Multiscale Positive-Unlabeled Detection of AI-Generated Texts
arXiv (Cornell University)(2023)
摘要
Recent releases of Large Language Models (LLMs), e.g. ChatGPT, are astonishing at generating human-like texts, but they may get misused for fake scholarly texts, fake news, fake tweets, et cetera. Previous works have proposed methods to detect these multiscale AI-generated texts, including simple ML classifiers, pretrained-model-based training-agnostic methods, and finetuned language classification models. However, mainstream detectors are formulated without considering the factor of corpus length: shorter corpuses are harder to detect compared with longer ones for shortage of informative features. In this paper, a Multiscale Positive-Unlabeled (MPU) training framework is proposed to address the challenge of multiscale text detection. Firstly, we acknowledge the human-resemblance property of short machine texts, and rephrase text classification as a Positive-Unlabeled (PU) problem by marking these short machine texts as "unlabeled" during training. In this PU context, we propose the length-sensitive Multiscale PU Loss, where we use a recurrent model in abstraction to estimate positive priors of scale-variant corpuses. Additionally, we introduce a Text Multiscaling module to enrich training corpuses. Experiments show that our MPU method augments detection performance on long AI-generated text, and significantly improves short-corpus detection of language model detectors. Language Models trained with MPU could outcompete existing detectors by large margins on multiscale AI-generated texts. The codes are available at https://github.com/mindspore-lab/mindone/tree/master/examples/detect_chatgpt and https://github.com/huawei-noah/Efficient-Computing/AIGC_text_detector.
更多查看译文
关键词
detection,positive-unlabeled,ai-generated
AI 理解论文
溯源树
样例
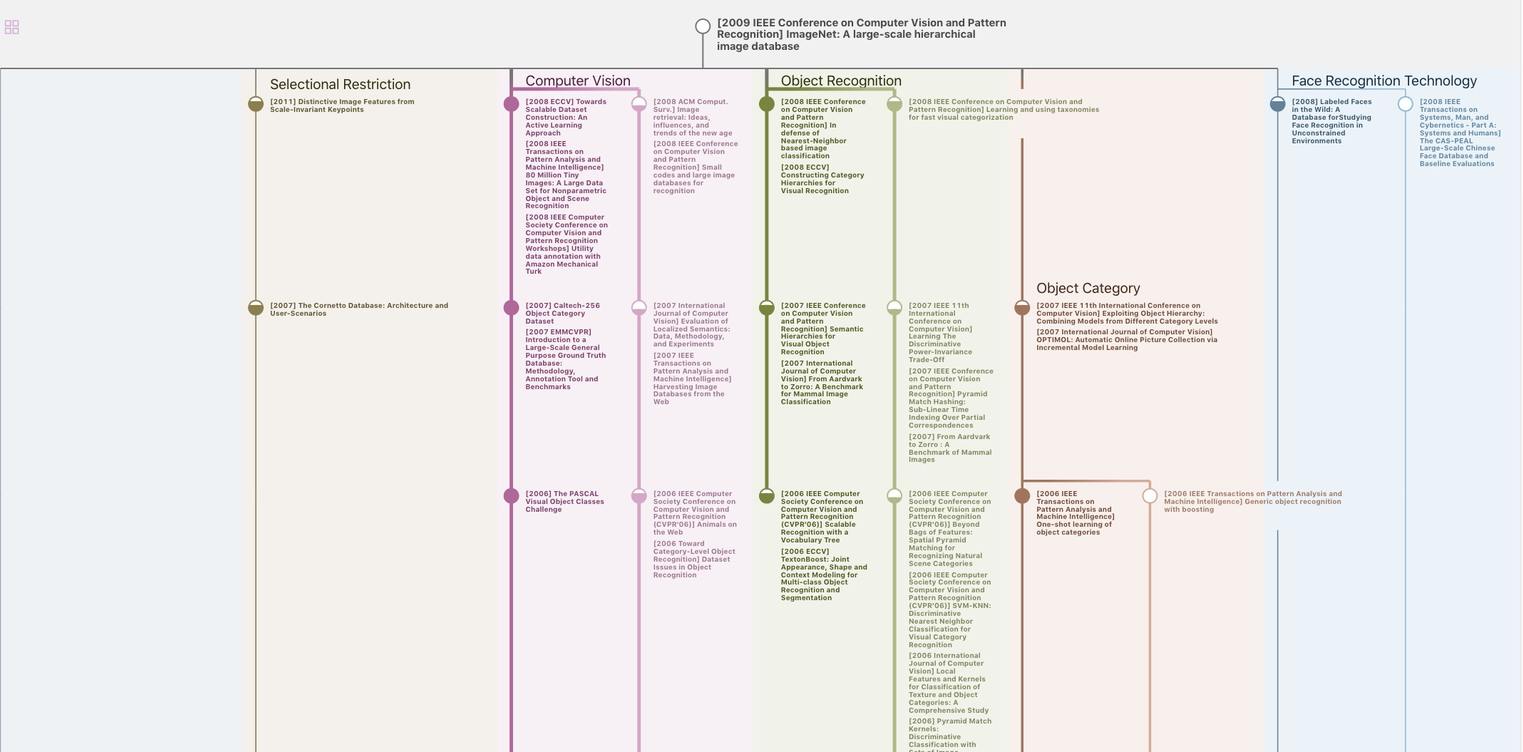
生成溯源树,研究论文发展脉络
Chat Paper
正在生成论文摘要