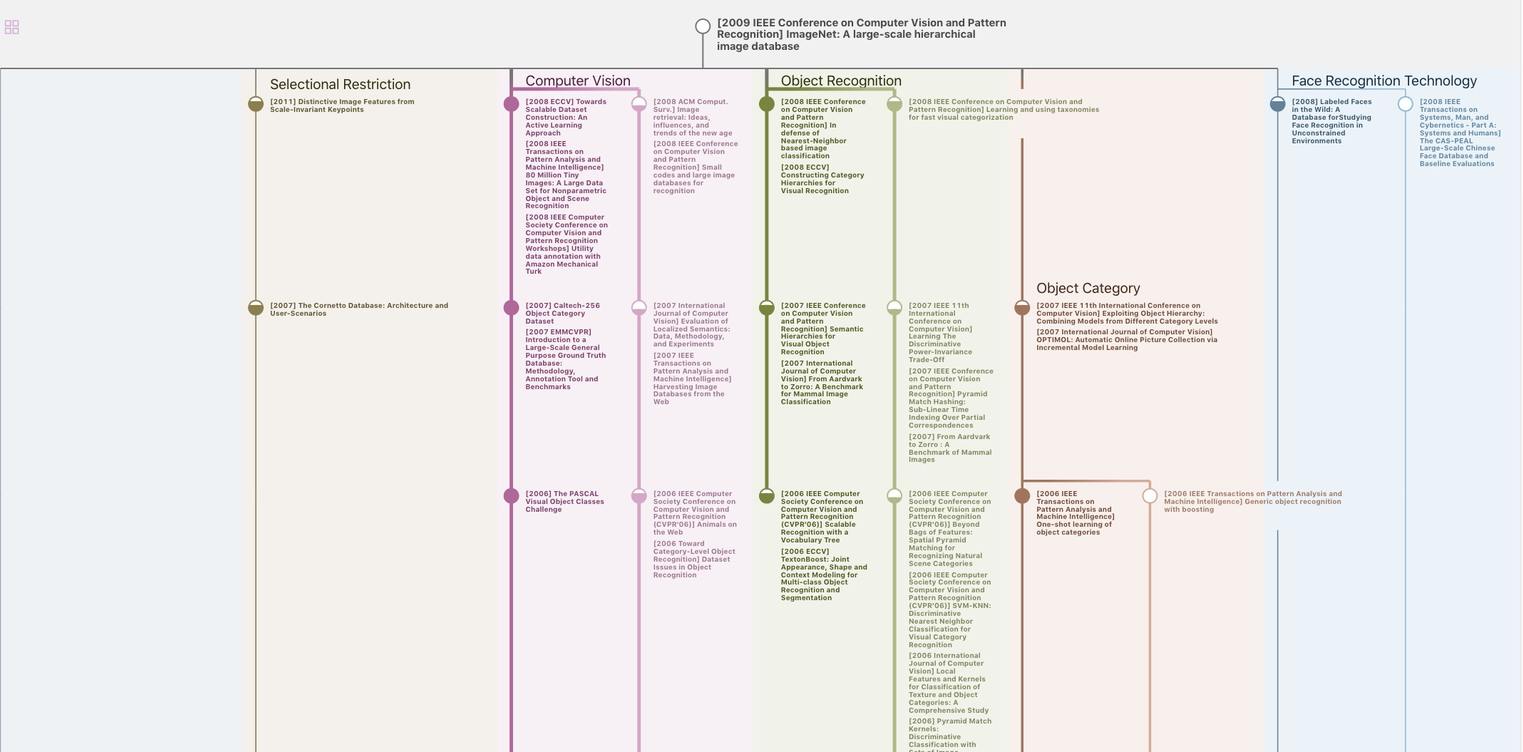
生成溯源树,研究论文发展脉络
Chat Paper
正在生成论文摘要