Numero: A Statistical Framework To Define Multivariable Subgroups In Complex Population-Based Datasets
INTERNATIONAL JOURNAL OF EPIDEMIOLOGY(2019)
摘要
Large-scale epidemiological and population data provide opportunities to identify subgroups of people who are at risk of disease or exposed to adverse environments. Clustering algorithms are popular data-driven tools to identify these subgroups; however, relying exclusively on algorithms may not produce the best results if the dataset does not have a clustered structure. For this reason, we propose a framework (the R-library Numero) that combines the self-organizing map algorithm, permutation analysis for statistical evidence and a final expert-driven subgrouping step. We used Numero to define subgroups in two examples without an obvious clustering structure: a biomedical dataset of kidney disease and another dataset of community-level socioeconomic indicators. We benchmarked the Numero subgroupings against popular clustering algorithms (principal components, K-means and hierarchical clustering). The Numero subgroupings were more intuitive and easier to interpret without losing mathematical quality. Therefore, we expect Numero to be useful for exploratory analyses of population-based epidemiological datasets.
更多查看译文
关键词
Multivariable statistics, data-driven subgrouping, self-organizing map, population data
AI 理解论文
溯源树
样例
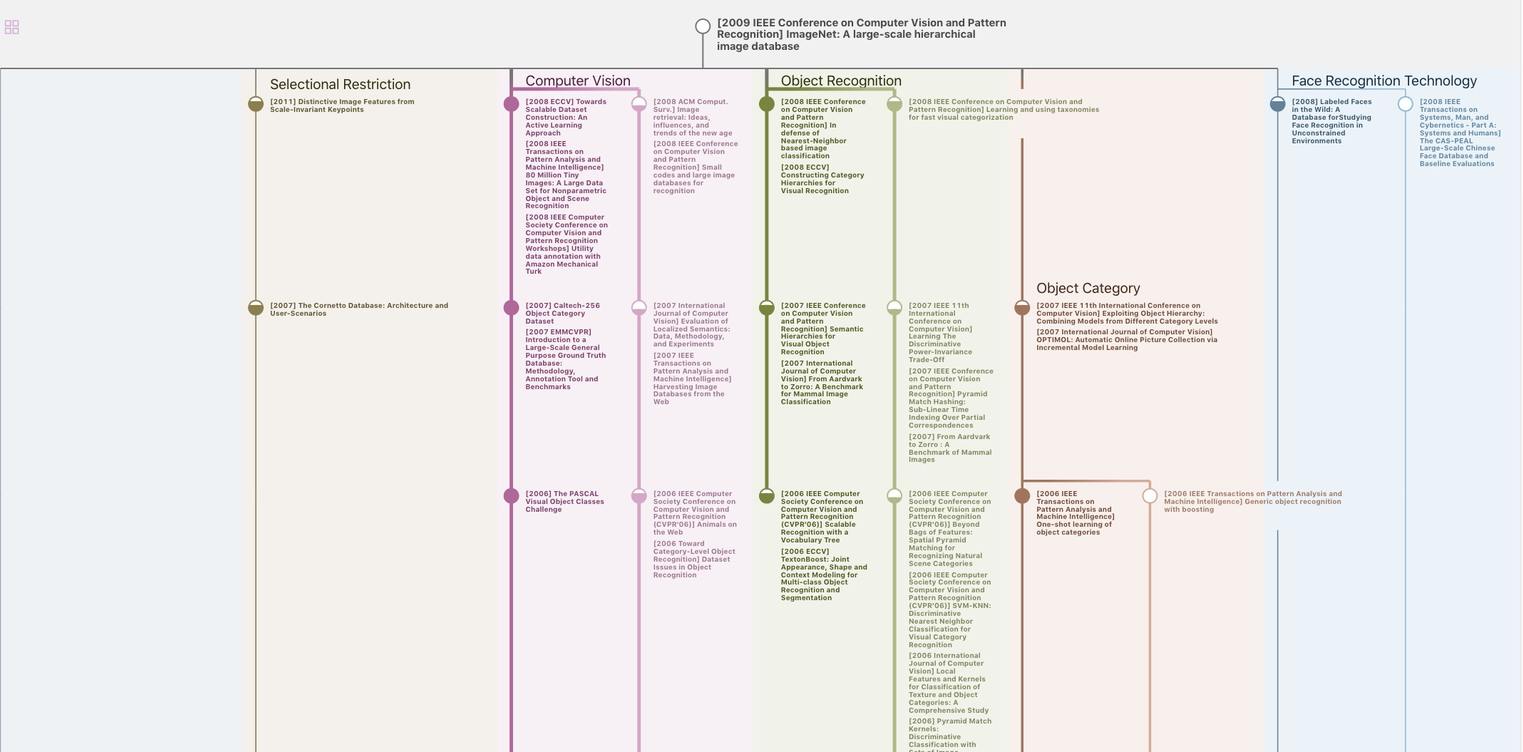
生成溯源树,研究论文发展脉络
Chat Paper
正在生成论文摘要