Learning-Based Cell Injection Control for Precise Drop-on-Demand Cell Printing
Annals of biomedical engineering(2018)
摘要
Drop-on-demand (DOD) printing is widely used in bioprinting for tissue engineering because of little damage to cell viability and cost-effectiveness. However, satellite droplets may be generated during printing, deviating cells from the desired position and affecting printing position accuracy. Current control on cell injection in DOD printing is primarily based on trial-and-error process, which is time-consuming and inflexible. In this paper, a novel machine learning technology based on Learning-based Cell Injection Control (LCIC) approach is demonstrated for effective DOD printing control while eliminating satellite droplets automatically. The LCIC approach includes a specific computational fluid dynamics (CFD) simulation model of piezoelectric DOD print-head considering inverse piezoelectric effect, which is used instead of repetitive experiments to collect data, and a multilayer perceptron (MLP) network trained by simulation data based on artificial neural network algorithm, using the well-known classification performance of MLP to optimize DOD printing parameters automatically. The test accuracy of the LCIC method was 90%. With the validation of LCIC method by experiments, satellite droplets from piezoelectric DOD printing are reduced significantly, improving the printing efficiency drastically to satisfy requirements of manufacturing precision for printing complex artificial tissues. The LCIC method can be further used to optimize the structure of DOD print-head and cell behaviors.
更多查看译文
关键词
Cell printing,Machine learning,Artificial neural network,Multilayer perceptron,Computational fluid dynamics
AI 理解论文
溯源树
样例
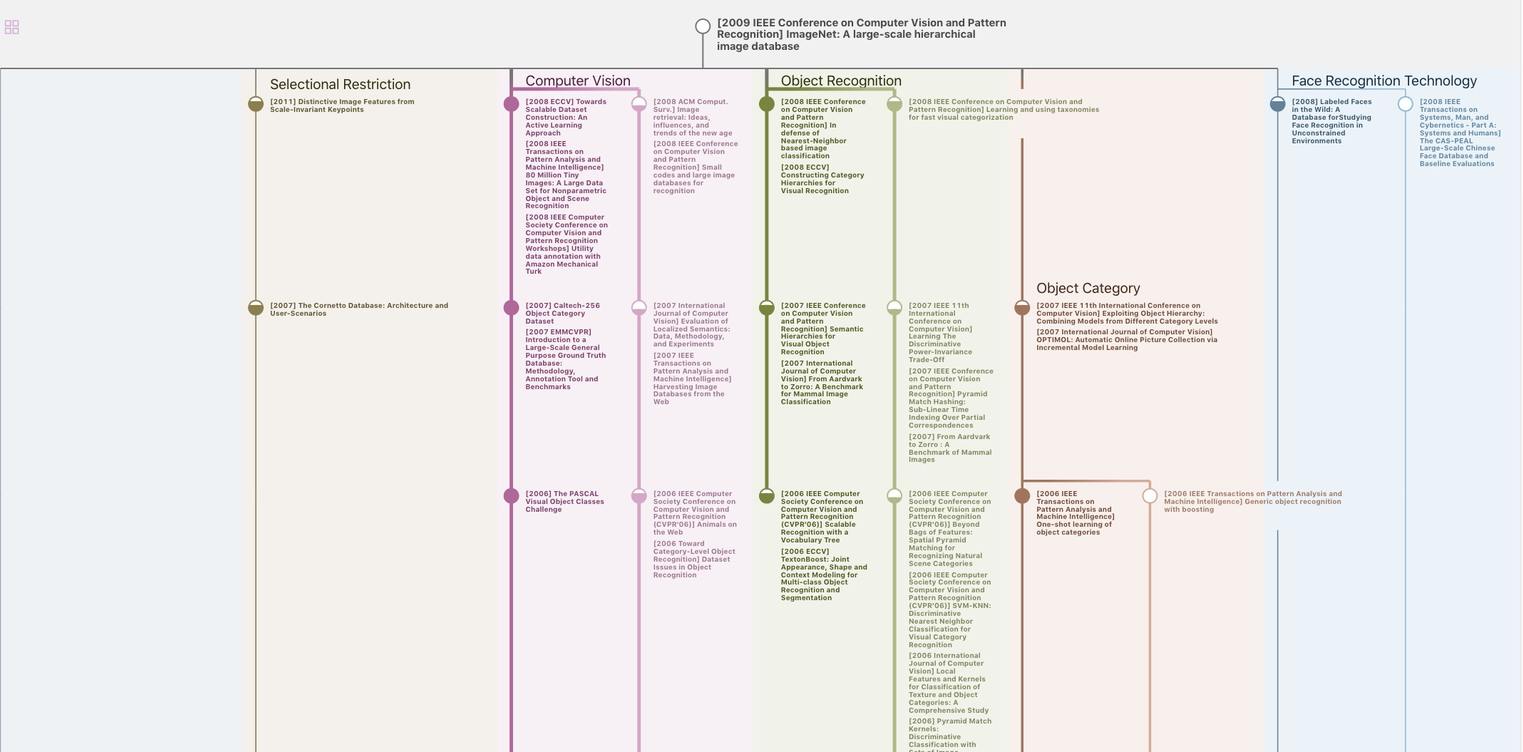
生成溯源树,研究论文发展脉络
Chat Paper
正在生成论文摘要