Combining SENSE and reduced field-of-view for high-resolution diffusion weighted magnetic resonance imaging
Biomedical engineering online(2018)
摘要
Background In diffusion-weighted magnetic resonance imaging (DWI) using single-shot echo planar imaging (ss-EPI), both reduced field-of-view (FOV) excitation and sensitivity encoding (SENSE) alone can increase in-plane resolution to some degree. However, when the two techniques are combined to further increase resolution without pronounced geometric distortion, the resulted images are often corrupted by high level of noise and artifact due to the numerical restriction in SENSE. Hence, this study is aimed to provide a reconstruction method to deal with this problem. Methods The proposed reconstruction method was developed and implemented to deal with the high level of noise and artifact in the combination of reduced FOV imaging and traditional SENSE, in which all the imaging data were considered jointly by incorporating the motion induced phase variations among excitations. The in vivo human spine diffusion images from ten subjects were acquired at 1.5 T and reconstructed using the proposed method, and compared with SENSE magnitude average results for a range of reduction factors in reduced FOV. These images were evaluated by two radiologists using visual scores (considering distortion, noise and artifact levels) from 1 to 10. Results The proposed method was able to reconstruct images with greatly reduced noise and artifact compared to SENSE magnitude average. The mean g-factors were maintained close to 1 along with enhanced signal-to-noise ratio efficiency. The image quality scores of the proposed method were significantly higher (P < 0.01) than SENSE magnitude average for all the evaluated reduction factors. Conclusion The proposed method can improve the combination of SENSE and reduced FOV for high-resolution ss-EPI DWI with reduced noise and artifact.
更多查看译文
关键词
Diffusion-weighted imaging,Parallel imaging,Reduced field-of-view,SENSE,Single-shot echo-planar imaging,g-Factor
AI 理解论文
溯源树
样例
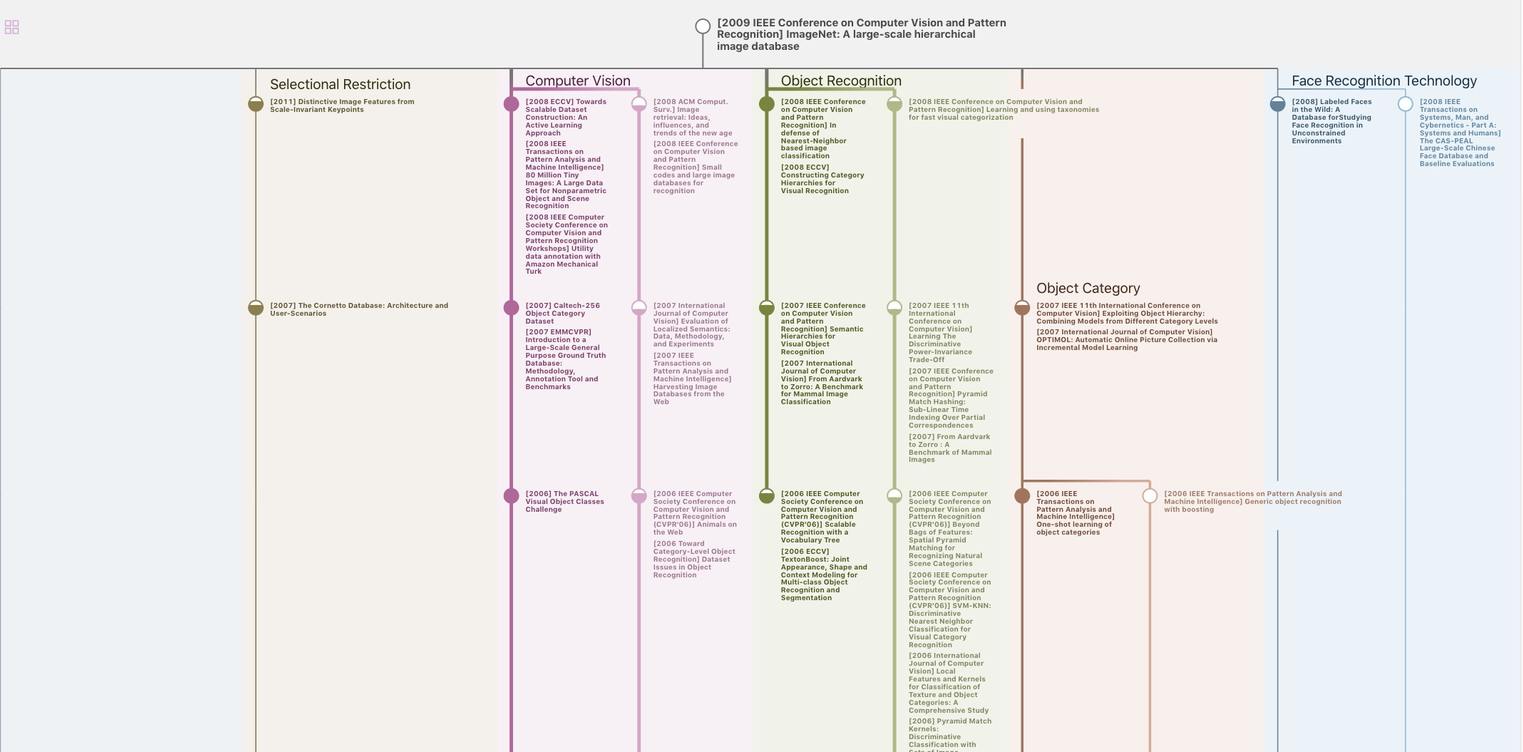
生成溯源树,研究论文发展脉络
Chat Paper
正在生成论文摘要