VSITA, an Improved Approach of Target Amplification in the Identification of Viral Pathogens.
Biomedical and environmental sciences : BES(2018)
摘要
OBJECTIVE:Unbiased next generation sequencing (NGS) is susceptible to interference from host or environmental sequences. Consequently, background depletion and virome enrichment techniques are usually needed for clinical samples where viral load is much lower than background sequences.
METHODS:A viral Sequence Independent Targeted Amplification (VSITA) approach using a set of non-ribosomal and virus-enriched octamers (V8) was developed and compared with traditionally used random hexamers (N6). Forty-five archived clinical samples of different types were used in parallel to compare the V8 and N6 enrichment performance of viral sequences and removal performance of ribosomal sequences in the step of reverse transcription followed by quantitative PCR (qPCR). Ten sera samples from patients with fever of unknown origin and 10 feces samples from patients with diarrhea of unknown origin were used in comparison of V8 and N6 enrichment performance following NGS analysis.
RESULTS:A minimum 30 hexamers matching to viral reference sequences (sense and antisense) were selected from a dataset of random 4,096 (46) hexamers (N6). Two random nucleotides were added to the 5' end of the selected hexamers, and 480 (30 × 42) octamers (V8) were obtained. In general, VSITA approach showed higher enrichment of virus-targeted cDNA and enhanced ability to remove unwanted ribosomal sequences in the majorities of 45 predefined clinical samples. Moreover, VSITA combined with NGS enabled to detect not only more viruses but also achieve more viral reads hit and higher viral genome coverage in 20 clinical samples with diarrhea or fever of unknown origin.
CONCLUSION:The VSITA approach designed in this study is demonstrated to possess higher sensitivity and broader genome coverage than traditionally used random hexamers in the NGS-based identification of viral pathogens directly from clinical samples.
更多查看译文
AI 理解论文
溯源树
样例
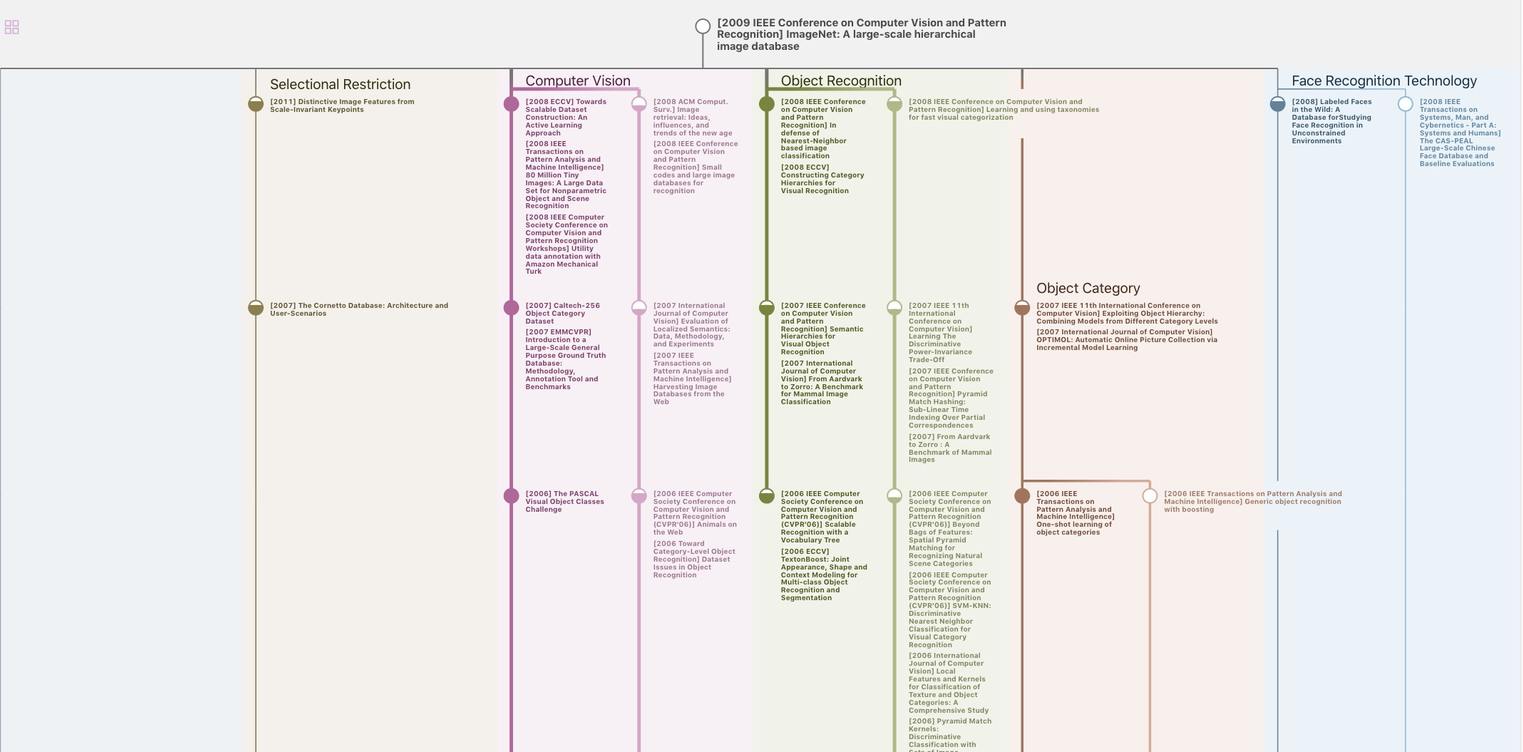
生成溯源树,研究论文发展脉络
Chat Paper
正在生成论文摘要