Gene selection for microarray cancer classification using a new evolutionary method employing artificial intelligence concepts.
Genomics(2017)
摘要
Gene selection is a demanding task for microarray data analysis. The diverse complexity of different cancers makes this issue still challenging. In this study, a novel evolutionary method based on genetic algorithms and artificial intelligence is proposed to identify predictive genes for cancer classification. A filter method was first applied to reduce the dimensionality of feature space followed by employing an integer-coded genetic algorithm with dynamic-length genotype, intelligent parameter settings, and modified operators. The algorithmic behaviors including convergence trends, mutation and crossover rate changes, and running time were studied, conceptually discussed, and shown to be coherent with literature findings. Two well-known filter methods, Laplacian and Fisher score, were examined considering similarities, the quality of selected genes, and their influences on the evolutionary approach. Several statistical tests concerning choice of classifier, choice of dataset, and choice of filter method were performed, and they revealed some significant differences between the performance of different classifiers and filter methods over datasets. The proposed method was benchmarked upon five popular high-dimensional cancer datasets; for each, top explored genes were reported. Comparing the experimental results with several state-of-the-art methods revealed that the proposed method outperforms previous methods in DLBCL dataset.
更多查看译文
关键词
Gene selection,Cancer classification,Microarray data analysis,Intelligent Dynamic Algorithm,Random-restart hill climbing,Reinforcement learning,Penalizing strategy,Cut and splice crossover,Self-refinement strategy,Feature selection
AI 理解论文
溯源树
样例
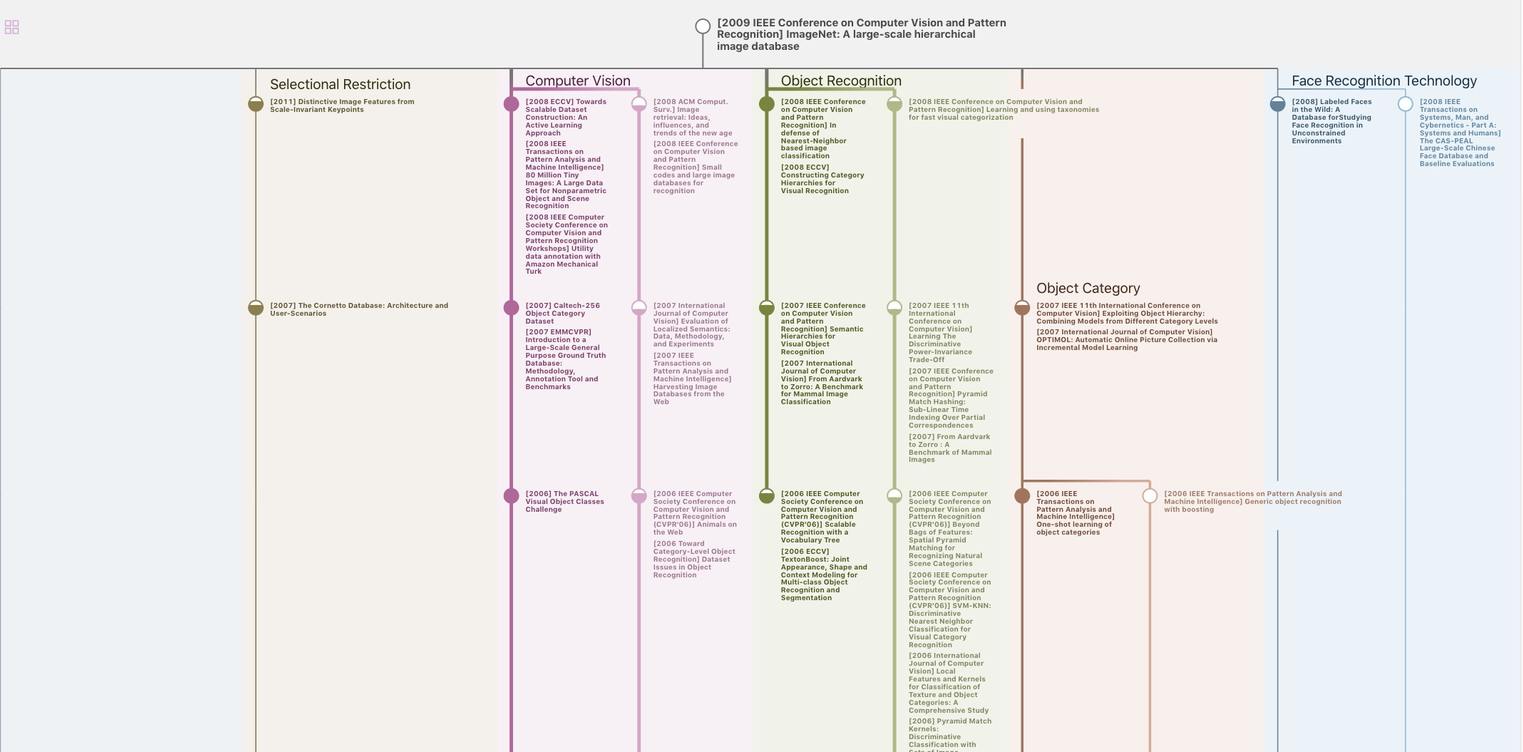
生成溯源树,研究论文发展脉络
Chat Paper
正在生成论文摘要