Identifying optimal dosage regimes under safety constraints: An application to long term opioid treatment of chronic pain.
STATISTICS IN MEDICINE(2018)
摘要
There is growing interest and investment in precision medicine as a means to provide the best possible health care. A treatment regime formalizes precision medicine as a sequence of decision rules, one per clinical intervention period, that specify if, when and how current treatment should be adjusted in response to a patient's evolving health status. It is standard to define a regime as optimal if, when applied to a population of interest, it maximizes the mean of some desirable clinical outcome, such as efficacy. However, in many clinical settings, a high-quality treatment regime must balance multiple competing outcomes; eg, when a high dose is associated with substantial symptom reduction but a greater risk of an adverse event. We consider the problem of estimating the most efficacious treatment regime subject to constraints on the risk of adverse events. We combine nonparametric Q-learning with policy-search to estimate a high-quality yet parsimonious treatment regime. This estimator applies to both observational and randomized data, as well as settings with variable, outcome-dependent follow-up, mixed treatment types, and multiple time points. This work is motivated by and framed in the context of dosing for chronic pain; however, the proposed framework can be applied generally to estimate a treatment regime which maximizes the mean of one primary outcome subject to constraints on one or more secondary outcomes. We illustrate the proposed method using data pooled from 5 open-label flexible dosing clinical trials for chronic pain.
更多查看译文
关键词
chronic pain,flexible dosing trials,policy-search,precision medicine,Q-learning,treatment dosing regimes
AI 理解论文
溯源树
样例
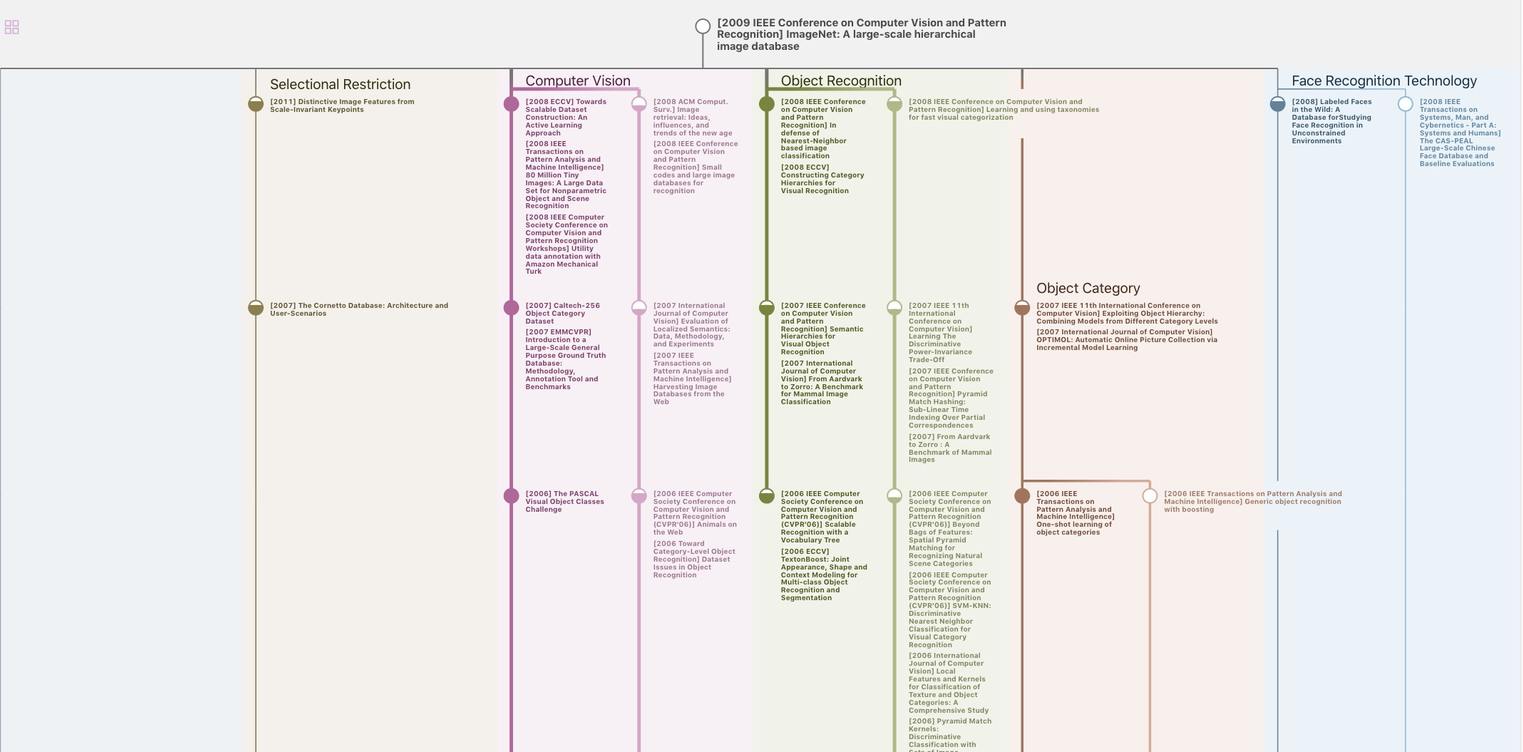
生成溯源树,研究论文发展脉络
Chat Paper
正在生成论文摘要