Machine learning-based dual-energy CT parametric mapping.
PHYSICS IN MEDICINE AND BIOLOGY(2018)
摘要
The aim is to develop and evaluate machine learning methods for generating quantitative parametric maps of effective atomic number (Z(eff)), relative electron density (rho(e)), mean excitation energy (I-x), and relative stopping power (RSP) from clinical dual-energy CT data. The maps could be used for material identification and radiation dose calculation. Machine learning methods of historical centroid (HC), random forest (RF), and artificial neural networks (ANN) were used to learn the relationship between dual-energy CT input data and ideal output parametric maps calculated for phantoms from the known compositions of 13 tissue substitutes. After training and model selection steps, the machine learning predictors were used to generate parametric maps from independent phantom and patient input data. Precision and accuracy were evaluated using the ideal maps. This process was repeated for a range of exposure doses, and performance was compared to that of the clinically-used dual-energy, physics-based method which served as the reference. The machine learning methods generated more accurate and precise parametric maps than those obtained using the reference method. Their performance advantage was particularly evident when using data from the lowest exposure, one-fifth of a typical dinical abdomen CT acquisition. The RF method achieved the greatest accuracy. In comparison, the ANN method was only 1% less accurate but had much better computational efficiency than RF, being able to produce parametric maps in 15 s. Machine learning methods outperformed the reference method in terms of accuracy and noise tolerance when generating parametric maps, encouraging further exploration of the techniques. Among the methods we evaluated, ANN is the most suitable for clinical use due to its combination of accuracy, excellent low-noise performance, and computational efficiency.
更多查看译文
关键词
CT,dual-energy CT,spectral-CT,machine learning
AI 理解论文
溯源树
样例
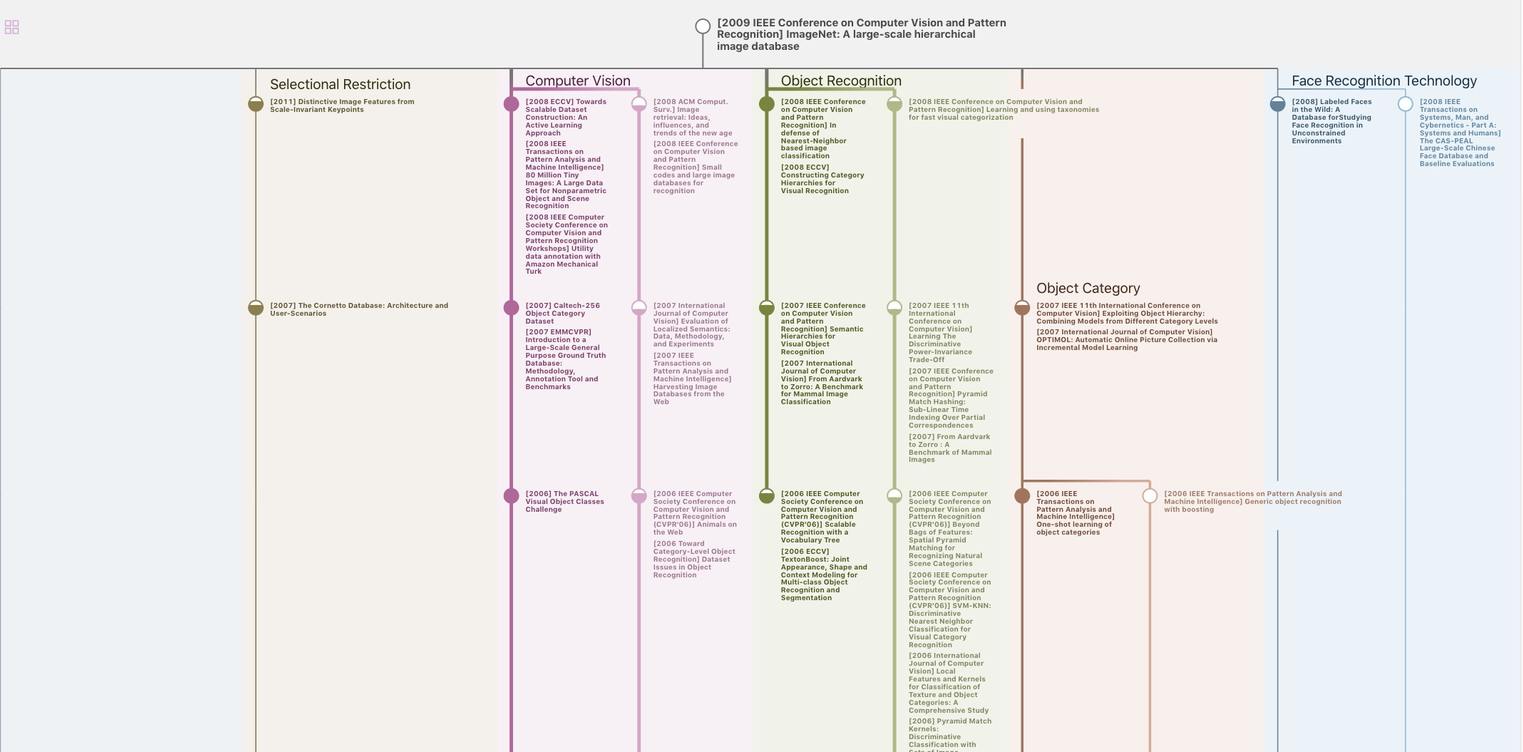
生成溯源树,研究论文发展脉络
Chat Paper
正在生成论文摘要