Verbal working memory and functional large-scale networks in schizophrenia.
Psychiatry Research: Neuroimaging(2017)
摘要
The aim of this study was to test whether bilinear and nonlinear effective connectivity (EC) measures of working memory fMRI data can differentiate between patients with schizophrenia (SZ) and healthy controls (HC). We applied bilinear and nonlinear Dynamic Causal Modeling (DCM) for the analysis of verbal working memory in 16 SZ and 21 HC. The connection strengths with nonlinear modulation between the dorsolateral prefrontal cortex (DLPFC) and the ventral tegmental area/substantia nigra (VTA/SN) were evaluated. We used Bayesian Model Selection at the group and family levels to compare the optimal bilinear and nonlinear models. Bayesian Model Averaging was used to assess the connection strengths with nonlinear modulation. The DCM analyses revealed that SZ and HC used different bilinear networks despite comparable behavioral performance. In addition, the connection strengths with nonlinear modulation between the DLPFC and the VTA/SN area showed differences between SZ and HC. The adoption of different functional networks in SZ and HC indicated neurobiological alterations underlying working memory performance, including different connection strengths with nonlinear modulation between the DLPFC and the VTA/SN area. These novel findings may increase our understanding of connectivity in working memory in schizophrenia.
更多查看译文
关键词
Working memory,Schizophrenia,Functional Magnetic Resonance Imaging,Functional large-scale networks,Nonlinear Dynamic Causal Modeling
AI 理解论文
溯源树
样例
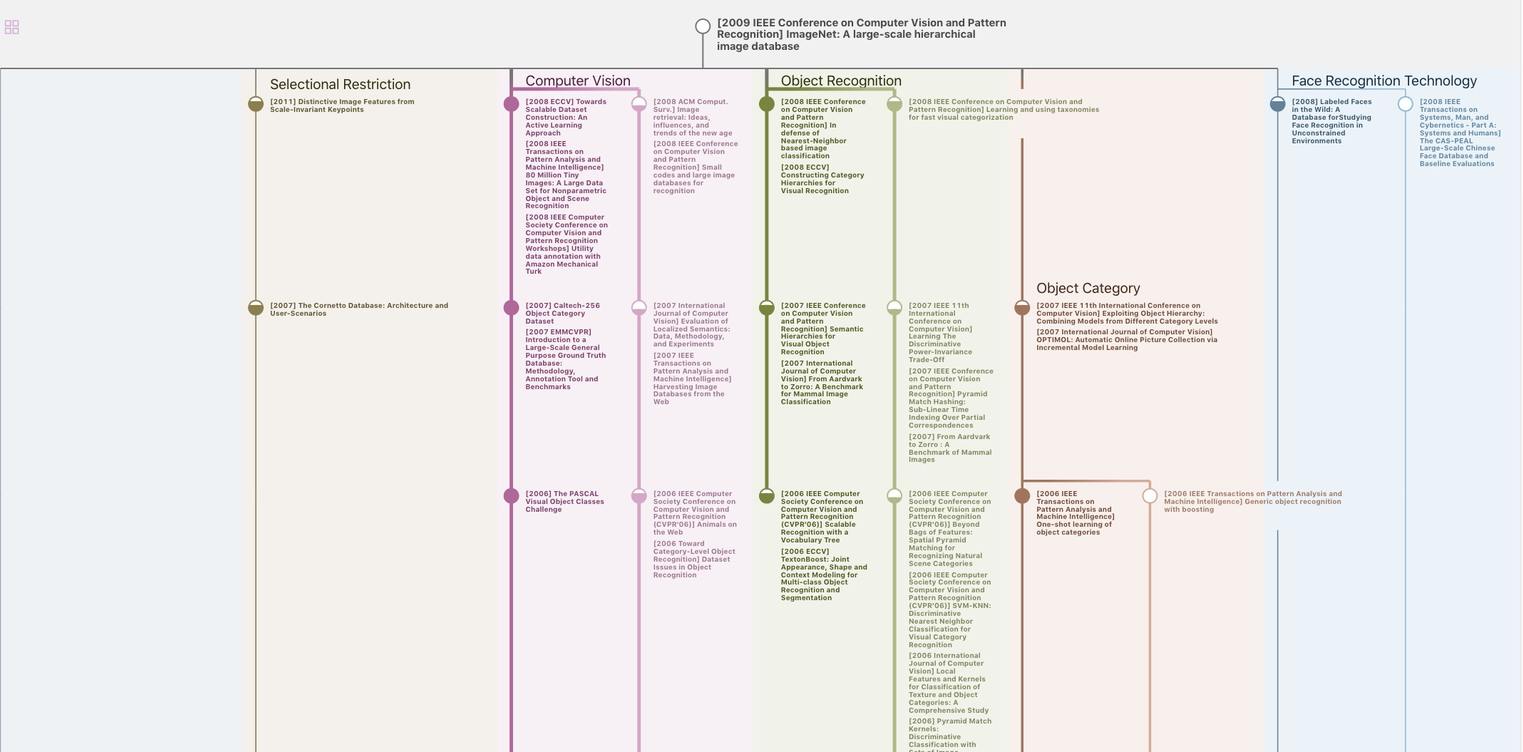
生成溯源树,研究论文发展脉络
Chat Paper
正在生成论文摘要