Influence analysis for skew-normal semiparametric joint models of multivariate longitudinal and multivariate survival data.
STATISTICS IN MEDICINE(2017)
摘要
The normality assumption of measurement error is a widely used distribution in joint models of longitudinal and survival data, but it may lead to unreasonable or even misleading results when longitudinal data reveal skewness feature. This paper proposes a new joint model for multivariate longitudinal and multivariate survival data by incorporating a nonparametric function into the trajectory function and hazard function and assuming that measurement errors in longitudinal measurement models follow a skew-normal distribution. A Monte Carlo Expectation-Maximization (EM) algorithm together with the penalized-splines technique and the MetropolisHastings algorithm within the Gibbs sampler is developed to estimate parameters and nonparametric functions in the considered joint models. Case deletion diagnostic measures are proposed to identify the potential influential observations, and an extended local influence method is presented to assess local influence ofminor perturbations. Simulation studies and a real example from a clinical trial are presented to illustrate the proposed methodologies. Copyright (C) 2017 JohnWiley & Sons, Ltd.
更多查看译文
关键词
case deletion measure,joint model,local influence analysis,Monte Carlo EM algorithm,penalized spline,skew-normal distribution
AI 理解论文
溯源树
样例
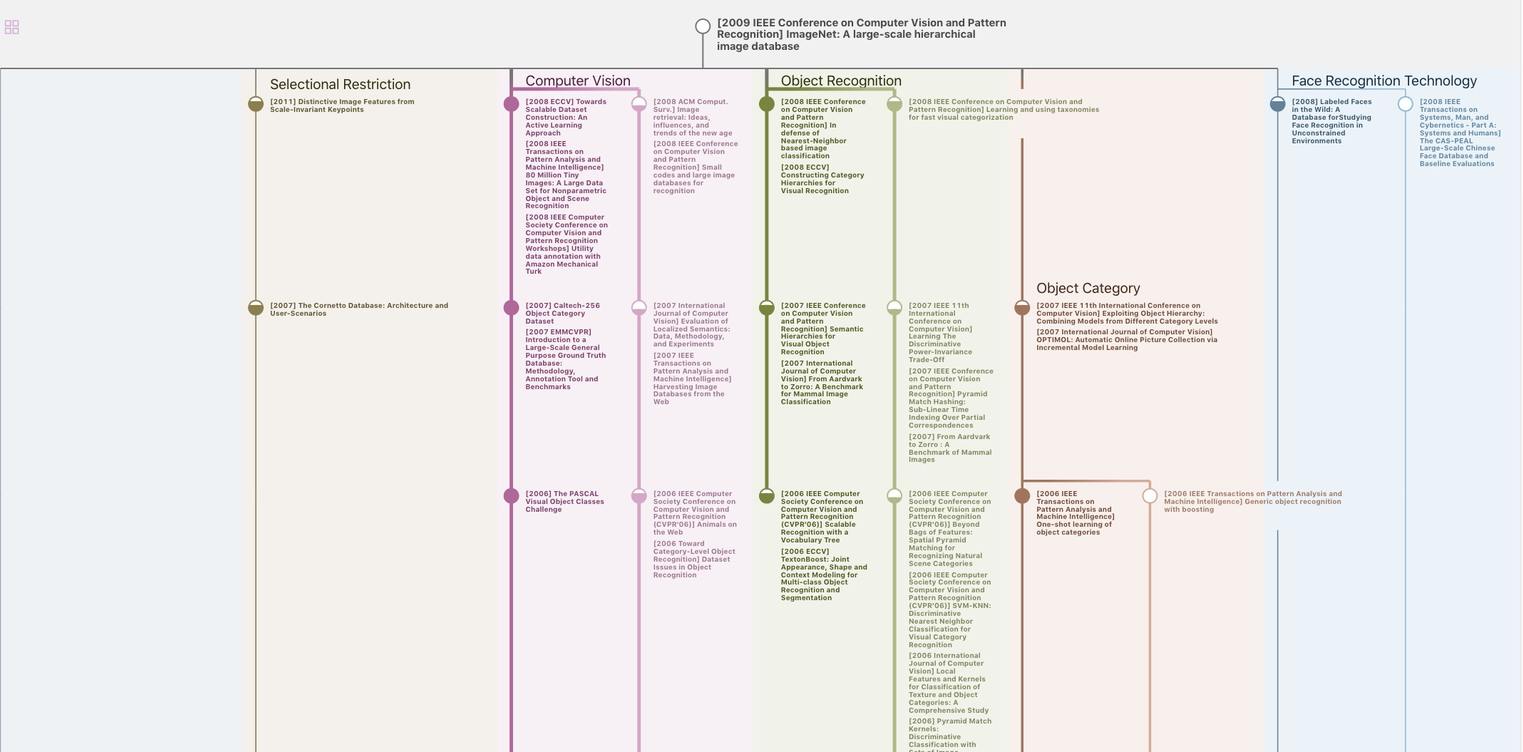
生成溯源树,研究论文发展脉络
Chat Paper
正在生成论文摘要